494 min to read
Linear Regression Example
Machine Learning in R
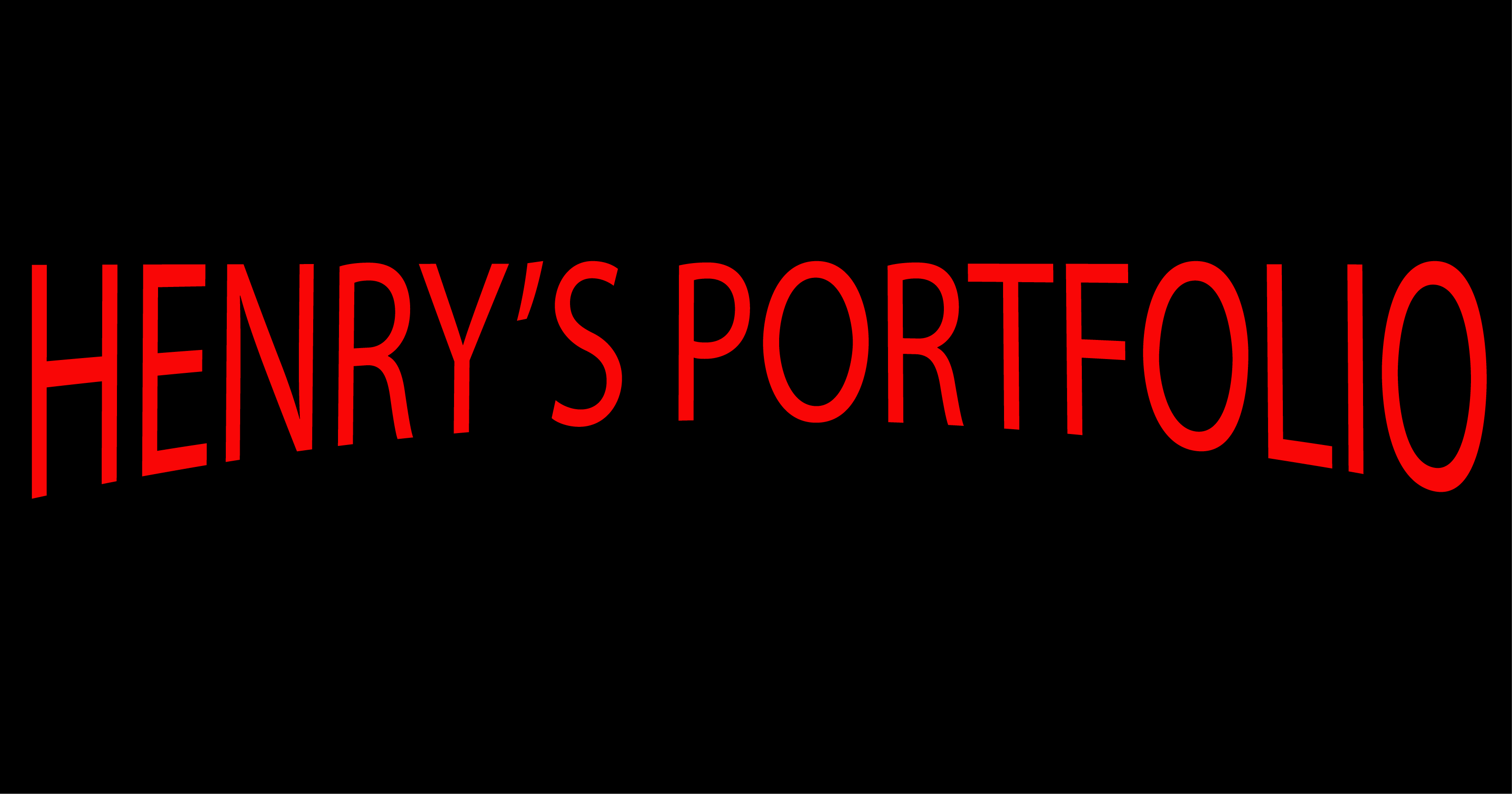
<!DOCTYPE html>
TAYKO SOFTWARE
Business Problem
Predicting Software Reselling Profits
Tayko is a software catalog firm. It sells games and educational software. Initially it began as a software manufacturer and later it added third-party titles to its offerings. It has recently put together a revised collection of items in a new catalog. Tayko is preparing to roll out the catalog in a mailing.
Tayko has joined a consortium of catalog firms that specialize in computer and software products. Through this it gets to send its cataloges to a names drawn from a pooled list of customers. It supplies a list of customers to the consortium and in return can get a list of new customers. Members can do predictive modeling on the available records in the pool to get a good set of names generating profits to the company.
In this regard, Tayko has supplied 200000 names to the pool of 5000000 names. It is entitled to draw 200000 names. To draw the best names, it tests with 2000 names for its new catalog. The work presented here uses Logistics Regression Model to help Tayko decide if the drawn list is good for its revenue generation.
OBJECTIVE: From the dataset Tayko.csv, Purchase output variable is considered for the analysis and prediction. The objective of the model is to classify records into ‘PURCHASE’ or "NO PURCHASE’.
The following list explains the details for the independent variables in the dataset:
Variable Name Description
US Is the address in US?
Freq Number of transactions in the last year
last_update_days_ago How many days ago last update was made
1st_update_days_ago How many days ago first update was made
Web_Order Customer placed at least one order via web
Gender Customer is male or not
Address_is_res Address is a residence
Purchase Person made purchase in testing mailing or not
Read in data
library(tibble)
library(kableExtra)
rm(list = ls())
Tayko.df <- read.csv("https://raw.githubusercontent.com/HenryBernreuter/test_data/main/CSV_Tayko.csv")
Tayko.df %>%
kbl() %>%
scroll_box(width = "750px", height = "500px")
sequence_number | Address_US | Freq | last_update | Web | Gender | Address_RES | Purchase | Spending |
---|---|---|---|---|---|---|---|---|
1 | 1 | 2 | 3662 | 1 | 0 | 1 | 1 | 128 |
2 | 1 | 0 | 2900 | 1 | 1 | 0 | 0 | 0 |
3 | 1 | 2 | 3883 | 0 | 0 | 0 | 1 | 127 |
4 | 1 | 1 | 829 | 0 | 1 | 0 | 0 | 0 |
5 | 1 | 1 | 869 | 0 | 0 | 0 | 0 | 0 |
6 | 1 | 1 | 1995 | 0 | 0 | 1 | 0 | 0 |
7 | 1 | 2 | 1498 | 0 | 0 | 1 | 0 | 0 |
8 | 1 | 1 | 3397 | 0 | 1 | 0 | 0 | 0 |
9 | 1 | 4 | 525 | 1 | 1 | 0 | 1 | 489 |
10 | 1 | 1 | 3215 | 0 | 0 | 0 | 1 | 174 |
11 | 1 | 0 | 734 | 0 | 1 | 0 | 0 | 0 |
12 | 1 | 2 | 1275 | 0 | 0 | 1 | 0 | 0 |
13 | 1 | 1 | 2802 | 1 | 1 | 0 | 0 | 0 |
14 | 1 | 5 | 2081 | 0 | 1 | 0 | 1 | 1416 |
15 | 0 | 1 | 1465 | 0 | 0 | 1 | 1 | 192 |
16 | 0 | 1 | 2523 | 1 | 0 | 0 | 0 | 0 |
17 | 1 | 2 | 1801 | 1 | 0 | 1 | 0 | 0 |
18 | 0 | 0 | 3694 | 1 | 0 | 0 | 0 | 0 |
19 | 1 | 2 | 1879 | 1 | 1 | 1 | 1 | 130 |
20 | 1 | 0 | 3120 | 0 | 1 | 0 | 0 | 0 |
21 | 1 | 2 | 2943 | 1 | 0 | 0 | 1 | 386 |
22 | 1 | 2 | 1928 | 0 | 1 | 0 | 1 | 161 |
23 | 1 | 1 | 310 | 0 | 0 | 1 | 0 | 0 |
24 | 1 | 2 | 1463 | 0 | 0 | 1 | 1 | 174 |
25 | 1 | 3 | 3613 | 1 | 1 | 0 | 1 | 131 |
26 | 1 | 1 | 2460 | 1 | 0 | 0 | 1 | 189 |
27 | 0 | 0 | 3394 | 0 | 0 | 0 | 0 | 0 |
28 | 1 | 0 | 1569 | 1 | 0 | 0 | 0 | 0 |
29 | 1 | 1 | 3299 | 0 | 0 | 0 | 1 | 90 |
30 | 1 | 2 | 3744 | 0 | 1 | 0 | 1 | 354 |
31 | 1 | 0 | 3090 | 0 | 0 | 0 | 0 | 0 |
32 | 0 | 2 | 2947 | 1 | 0 | 0 | 1 | 352 |
33 | 0 | 1 | 2123 | 0 | 1 | 0 | 0 | 0 |
34 | 1 | 0 | 2905 | 1 | 1 | 0 | 0 | 0 |
35 | 0 | 0 | 2005 | 0 | 1 | 1 | 0 | 0 |
36 | 1 | 1 | 1158 | 0 | 1 | 1 | 0 | 0 |
37 | 1 | 2 | 2478 | 0 | 1 | 0 | 1 | 185 |
38 | 0 | 1 | 1058 | 0 | 0 | 0 | 0 | 0 |
39 | 1 | 1 | 1774 | 0 | 0 | 0 | 0 | 0 |
40 | 1 | 0 | 3694 | 1 | 0 | 0 | 0 | 0 |
41 | 1 | 2 | 1752 | 1 | 0 | 1 | 0 | 0 |
42 | 1 | 2 | 3698 | 0 | 1 | 0 | 1 | 34 |
43 | 1 | 2 | 1318 | 0 | 1 | 1 | 1 | 161 |
44 | 1 | 9 | 868 | 1 | 0 | 1 | 1 | 639 |
45 | 1 | 2 | 2752 | 0 | 1 | 0 | 1 | 638 |
46 | 1 | 1 | 3338 | 0 | 1 | 1 | 1 | 159 |
47 | 1 | 0 | 3747 | 0 | 0 | 0 | 0 | 0 |
48 | 1 | 0 | 2905 | 1 | 0 | 0 | 0 | 0 |
49 | 1 | 2 | 1786 | 0 | 0 | 0 | 1 | 41 |
50 | 0 | 1 | 316 | 1 | 1 | 0 | 0 | 0 |
51 | 1 | 2 | 2226 | 0 | 1 | 0 | 1 | 232 |
52 | 1 | 2 | 1920 | 0 | 0 | 1 | 0 | 0 |
53 | 1 | 8 | 106 | 1 | 0 | 0 | 1 | 1030 |
54 | 1 | 0 | 1822 | 1 | 1 | 0 | 0 | 0 |
55 | 1 | 1 | 1170 | 1 | 1 | 0 | 1 | 375 |
56 | 1 | 0 | 3157 | 0 | 1 | 1 | 0 | 0 |
57 | 1 | 5 | 1856 | 0 | 0 | 1 | 1 | 362 |
58 | 0 | 1 | 2212 | 0 | 0 | 0 | 1 | 95 |
59 | 1 | 2 | 3338 | 0 | 1 | 0 | 1 | 109 |
60 | 1 | 0 | 3367 | 0 | 1 | 0 | 0 | 0 |
61 | 1 | 3 | 2957 | 1 | 1 | 0 | 1 | 136 |
62 | 1 | 1 | 834 | 0 | 1 | 0 | 0 | 0 |
63 | 1 | 3 | 2908 | 0 | 1 | 0 | 1 | 261 |
64 | 1 | 1 | 906 | 1 | 1 | 0 | 1 | 43 |
65 | 1 | 6 | 1408 | 0 | 1 | 1 | 1 | 389 |
66 | 1 | 3 | 1835 | 1 | 0 | 0 | 1 | 80 |
67 | 1 | 1 | 2803 | 0 | 0 | 0 | 0 | 0 |
68 | 1 | 4 | 479 | 1 | 1 | 0 | 1 | 394 |
69 | 1 | 6 | 1470 | 0 | 1 | 0 | 1 | 588 |
70 | 1 | 1 | 3654 | 1 | 1 | 0 | 0 | 0 |
71 | 1 | 1 | 3716 | 0 | 0 | 1 | 1 | 298 |
72 | 1 | 0 | 3125 | 0 | 0 | 0 | 0 | 0 |
73 | 1 | 4 | 1995 | 0 | 1 | 1 | 0 | 0 |
74 | 1 | 0 | 3747 | 0 | 1 | 0 | 0 | 0 |
75 | 1 | 1 | 2438 | 0 | 1 | 1 | 0 | 0 |
76 | 1 | 1 | 2848 | 1 | 1 | 0 | 0 | 0 |
77 | 0 | 1 | 3125 | 1 | 1 | 0 | 1 | 160 |
78 | 1 | 2 | 541 | 1 | 1 | 1 | 1 | 50 |
79 | 1 | 1 | 2859 | 1 | 0 | 0 | 1 | 161 |
80 | 1 | 1 | 1095 | 1 | 0 | 1 | 0 | 0 |
81 | 1 | 1 | 2508 | 0 | 1 | 0 | 1 | 233 |
82 | 1 | 1 | 2862 | 0 | 0 | 0 | 1 | 129 |
83 | 1 | 0 | 3745 | 1 | 0 | 0 | 0 | 0 |
84 | 1 | 2 | 3698 | 1 | 0 | 0 | 1 | 98 |
85 | 1 | 0 | 3746 | 0 | 1 | 0 | 0 | 0 |
86 | 1 | 2 | 2962 | 1 | 0 | 0 | 1 | 132 |
87 | 1 | 2 | 841 | 1 | 0 | 1 | 1 | 158 |
88 | 1 | 1 | 2912 | 1 | 1 | 0 | 0 | 0 |
89 | 0 | 2 | 458 | 1 | 0 | 0 | 1 | 248 |
90 | 1 | 1 | 1269 | 1 | 0 | 0 | 1 | 277 |
91 | 1 | 1 | 2982 | 1 | 1 | 0 | 0 | 0 |
92 | 1 | 1 | 1415 | 1 | 0 | 1 | 0 | 0 |
93 | 1 | 0 | 4005 | 0 | 0 | 0 | 0 | 0 |
94 | 1 | 1 | 2102 | 1 | 1 | 0 | 1 | 54 |
95 | 1 | 1 | 2872 | 0 | 1 | 0 | 0 | 0 |
96 | 1 | 1 | 2548 | 1 | 0 | 0 | 1 | 230 |
97 | 1 | 0 | 2740 | 0 | 0 | 0 | 0 | 0 |
98 | 1 | 0 | 217 | 0 | 0 | 0 | 0 | 0 |
99 | 0 | 1 | 366 | 0 | 1 | 0 | 0 | 0 |
100 | 1 | 3 | 822 | 1 | 0 | 1 | 1 | 287 |
101 | 1 | 0 | 3746 | 0 | 0 | 0 | 0 | 0 |
102 | 1 | 1 | 3772 | 0 | 1 | 0 | 0 | 0 |
103 | 1 | 0 | 2781 | 0 | 1 | 0 | 0 | 0 |
104 | 1 | 1 | 511 | 0 | 0 | 0 | 0 | 0 |
105 | 1 | 1 | 1112 | 0 | 1 | 0 | 1 | 164 |
106 | 0 | 1 | 2807 | 1 | 0 | 0 | 1 | 405 |
107 | 1 | 0 | 1011 | 0 | 1 | 0 | 0 | 0 |
108 | 1 | 1 | 2907 | 0 | 1 | 0 | 1 | 160 |
109 | 1 | 1 | 3724 | 0 | 1 | 0 | 1 | 305 |
110 | 1 | 1 | 3356 | 0 | 0 | 0 | 0 | 0 |
111 | 0 | 3 | 3791 | 1 | 1 | 1 | 1 | 534 |
112 | 1 | 2 | 3642 | 1 | 0 | 0 | 0 | 0 |
113 | 0 | 1 | 4005 | 1 | 1 | 0 | 1 | 105 |
114 | 1 | 1 | 1130 | 1 | 1 | 0 | 1 | 79 |
115 | 1 | 6 | 1128 | 1 | 0 | 1 | 1 | 404 |
116 | 1 | 2 | 1521 | 0 | 1 | 1 | 0 | 0 |
117 | 1 | 2 | 1882 | 0 | 1 | 1 | 0 | 0 |
118 | 0 | 2 | 1529 | 0 | 1 | 0 | 1 | 186 |
119 | 1 | 1 | 2404 | 0 | 0 | 0 | 1 | 114 |
120 | 1 | 1 | 667 | 0 | 1 | 0 | 1 | 324 |
121 | 1 | 1 | 4157 | 1 | 1 | 0 | 1 | 69 |
122 | 1 | 2 | 3436 | 1 | 1 | 0 | 1 | 225 |
123 | 1 | 1 | 1154 | 0 | 0 | 1 | 1 | 205 |
124 | 1 | 1 | 1263 | 1 | 1 | 0 | 0 | 0 |
125 | 1 | 1 | 1863 | 0 | 1 | 1 | 1 | 167 |
126 | 1 | 3 | 731 | 0 | 0 | 1 | 0 | 0 |
127 | 1 | 0 | 1816 | 1 | 0 | 0 | 0 | 0 |
128 | 1 | 1 | 1302 | 1 | 1 | 0 | 1 | 67 |
129 | 1 | 2 | 785 | 1 | 0 | 0 | 1 | 206 |
130 | 1 | 1 | 3883 | 0 | 1 | 0 | 1 | 67 |
131 | 1 | 1 | 2960 | 0 | 1 | 1 | 1 | 120 |
132 | 1 | 5 | 617 | 0 | 0 | 0 | 1 | 356 |
133 | 1 | 1 | 3009 | 0 | 0 | 1 | 1 | 129 |
134 | 1 | 1 | 2404 | 0 | 1 | 0 | 0 | 0 |
135 | 1 | 1 | 1968 | 0 | 1 | 0 | 0 | 0 |
136 | 1 | 4 | 1121 | 1 | 1 | 0 | 1 | 420 |
137 | 1 | 0 | 2879 | 1 | 0 | 0 | 0 | 0 |
138 | 1 | 1 | 2648 | 1 | 1 | 0 | 1 | 130 |
139 | 1 | 0 | 2891 | 1 | 1 | 0 | 0 | 0 |
140 | 1 | 3 | 1240 | 1 | 1 | 1 | 1 | 141 |
141 | 1 | 1 | 2856 | 0 | 1 | 0 | 0 | 0 |
142 | 1 | 0 | 2977 | 0 | 0 | 0 | 0 | 0 |
143 | 1 | 1 | 2960 | 1 | 1 | 0 | 1 | 129 |
144 | 1 | 1 | 3047 | 0 | 1 | 1 | 0 | 0 |
145 | 1 | 0 | 2765 | 1 | 0 | 0 | 0 | 0 |
146 | 1 | 1 | 1109 | 0 | 0 | 0 | 1 | 21 |
147 | 1 | 5 | 2268 | 0 | 0 | 0 | 1 | 605 |
148 | 1 | 1 | 1147 | 1 | 0 | 0 | 1 | 164 |
149 | 1 | 2 | 2425 | 0 | 0 | 0 | 1 | 130 |
150 | 1 | 1 | 2296 | 0 | 0 | 0 | 1 | 161 |
151 | 1 | 0 | 3619 | 0 | 0 | 0 | 0 | 0 |
152 | 1 | 2 | 1016 | 1 | 0 | 0 | 1 | 451 |
153 | 1 | 1 | 723 | 0 | 1 | 1 | 0 | 0 |
154 | 1 | 2 | 841 | 0 | 1 | 1 | 0 | 0 |
155 | 1 | 1 | 890 | 0 | 1 | 0 | 0 | 0 |
156 | 1 | 3 | 1242 | 1 | 1 | 1 | 1 | 432 |
157 | 1 | 0 | 3747 | 1 | 1 | 0 | 0 | 0 |
158 | 1 | 1 | 2060 | 0 | 1 | 0 | 0 | 0 |
159 | 1 | 2 | 3074 | 1 | 0 | 0 | 1 | 163 |
160 | 1 | 3 | 2024 | 0 | 1 | 0 | 1 | 547 |
161 | 1 | 0 | 3138 | 1 | 0 | 1 | 0 | 0 |
162 | 1 | 1 | 3334 | 1 | 0 | 0 | 0 | 0 |
163 | 0 | 0 | 90 | 0 | 1 | 0 | 0 | 0 |
164 | 1 | 1 | 2632 | 1 | 0 | 0 | 1 | 140 |
165 | 1 | 1 | 3451 | 1 | 0 | 0 | 0 | 0 |
166 | 0 | 2 | 3543 | 0 | 0 | 0 | 1 | 129 |
167 | 1 | 1 | 169 | 1 | 1 | 0 | 1 | 163 |
168 | 1 | 3 | 3662 | 0 | 1 | 1 | 1 | 310 |
169 | 1 | 2 | 582 | 1 | 1 | 0 | 1 | 326 |
170 | 1 | 2 | 969 | 0 | 0 | 1 | 1 | 43 |
171 | 1 | 1 | 1968 | 1 | 1 | 0 | 0 | 0 |
172 | 0 | 1 | 364 | 1 | 0 | 0 | 1 | 249 |
173 | 0 | 0 | 2185 | 0 | 1 | 0 | 0 | 0 |
174 | 1 | 0 | 91 | 0 | 0 | 0 | 0 | 0 |
175 | 1 | 0 | 3502 | 0 | 1 | 0 | 0 | 0 |
176 | 1 | 1 | 3485 | 0 | 1 | 0 | 0 | 0 |
177 | 1 | 1 | 2855 | 0 | 1 | 0 | 0 | 0 |
178 | 1 | 1 | 1890 | 1 | 0 | 0 | 1 | 77 |
179 | 1 | 1 | 3944 | 1 | 1 | 0 | 1 | 68 |
180 | 1 | 3 | 2347 | 0 | 0 | 0 | 1 | 219 |
181 | 1 | 1 | 3237 | 0 | 1 | 1 | 0 | 0 |
182 | 1 | 2 | 3772 | 1 | 0 | 0 | 1 | 149 |
183 | 0 | 2 | 3583 | 1 | 0 | 0 | 1 | 140 |
184 | 0 | 1 | 140 | 0 | 1 | 1 | 0 | 0 |
185 | 1 | 1 | 2960 | 0 | 1 | 1 | 1 | 129 |
186 | 1 | 1 | 1598 | 1 | 1 | 0 | 1 | 40 |
187 | 1 | 3 | 1689 | 0 | 0 | 1 | 1 | 108 |
188 | 1 | 1 | 2675 | 0 | 1 | 0 | 1 | 30 |
189 | 1 | 4 | 1989 | 0 | 1 | 0 | 1 | 130 |
190 | 1 | 1 | 1569 | 1 | 1 | 0 | 1 | 32 |
191 | 1 | 1 | 1906 | 1 | 0 | 0 | 1 | 50 |
192 | 1 | 1 | 2408 | 1 | 0 | 0 | 1 | 54 |
193 | 1 | 2 | 1864 | 0 | 1 | 0 | 1 | 243 |
194 | 1 | 1 | 2947 | 0 | 0 | 0 | 1 | 80 |
195 | 0 | 2 | 2723 | 1 | 1 | 0 | 1 | 237 |
196 | 1 | 1 | 3660 | 1 | 1 | 0 | 0 | 0 |
197 | 1 | 1 | 2893 | 0 | 1 | 0 | 0 | 0 |
198 | 1 | 3 | 2466 | 1 | 1 | 0 | 1 | 145 |
199 | 1 | 2 | 1882 | 0 | 0 | 1 | 0 | 0 |
200 | 0 | 1 | 2502 | 0 | 0 | 1 | 0 | 0 |
201 | 1 | 3 | 2024 | 1 | 1 | 0 | 1 | 437 |
202 | 1 | 2 | 2080 | 1 | 1 | 1 | 1 | 35 |
203 | 1 | 2 | 1856 | 0 | 0 | 1 | 0 | 0 |
204 | 1 | 1 | 583 | 0 | 0 | 0 | 1 | 412 |
205 | 0 | 0 | 3369 | 0 | 0 | 0 | 0 | 0 |
206 | 1 | 0 | 181 | 0 | 0 | 0 | 0 | 0 |
207 | 1 | 1 | 50 | 1 | 1 | 0 | 1 | 25 |
208 | 1 | 1 | 3270 | 1 | 0 | 0 | 1 | 153 |
209 | 0 | 1 | 1926 | 0 | 1 | 0 | 1 | 62 |
210 | 1 | 1 | 1567 | 1 | 0 | 0 | 1 | 32 |
211 | 1 | 2 | 2404 | 1 | 0 | 0 | 1 | 187 |
212 | 1 | 1 | 906 | 0 | 0 | 0 | 1 | 80 |
213 | 1 | 2 | 1577 | 1 | 0 | 0 | 1 | 277 |
214 | 1 | 2 | 1037 | 0 | 1 | 0 | 1 | 278 |
215 | 0 | 1 | 2768 | 0 | 0 | 0 | 0 | 0 |
216 | 1 | 1 | 3522 | 0 | 0 | 0 | 0 | 0 |
217 | 0 | 4 | 511 | 1 | 0 | 0 | 1 | 268 |
218 | 1 | 1 | 833 | 0 | 0 | 0 | 0 | 0 |
219 | 1 | 3 | 191 | 1 | 1 | 0 | 1 | 363 |
220 | 1 | 1 | 182 | 1 | 1 | 0 | 1 | 1075 |
221 | 1 | 2 | 2201 | 0 | 0 | 0 | 1 | 164 |
222 | 1 | 3 | 1962 | 1 | 1 | 1 | 0 | 0 |
223 | 1 | 1 | 3236 | 1 | 1 | 0 | 1 | 128 |
224 | 0 | 2 | 583 | 1 | 0 | 0 | 1 | 69 |
225 | 1 | 1 | 3453 | 1 | 0 | 0 | 0 | 0 |
226 | 1 | 0 | 3746 | 0 | 1 | 0 | 0 | 0 |
227 | 1 | 1 | 2081 | 1 | 0 | 1 | 1 | 130 |
228 | 0 | 1 | 2541 | 0 | 0 | 0 | 1 | 174 |
229 | 1 | 3 | 2205 | 0 | 0 | 0 | 1 | 36 |
230 | 1 | 1 | 3263 | 0 | 0 | 0 | 0 | 0 |
231 | 1 | 1 | 2918 | 1 | 1 | 0 | 0 | 0 |
232 | 1 | 1 | 2996 | 1 | 0 | 0 | 1 | 184 |
233 | 0 | 1 | 1701 | 0 | 0 | 0 | 1 | 110 |
234 | 1 | 1 | 3883 | 1 | 1 | 0 | 1 | 33 |
235 | 1 | 2 | 2802 | 1 | 0 | 0 | 1 | 130 |
236 | 1 | 1 | 1946 | 1 | 0 | 0 | 1 | 130 |
237 | 1 | 1 | 2562 | 1 | 1 | 0 | 1 | 35 |
238 | 1 | 1 | 2705 | 0 | 1 | 0 | 1 | 130 |
239 | 1 | 1 | 2333 | 1 | 1 | 1 | 1 | 161 |
240 | 1 | 2 | 2933 | 1 | 1 | 0 | 1 | 54 |
241 | 1 | 1 | 290 | 1 | 1 | 0 | 1 | 163 |
242 | 1 | 0 | 3745 | 0 | 1 | 0 | 0 | 0 |
243 | 1 | 1 | 3517 | 0 | 0 | 0 | 0 | 0 |
244 | 1 | 1 | 890 | 0 | 1 | 0 | 0 | 0 |
245 | 0 | 1 | 42 | 0 | 0 | 1 | 0 | 0 |
246 | 0 | 1 | 772 | 0 | 0 | 0 | 1 | 80 |
247 | 1 | 2 | 2194 | 0 | 0 | 1 | 1 | 161 |
248 | 0 | 2 | 1141 | 0 | 1 | 0 | 1 | 208 |
249 | 1 | 1 | 3011 | 0 | 1 | 0 | 1 | 80 |
250 | 1 | 2 | 2080 | 1 | 0 | 1 | 1 | 35 |
251 | 1 | 0 | 3507 | 0 | 0 | 0 | 0 | 0 |
252 | 1 | 1 | 1968 | 1 | 1 | 0 | 0 | 0 |
253 | 1 | 1 | 962 | 0 | 0 | 0 | 1 | 141 |
254 | 1 | 1 | 2212 | 0 | 0 | 0 | 0 | 0 |
255 | 1 | 1 | 2648 | 1 | 1 | 0 | 1 | 130 |
256 | 1 | 2 | 1415 | 0 | 1 | 1 | 0 | 0 |
257 | 1 | 2 | 1758 | 1 | 1 | 0 | 1 | 167 |
258 | 1 | 1 | 2383 | 0 | 1 | 0 | 0 | 0 |
259 | 0 | 1 | 814 | 1 | 1 | 0 | 1 | 172 |
260 | 1 | 1 | 58 | 1 | 0 | 0 | 1 | 99 |
261 | 0 | 0 | 3528 | 1 | 1 | 0 | 0 | 0 |
262 | 1 | 1 | 2960 | 0 | 0 | 0 | 1 | 130 |
263 | 1 | 2 | 1882 | 1 | 0 | 0 | 1 | 210 |
264 | 1 | 0 | 2737 | 0 | 1 | 1 | 0 | 0 |
265 | 1 | 2 | 2163 | 0 | 0 | 0 | 1 | 107 |
266 | 0 | 1 | 1683 | 0 | 0 | 0 | 1 | 85 |
267 | 1 | 6 | 800 | 1 | 1 | 0 | 1 | 1294 |
268 | 1 | 2 | 304 | 1 | 0 | 0 | 1 | 303 |
269 | 1 | 4 | 1134 | 1 | 0 | 0 | 1 | 271 |
270 | 1 | 1 | 1361 | 0 | 0 | 0 | 1 | 1250 |
271 | 1 | 2 | 1204 | 0 | 0 | 0 | 1 | 81 |
272 | 1 | 1 | 4065 | 0 | 0 | 0 | 1 | 32 |
273 | 1 | 0 | 616 | 1 | 0 | 1 | 0 | 0 |
274 | 1 | 1 | 1415 | 0 | 1 | 1 | 0 | 0 |
275 | 0 | 0 | 2705 | 0 | 1 | 0 | 0 | 0 |
276 | 1 | 1 | 107 | 1 | 1 | 0 | 1 | 129 |
277 | 0 | 2 | 2009 | 0 | 1 | 0 | 1 | 163 |
278 | 1 | 1 | 833 | 0 | 1 | 0 | 0 | 0 |
279 | 0 | 3 | 1086 | 0 | 1 | 1 | 1 | 135 |
280 | 1 | 4 | 1879 | 0 | 0 | 0 | 1 | 164 |
281 | 1 | 0 | 744 | 1 | 0 | 0 | 0 | 0 |
282 | 1 | 2 | 1786 | 1 | 0 | 0 | 1 | 95 |
283 | 1 | 4 | 1129 | 0 | 0 | 0 | 1 | 280 |
284 | 1 | 2 | 1899 | 0 | 0 | 1 | 1 | 166 |
285 | 1 | 0 | 301 | 0 | 1 | 0 | 0 | 0 |
286 | 1 | 1 | 1162 | 1 | 0 | 1 | 1 | 164 |
287 | 1 | 0 | 2735 | 0 | 1 | 0 | 0 | 0 |
288 | 1 | 1 | 2478 | 0 | 0 | 0 | 1 | 136 |
289 | 1 | 1 | 7 | 0 | 1 | 0 | 1 | 129 |
290 | 0 | 2 | 1130 | 0 | 0 | 0 | 1 | 309 |
291 | 0 | 0 | 1035 | 1 | 1 | 1 | 0 | 0 |
292 | 1 | 2 | 2891 | 1 | 0 | 1 | 1 | 143 |
293 | 1 | 1 | 2599 | 1 | 0 | 0 | 1 | 130 |
294 | 0 | 3 | 1415 | 0 | 1 | 1 | 0 | 0 |
295 | 1 | 2 | 1120 | 1 | 1 | 0 | 1 | 200 |
296 | 1 | 1 | 833 | 0 | 1 | 0 | 0 | 0 |
297 | 0 | 3 | 283 | 0 | 0 | 0 | 1 | 405 |
298 | 1 | 2 | 1752 | 1 | 1 | 0 | 0 | 0 |
299 | 1 | 1 | 3025 | 1 | 0 | 0 | 1 | 153 |
300 | 1 | 2 | 1175 | 0 | 0 | 0 | 1 | 44 |
301 | 1 | 2 | 3791 | 1 | 1 | 0 | 1 | 88 |
302 | 0 | 1 | 2767 | 1 | 0 | 0 | 1 | 124 |
303 | 1 | 1 | 1690 | 0 | 0 | 0 | 1 | 90 |
304 | 1 | 1 | 1666 | 0 | 0 | 0 | 1 | 165 |
305 | 0 | 1 | 2793 | 1 | 0 | 0 | 1 | 170 |
306 | 1 | 1 | 512 | 0 | 0 | 1 | 0 | 0 |
307 | 1 | 0 | 3914 | 0 | 0 | 0 | 0 | 0 |
308 | 1 | 1 | 1290 | 0 | 0 | 0 | 1 | 112 |
309 | 0 | 1 | 1626 | 0 | 0 | 0 | 1 | 165 |
310 | 1 | 1 | 1968 | 1 | 0 | 0 | 1 | 255 |
311 | 1 | 1 | 2305 | 1 | 0 | 0 | 1 | 60 |
312 | 1 | 0 | 1505 | 0 | 1 | 0 | 0 | 0 |
313 | 1 | 4 | 526 | 1 | 0 | 1 | 1 | 427 |
314 | 1 | 0 | 3394 | 0 | 1 | 0 | 0 | 0 |
315 | 1 | 1 | 1991 | 0 | 1 | 0 | 1 | 261 |
316 | 0 | 1 | 3453 | 0 | 1 | 0 | 0 | 0 |
317 | 0 | 1 | 408 | 0 | 0 | 1 | 1 | 188 |
318 | 1 | 1 | 2102 | 1 | 1 | 0 | 1 | 161 |
319 | 1 | 4 | 2100 | 0 | 1 | 0 | 1 | 204 |
320 | 0 | 3 | 3398 | 1 | 0 | 0 | 1 | 192 |
321 | 0 | 2 | 3479 | 0 | 0 | 1 | 1 | 189 |
322 | 1 | 0 | 441 | 1 | 1 | 0 | 0 | 0 |
323 | 1 | 2 | 520 | 1 | 1 | 0 | 1 | 212 |
324 | 1 | 2 | 2194 | 0 | 1 | 1 | 1 | 30 |
325 | 1 | 2 | 217 | 1 | 1 | 0 | 0 | 0 |
326 | 1 | 0 | 3401 | 0 | 1 | 0 | 0 | 0 |
327 | 1 | 2 | 1582 | 1 | 0 | 1 | 1 | 3 |
328 | 1 | 1 | 2380 | 1 | 1 | 0 | 0 | 0 |
329 | 1 | 0 | 3522 | 0 | 0 | 0 | 0 | 0 |
330 | 1 | 1 | 868 | 1 | 0 | 0 | 0 | 0 |
331 | 1 | 1 | 2758 | 1 | 0 | 0 | 1 | 161 |
332 | 1 | 3 | 2481 | 1 | 1 | 0 | 1 | 619 |
333 | 1 | 0 | 3522 | 0 | 0 | 0 | 0 | 0 |
334 | 1 | 0 | 2943 | 0 | 1 | 0 | 0 | 0 |
335 | 1 | 1 | 2404 | 0 | 1 | 0 | 1 | 114 |
336 | 1 | 1 | 2278 | 1 | 1 | 0 | 0 | 0 |
337 | 1 | 0 | 1710 | 1 | 0 | 0 | 0 | 0 |
338 | 1 | 1 | 833 | 0 | 0 | 0 | 0 | 0 |
339 | 1 | 1 | 2529 | 0 | 0 | 0 | 0 | 0 |
340 | 1 | 1 | 868 | 0 | 1 | 0 | 0 | 0 |
341 | 1 | 2 | 916 | 1 | 0 | 1 | 1 | 43 |
342 | 1 | 0 | 2539 | 0 | 1 | 0 | 0 | 0 |
343 | 1 | 1 | 2299 | 0 | 1 | 0 | 0 | 0 |
344 | 1 | 1 | 1864 | 0 | 0 | 0 | 1 | 30 |
345 | 0 | 2 | 295 | 0 | 0 | 0 | 1 | 164 |
346 | 1 | 2 | 1128 | 0 | 1 | 0 | 1 | 248 |
347 | 1 | 4 | 492 | 1 | 1 | 1 | 1 | 139 |
348 | 1 | 1 | 3334 | 0 | 1 | 0 | 0 | 0 |
349 | 1 | 1 | 2962 | 1 | 1 | 0 | 1 | 96 |
350 | 1 | 1 | 4157 | 0 | 1 | 0 | 1 | 98 |
351 | 1 | 1 | 891 | 1 | 1 | 0 | 1 | 81 |
352 | 1 | 3 | 2065 | 0 | 1 | 0 | 1 | 197 |
353 | 1 | 1 | 1346 | 0 | 1 | 1 | 0 | 0 |
354 | 1 | 2 | 2054 | 1 | 1 | 0 | 1 | 25 |
355 | 0 | 3 | 1134 | 0 | 0 | 1 | 1 | 314 |
356 | 1 | 1 | 2831 | 1 | 1 | 0 | 0 | 0 |
357 | 1 | 2 | 681 | 0 | 0 | 1 | 0 | 0 |
358 | 1 | 1 | 3185 | 0 | 1 | 1 | 0 | 0 |
359 | 1 | 1 | 3548 | 0 | 0 | 0 | 0 | 0 |
360 | 1 | 0 | 304 | 0 | 1 | 1 | 0 | 0 |
361 | 1 | 2 | 3382 | 0 | 0 | 0 | 0 | 0 |
362 | 0 | 3 | 841 | 1 | 1 | 1 | 1 | 50 |
363 | 1 | 0 | 2744 | 1 | 0 | 0 | 0 | 0 |
364 | 0 | 1 | 2073 | 0 | 0 | 0 | 0 | 0 |
365 | 1 | 2 | 3655 | 0 | 1 | 0 | 1 | 35 |
366 | 0 | 1 | 1463 | 1 | 1 | 0 | 0 | 0 |
367 | 1 | 1 | 3011 | 0 | 1 | 0 | 0 | 0 |
368 | 1 | 1 | 2802 | 1 | 1 | 0 | 1 | 161 |
369 | 1 | 3 | 2016 | 1 | 0 | 0 | 1 | 1353 |
370 | 1 | 10 | 430 | 1 | 1 | 0 | 1 | 1184 |
371 | 1 | 1 | 3146 | 1 | 0 | 0 | 1 | 10 |
372 | 1 | 0 | 3507 | 0 | 0 | 0 | 0 | 0 |
373 | 1 | 2 | 1536 | 0 | 1 | 0 | 1 | 1500 |
374 | 1 | 1 | 3248 | 0 | 0 | 1 | 1 | 132 |
375 | 1 | 1 | 3095 | 0 | 0 | 0 | 0 | 0 |
376 | 0 | 1 | 3235 | 1 | 1 | 1 | 1 | 110 |
377 | 1 | 2 | 1031 | 0 | 1 | 0 | 1 | 191 |
378 | 1 | 3 | 2201 | 0 | 0 | 0 | 1 | 99 |
379 | 1 | 1 | 2529 | 1 | 0 | 0 | 1 | 35 |
380 | 1 | 3 | 3129 | 0 | 1 | 0 | 1 | 217 |
381 | 1 | 2 | 3283 | 1 | 0 | 0 | 1 | 128 |
382 | 1 | 1 | 1646 | 1 | 0 | 1 | 0 | 0 |
383 | 1 | 0 | 3401 | 0 | 0 | 0 | 0 | 0 |
384 | 0 | 3 | 479 | 0 | 0 | 0 | 1 | 459 |
385 | 1 | 1 | 1192 | 1 | 1 | 0 | 1 | 44 |
386 | 1 | 1 | 314 | 0 | 1 | 0 | 1 | 149 |
387 | 0 | 2 | 506 | 1 | 1 | 0 | 1 | 282 |
388 | 1 | 2 | 2072 | 0 | 0 | 0 | 1 | 105 |
389 | 1 | 1 | 2618 | 1 | 1 | 1 | 1 | 6 |
390 | 1 | 1 | 9 | 0 | 1 | 1 | 1 | 196 |
391 | 1 | 1 | 3278 | 1 | 0 | 0 | 1 | 153 |
392 | 1 | 1 | 217 | 0 | 0 | 1 | 0 | 0 |
393 | 1 | 3 | 1529 | 0 | 1 | 1 | 1 | 424 |
394 | 1 | 3 | 3583 | 0 | 0 | 0 | 1 | 205 |
395 | 1 | 2 | 1856 | 0 | 0 | 1 | 0 | 0 |
396 | 1 | 0 | 2632 | 0 | 1 | 0 | 0 | 0 |
397 | 1 | 2 | 1415 | 0 | 1 | 1 | 0 | 0 |
398 | 1 | 0 | 1249 | 0 | 0 | 0 | 0 | 0 |
399 | 1 | 0 | 3159 | 1 | 0 | 0 | 0 | 0 |
400 | 0 | 2 | 458 | 1 | 1 | 0 | 1 | 114 |
401 | 0 | 2 | 864 | 0 | 1 | 0 | 1 | 163 |
402 | 1 | 0 | 3747 | 0 | 0 | 0 | 0 | 0 |
403 | 1 | 2 | 3975 | 1 | 1 | 0 | 1 | 170 |
404 | 1 | 2 | 2044 | 1 | 1 | 1 | 1 | 136 |
405 | 1 | 2 | 1646 | 0 | 1 | 1 | 1 | 83 |
406 | 1 | 0 | 1709 | 0 | 0 | 0 | 0 | 0 |
407 | 1 | 1 | 2404 | 0 | 0 | 0 | 1 | 114 |
408 | 1 | 0 | 2765 | 0 | 0 | 0 | 0 | 0 |
409 | 1 | 1 | 906 | 1 | 0 | 1 | 1 | 141 |
410 | 1 | 2 | 1498 | 1 | 0 | 1 | 1 | 40 |
411 | 0 | 0 | 2891 | 0 | 1 | 0 | 0 | 0 |
412 | 0 | 2 | 2744 | 1 | 0 | 0 | 1 | 86 |
413 | 1 | 1 | 1646 | 0 | 1 | 1 | 0 | 0 |
414 | 1 | 1 | 1240 | 0 | 0 | 0 | 0 | 0 |
415 | 1 | 0 | 2802 | 0 | 1 | 0 | 0 | 0 |
416 | 1 | 0 | 534 | 0 | 1 | 1 | 0 | 0 |
417 | 1 | 2 | 1346 | 0 | 0 | 1 | 0 | 0 |
418 | 1 | 3 | 1842 | 1 | 1 | 1 | 1 | 169 |
419 | 1 | 2 | 1780 | 0 | 0 | 1 | 0 | 0 |
420 | 1 | 2 | 247 | 0 | 0 | 0 | 1 | 324 |
421 | 1 | 1 | 2919 | 0 | 1 | 1 | 0 | 0 |
422 | 1 | 0 | 2735 | 0 | 1 | 0 | 0 | 0 |
423 | 1 | 1 | 758 | 0 | 1 | 0 | 1 | 83 |
424 | 1 | 4 | 1122 | 0 | 0 | 0 | 1 | 274 |
425 | 0 | 3 | 1137 | 0 | 0 | 1 | 1 | 287 |
426 | 1 | 1 | 644 | 0 | 1 | 1 | 0 | 0 |
427 | 0 | 4 | 1794 | 1 | 1 | 1 | 1 | 259 |
428 | 1 | 1 | 3125 | 1 | 0 | 0 | 1 | 103 |
429 | 1 | 1 | 974 | 0 | 0 | 0 | 0 | 0 |
430 | 0 | 0 | 3747 | 0 | 1 | 0 | 0 | 0 |
431 | 1 | 2 | 216 | 1 | 0 | 0 | 1 | 134 |
432 | 1 | 4 | 1498 | 0 | 1 | 1 | 1 | 209 |
433 | 1 | 3 | 1739 | 0 | 1 | 0 | 1 | 226 |
434 | 1 | 0 | 345 | 1 | 1 | 0 | 0 | 0 |
435 | 1 | 2 | 1801 | 1 | 1 | 0 | 0 | 0 |
436 | 1 | 1 | 216 | 1 | 1 | 0 | 0 | 0 |
437 | 1 | 2 | 1130 | 0 | 0 | 0 | 1 | 252 |
438 | 0 | 1 | 1612 | 0 | 0 | 0 | 1 | 43 |
439 | 1 | 3 | 1568 | 1 | 0 | 0 | 1 | 344 |
440 | 1 | 1 | 2102 | 1 | 1 | 0 | 1 | 54 |
441 | 1 | 1 | 2863 | 0 | 0 | 0 | 0 | 0 |
442 | 1 | 1 | 535 | 0 | 1 | 1 | 1 | 149 |
443 | 1 | 1 | 3430 | 0 | 0 | 0 | 0 | 0 |
444 | 0 | 1 | 1207 | 0 | 1 | 0 | 0 | 0 |
445 | 1 | 1 | 486 | 0 | 1 | 0 | 1 | 30 |
446 | 1 | 1 | 3262 | 1 | 1 | 0 | 1 | 158 |
447 | 1 | 1 | 2249 | 0 | 0 | 0 | 1 | 54 |
448 | 0 | 3 | 2647 | 0 | 1 | 0 | 1 | 1255 |
449 | 1 | 2 | 3593 | 0 | 1 | 0 | 1 | 98 |
450 | 1 | 1 | 1890 | 0 | 0 | 0 | 1 | 85 |
451 | 1 | 1 | 3306 | 0 | 0 | 0 | 0 | 0 |
452 | 1 | 0 | 3747 | 0 | 0 | 0 | 0 | 0 |
453 | 0 | 1 | 4188 | 1 | 1 | 0 | 1 | 137 |
454 | 1 | 1 | 2772 | 1 | 0 | 0 | 1 | 135 |
455 | 1 | 2 | 1261 | 0 | 0 | 0 | 1 | 44 |
456 | 1 | 1 | 827 | 1 | 1 | 0 | 1 | 163 |
457 | 1 | 2 | 3590 | 1 | 0 | 0 | 1 | 116 |
458 | 1 | 2 | 1120 | 1 | 0 | 1 | 1 | 172 |
459 | 1 | 3 | 1570 | 1 | 0 | 0 | 1 | 104 |
460 | 1 | 2 | 1253 | 0 | 1 | 0 | 0 | 0 |
461 | 1 | 1 | 2977 | 0 | 0 | 0 | 1 | 70 |
462 | 0 | 2 | 458 | 1 | 0 | 0 | 1 | 271 |
463 | 1 | 1 | 1962 | 1 | 1 | 1 | 0 | 0 |
464 | 1 | 1 | 3255 | 1 | 1 | 0 | 0 | 0 |
465 | 0 | 1 | 1091 | 0 | 0 | 0 | 0 | 0 |
466 | 1 | 1 | 1010 | 0 | 0 | 0 | 0 | 0 |
467 | 1 | 1 | 2991 | 1 | 0 | 1 | 1 | 184 |
468 | 1 | 0 | 2740 | 0 | 0 | 0 | 0 | 0 |
469 | 1 | 0 | 2728 | 1 | 1 | 0 | 0 | 0 |
470 | 1 | 1 | 4065 | 0 | 1 | 0 | 0 | 0 |
471 | 1 | 1 | 2102 | 1 | 0 | 0 | 1 | 161 |
472 | 0 | 0 | 220 | 0 | 1 | 0 | 0 | 0 |
473 | 1 | 1 | 216 | 0 | 1 | 0 | 0 | 0 |
474 | 1 | 2 | 2950 | 0 | 1 | 0 | 0 | 0 |
475 | 1 | 1 | 23 | 0 | 1 | 0 | 0 | 0 |
476 | 1 | 1 | 2999 | 1 | 0 | 0 | 1 | 34 |
477 | 1 | 3 | 463 | 1 | 1 | 0 | 1 | 322 |
478 | 1 | 1 | 743 | 0 | 0 | 0 | 0 | 0 |
479 | 1 | 1 | 700 | 1 | 1 | 1 | 0 | 0 |
480 | 1 | 2 | 197 | 1 | 0 | 0 | 0 | 0 |
481 | 1 | 3 | 301 | 0 | 0 | 0 | 1 | 1249 |
482 | 1 | 0 | 2905 | 1 | 0 | 0 | 0 | 0 |
483 | 0 | 1 | 665 | 0 | 1 | 1 | 0 | 0 |
484 | 1 | 1 | 308 | 0 | 1 | 0 | 1 | 40 |
485 | 1 | 0 | 2735 | 0 | 0 | 0 | 0 | 0 |
486 | 1 | 1 | 2415 | 1 | 1 | 0 | 1 | 35 |
487 | 1 | 1 | 113 | 1 | 0 | 0 | 1 | 308 |
488 | 1 | 4 | 1653 | 1 | 0 | 1 | 1 | 192 |
489 | 1 | 0 | 3507 | 0 | 1 | 0 | 0 | 0 |
490 | 1 | 2 | 1708 | 1 | 1 | 0 | 1 | 122 |
491 | 1 | 1 | 3822 | 0 | 1 | 0 | 0 | 0 |
492 | 0 | 2 | 1976 | 0 | 0 | 0 | 1 | 43 |
493 | 1 | 5 | 240 | 0 | 0 | 1 | 1 | 588 |
494 | 1 | 4 | 2481 | 1 | 0 | 0 | 1 | 160 |
495 | 1 | 0 | 2877 | 0 | 1 | 0 | 0 | 0 |
496 | 1 | 2 | 2812 | 0 | 0 | 0 | 1 | 183 |
497 | 1 | 0 | 1227 | 0 | 1 | 0 | 0 | 0 |
498 | 1 | 3 | 2052 | 0 | 0 | 0 | 1 | 200 |
499 | 1 | 2 | 2746 | 1 | 0 | 1 | 1 | 182 |
500 | 0 | 1 | 2236 | 0 | 0 | 0 | 0 | 0 |
501 | 1 | 1 | 216 | 0 | 1 | 0 | 0 | 0 |
502 | 1 | 0 | 1451 | 0 | 0 | 0 | 0 | 0 |
503 | 0 | 0 | 3353 | 1 | 1 | 0 | 0 | 0 |
504 | 1 | 1 | 206 | 0 | 0 | 0 | 0 | 0 |
505 | 1 | 2 | 2962 | 1 | 1 | 0 | 1 | 95 |
506 | 1 | 1 | 3655 | 0 | 1 | 0 | 0 | 0 |
507 | 0 | 0 | 3107 | 0 | 0 | 0 | 0 | 0 |
508 | 1 | 1 | 3549 | 1 | 1 | 0 | 1 | 229 |
509 | 1 | 0 | 1710 | 0 | 1 | 0 | 0 | 0 |
510 | 1 | 1 | 196 | 0 | 1 | 0 | 0 | 0 |
511 | 1 | 1 | 3020 | 1 | 0 | 0 | 1 | 129 |
512 | 1 | 0 | 3747 | 0 | 1 | 0 | 0 | 0 |
513 | 1 | 0 | 2767 | 1 | 1 | 0 | 0 | 0 |
514 | 0 | 0 | 3747 | 1 | 0 | 0 | 0 | 0 |
515 | 1 | 0 | 2730 | 0 | 0 | 0 | 0 | 0 |
516 | 1 | 3 | 1592 | 1 | 1 | 1 | 1 | 30 |
517 | 1 | 1 | 681 | 1 | 0 | 1 | 0 | 0 |
518 | 1 | 2 | 3718 | 0 | 0 | 0 | 1 | 98 |
519 | 1 | 2 | 1379 | 0 | 0 | 1 | 1 | 63 |
520 | 1 | 3 | 148 | 0 | 1 | 1 | 1 | 214 |
521 | 1 | 0 | 3353 | 1 | 1 | 0 | 0 | 0 |
522 | 0 | 1 | 206 | 1 | 0 | 0 | 1 | 264 |
523 | 1 | 0 | 2781 | 0 | 0 | 0 | 0 | 0 |
524 | 1 | 1 | 216 | 1 | 0 | 0 | 0 | 0 |
525 | 0 | 0 | 3159 | 0 | 1 | 1 | 0 | 0 |
526 | 1 | 1 | 1792 | 1 | 0 | 0 | 1 | 167 |
527 | 1 | 1 | 2845 | 0 | 1 | 0 | 1 | 120 |
528 | 1 | 1 | 2607 | 1 | 0 | 0 | 1 | 6 |
529 | 1 | 1 | 118 | 0 | 0 | 0 | 0 | 0 |
530 | 1 | 2 | 1498 | 0 | 1 | 1 | 0 | 0 |
531 | 0 | 0 | 3489 | 0 | 1 | 0 | 0 | 0 |
532 | 1 | 1 | 1008 | 0 | 0 | 0 | 0 | 0 |
533 | 0 | 1 | 17 | 1 | 0 | 0 | 1 | 94 |
534 | 1 | 2 | 491 | 1 | 1 | 1 | 1 | 139 |
535 | 1 | 1 | 2941 | 1 | 1 | 0 | 0 | 0 |
536 | 1 | 1 | 1536 | 0 | 0 | 0 | 0 | 0 |
537 | 0 | 2 | 3513 | 1 | 1 | 0 | 1 | 219 |
538 | 1 | 1 | 1184 | 0 | 1 | 0 | 1 | 44 |
539 | 0 | 2 | 3636 | 1 | 1 | 0 | 1 | 128 |
540 | 1 | 0 | 3745 | 1 | 0 | 0 | 0 | 0 |
541 | 0 | 2 | 1995 | 1 | 0 | 1 | 1 | 243 |
542 | 0 | 1 | 2102 | 1 | 1 | 0 | 1 | 171 |
543 | 1 | 1 | 1851 | 1 | 1 | 0 | 1 | 30 |
544 | 1 | 1 | 3684 | 0 | 1 | 0 | 0 | 0 |
545 | 1 | 1 | 3027 | 1 | 0 | 0 | 1 | 96 |
546 | 0 | 0 | 3747 | 1 | 0 | 0 | 0 | 0 |
547 | 1 | 3 | 2957 | 0 | 1 | 0 | 1 | 130 |
548 | 1 | 1 | 2918 | 1 | 1 | 0 | 0 | 0 |
549 | 1 | 0 | 3401 | 1 | 0 | 0 | 0 | 0 |
550 | 1 | 1 | 2355 | 0 | 0 | 0 | 0 | 0 |
551 | 0 | 1 | 2220 | 0 | 1 | 0 | 1 | 171 |
552 | 0 | 0 | 3746 | 0 | 1 | 0 | 0 | 0 |
553 | 0 | 5 | 519 | 1 | 0 | 0 | 1 | 585 |
554 | 1 | 0 | 2740 | 0 | 1 | 0 | 0 | 0 |
555 | 0 | 2 | 2142 | 0 | 0 | 1 | 1 | 174 |
556 | 1 | 1 | 597 | 1 | 0 | 0 | 1 | 240 |
557 | 0 | 0 | 3394 | 1 | 1 | 0 | 0 | 0 |
558 | 1 | 1 | 1618 | 1 | 0 | 1 | 0 | 0 |
559 | 1 | 1 | 1168 | 1 | 0 | 0 | 1 | 40 |
560 | 1 | 0 | 3694 | 1 | 0 | 0 | 0 | 0 |
561 | 1 | 5 | 1374 | 0 | 1 | 0 | 1 | 369 |
562 | 1 | 2 | 1995 | 1 | 0 | 1 | 1 | 117 |
563 | 1 | 0 | 3507 | 0 | 0 | 0 | 0 | 0 |
564 | 1 | 1 | 1415 | 0 | 0 | 1 | 0 | 0 |
565 | 1 | 1 | 204 | 0 | 1 | 0 | 0 | 0 |
566 | 1 | 1 | 3201 | 1 | 1 | 0 | 1 | 129 |
567 | 1 | 3 | 2872 | 1 | 0 | 0 | 1 | 122 |
568 | 1 | 1 | 1795 | 0 | 0 | 0 | 1 | 230 |
569 | 1 | 1 | 581 | 1 | 0 | 0 | 1 | 331 |
570 | 1 | 0 | 3680 | 0 | 1 | 0 | 0 | 0 |
571 | 0 | 2 | 2459 | 1 | 1 | 0 | 1 | 153 |
572 | 1 | 2 | 1842 | 0 | 0 | 1 | 0 | 0 |
573 | 1 | 0 | 3747 | 0 | 1 | 0 | 0 | 0 |
574 | 1 | 5 | 216 | 1 | 1 | 1 | 1 | 123 |
575 | 0 | 2 | 2831 | 1 | 1 | 0 | 1 | 170 |
576 | 1 | 1 | 2417 | 1 | 1 | 0 | 1 | 130 |
577 | 1 | 1 | 3944 | 1 | 1 | 0 | 1 | 128 |
578 | 1 | 2 | 1646 | 1 | 0 | 1 | 1 | 130 |
579 | 1 | 1 | 2564 | 0 | 0 | 0 | 1 | 130 |
580 | 1 | 2 | 3374 | 1 | 0 | 1 | 1 | 128 |
581 | 1 | 2 | 3759 | 0 | 1 | 0 | 0 | 0 |
582 | 1 | 3 | 1134 | 1 | 0 | 0 | 1 | 406 |
583 | 1 | 1 | 1680 | 1 | 1 | 0 | 1 | 116 |
584 | 1 | 0 | 1870 | 0 | 1 | 0 | 0 | 0 |
585 | 1 | 3 | 465 | 0 | 1 | 0 | 1 | 133 |
586 | 1 | 2 | 3642 | 0 | 0 | 0 | 1 | 129 |
587 | 0 | 3 | 260 | 0 | 0 | 1 | 1 | 135 |
588 | 1 | 2 | 3914 | 0 | 0 | 1 | 1 | 581 |
589 | 0 | 2 | 3521 | 0 | 0 | 0 | 1 | 53 |
590 | 1 | 2 | 1850 | 0 | 0 | 0 | 1 | 35 |
591 | 1 | 0 | 181 | 1 | 0 | 0 | 0 | 0 |
592 | 1 | 1 | 2257 | 1 | 0 | 0 | 1 | 91 |
593 | 1 | 1 | 2809 | 0 | 1 | 0 | 0 | 0 |
594 | 1 | 2 | 1842 | 1 | 0 | 1 | 0 | 0 |
595 | 1 | 1 | 2878 | 0 | 0 | 0 | 0 | 0 |
596 | 1 | 2 | 2199 | 1 | 1 | 0 | 1 | 81 |
597 | 1 | 1 | 2445 | 1 | 0 | 1 | 1 | 35 |
598 | 1 | 1 | 3208 | 0 | 0 | 0 | 0 | 0 |
599 | 1 | 1 | 2481 | 1 | 1 | 0 | 1 | 153 |
600 | 1 | 1 | 216 | 1 | 0 | 1 | 0 | 0 |
601 | 1 | 7 | 216 | 1 | 1 | 1 | 1 | 150 |
602 | 1 | 1 | 3199 | 1 | 0 | 0 | 1 | 159 |
603 | 1 | 1 | 2781 | 1 | 0 | 0 | 1 | 130 |
604 | 1 | 1 | 2229 | 0 | 1 | 0 | 0 | 0 |
605 | 0 | 0 | 238 | 1 | 1 | 1 | 0 | 0 |
606 | 1 | 0 | 3398 | 0 | 1 | 0 | 0 | 0 |
607 | 1 | 2 | 2326 | 1 | 1 | 0 | 1 | 161 |
608 | 1 | 1 | 3630 | 0 | 1 | 0 | 0 | 0 |
609 | 1 | 1 | 2751 | 1 | 1 | 0 | 0 | 0 |
610 | 1 | 1 | 532 | 0 | 0 | 1 | 0 | 0 |
611 | 1 | 1 | 3548 | 0 | 0 | 0 | 0 | 0 |
612 | 0 | 1 | 2962 | 0 | 0 | 0 | 1 | 136 |
613 | 1 | 1 | 90 | 1 | 0 | 1 | 0 | 0 |
614 | 1 | 1 | 2863 | 0 | 1 | 0 | 0 | 0 |
615 | 1 | 2 | 162 | 1 | 1 | 0 | 1 | 1162 |
616 | 0 | 0 | 2257 | 0 | 1 | 0 | 0 | 0 |
617 | 1 | 0 | 2877 | 0 | 0 | 0 | 0 | 0 |
618 | 1 | 12 | 520 | 1 | 0 | 0 | 1 | 1195 |
619 | 0 | 1 | 2878 | 0 | 1 | 0 | 0 | 0 |
620 | 1 | 0 | 3522 | 0 | 1 | 0 | 0 | 0 |
621 | 0 | 1 | 1491 | 0 | 0 | 0 | 1 | 57 |
622 | 1 | 2 | 1837 | 1 | 0 | 1 | 0 | 0 |
623 | 1 | 1 | 717 | 1 | 0 | 0 | 1 | 30 |
624 | 1 | 3 | 3740 | 0 | 0 | 0 | 1 | 98 |
625 | 1 | 2 | 2957 | 0 | 1 | 0 | 1 | 129 |
626 | 1 | 0 | 3745 | 0 | 1 | 0 | 0 | 0 |
627 | 1 | 2 | 3213 | 1 | 0 | 1 | 1 | 34 |
628 | 1 | 1 | 1554 | 0 | 0 | 0 | 1 | 277 |
629 | 1 | 0 | 1598 | 0 | 0 | 0 | 0 | 0 |
630 | 1 | 1 | 554 | 1 | 0 | 0 | 0 | 0 |
631 | 1 | 1 | 3791 | 1 | 0 | 0 | 0 | 0 |
632 | 1 | 0 | 1709 | 0 | 0 | 0 | 0 | 0 |
633 | 1 | 1 | 1885 | 0 | 1 | 0 | 0 | 0 |
634 | 1 | 1 | 4065 | 1 | 1 | 0 | 1 | 128 |
635 | 1 | 2 | 850 | 1 | 1 | 0 | 1 | 210 |
636 | 1 | 5 | 15 | 0 | 1 | 0 | 1 | 142 |
637 | 1 | 1 | 3390 | 1 | 1 | 0 | 0 | 0 |
638 | 1 | 1 | 2361 | 0 | 1 | 0 | 0 | 0 |
639 | 0 | 1 | 2704 | 0 | 1 | 0 | 0 | 0 |
640 | 1 | 0 | 3680 | 0 | 0 | 0 | 0 | 0 |
641 | 1 | 1 | 120 | 0 | 1 | 0 | 1 | 149 |
642 | 1 | 1 | 3478 | 0 | 0 | 0 | 0 | 0 |
643 | 1 | 1 | 582 | 0 | 1 | 0 | 1 | 69 |
644 | 1 | 1 | 2704 | 1 | 1 | 0 | 1 | 8 |
645 | 1 | 7 | 1128 | 1 | 1 | 1 | 1 | 236 |
646 | 1 | 2 | 3944 | 0 | 1 | 1 | 1 | 362 |
647 | 0 | 0 | 3094 | 0 | 1 | 1 | 0 | 0 |
648 | 0 | 2 | 114 | 1 | 1 | 0 | 0 | 0 |
649 | 1 | 2 | 3381 | 1 | 0 | 0 | 1 | 98 |
650 | 1 | 1 | 3158 | 0 | 0 | 0 | 1 | 34 |
651 | 1 | 1 | 2759 | 0 | 1 | 0 | 1 | 35 |
652 | 1 | 2 | 1752 | 0 | 1 | 1 | 0 | 0 |
653 | 1 | 3 | 792 | 0 | 1 | 0 | 1 | 331 |
654 | 1 | 0 | 3394 | 0 | 1 | 0 | 0 | 0 |
655 | 1 | 0 | 4096 | 0 | 1 | 0 | 0 | 0 |
656 | 1 | 1 | 3327 | 1 | 1 | 1 | 1 | 98 |
657 | 1 | 1 | 2848 | 0 | 0 | 0 | 0 | 0 |
658 | 1 | 1 | 331 | 1 | 1 | 0 | 0 | 0 |
659 | 0 | 1 | 2662 | 0 | 0 | 0 | 0 | 0 |
660 | 1 | 1 | 1032 | 1 | 1 | 0 | 0 | 0 |
661 | 0 | 1 | 2466 | 0 | 1 | 0 | 0 | 0 |
662 | 1 | 0 | 3171 | 0 | 1 | 1 | 0 | 0 |
663 | 1 | 0 | 2891 | 1 | 0 | 0 | 0 | 0 |
664 | 0 | 2 | 3157 | 1 | 1 | 0 | 1 | 162 |
665 | 1 | 2 | 490 | 1 | 0 | 0 | 1 | 276 |
666 | 1 | 2 | 2012 | 1 | 1 | 1 | 1 | 160 |
667 | 0 | 1 | 2487 | 1 | 0 | 0 | 1 | 163 |
668 | 1 | 1 | 3342 | 0 | 1 | 0 | 1 | 98 |
669 | 1 | 1 | 2787 | 1 | 0 | 0 | 0 | 0 |
670 | 1 | 0 | 1164 | 0 | 1 | 0 | 0 | 0 |
671 | 1 | 1 | 2872 | 0 | 1 | 0 | 0 | 0 |
672 | 0 | 1 | 1091 | 1 | 1 | 0 | 0 | 0 |
673 | 1 | 0 | 4157 | 1 | 0 | 0 | 0 | 0 |
674 | 1 | 3 | 2829 | 0 | 1 | 0 | 1 | 163 |
675 | 1 | 2 | 1346 | 0 | 1 | 1 | 0 | 0 |
676 | 0 | 1 | 1017 | 0 | 0 | 0 | 1 | 197 |
677 | 1 | 1 | 3024 | 0 | 1 | 0 | 0 | 0 |
678 | 1 | 1 | 581 | 0 | 1 | 1 | 1 | 203 |
679 | 1 | 0 | 2221 | 0 | 1 | 0 | 0 | 0 |
680 | 1 | 1 | 3397 | 1 | 1 | 0 | 0 | 0 |
681 | 1 | 4 | 2807 | 1 | 0 | 1 | 1 | 239 |
682 | 1 | 1 | 3041 | 1 | 0 | 0 | 1 | 30 |
683 | 1 | 0 | 3423 | 1 | 1 | 0 | 0 | 0 |
684 | 1 | 2 | 1582 | 0 | 1 | 1 | 0 | 0 |
685 | 1 | 3 | 441 | 0 | 1 | 0 | 0 | 0 |
686 | 1 | 1 | 3397 | 0 | 0 | 0 | 0 | 0 |
687 | 1 | 2 | 2723 | 0 | 0 | 0 | 1 | 161 |
688 | 1 | 1 | 3397 | 1 | 0 | 0 | 0 | 0 |
689 | 0 | 1 | 2878 | 1 | 1 | 0 | 0 | 0 |
690 | 1 | 1 | 2402 | 0 | 1 | 0 | 1 | 85 |
691 | 1 | 1 | 709 | 1 | 0 | 0 | 1 | 160 |
692 | 1 | 1 | 1962 | 0 | 0 | 1 | 0 | 0 |
693 | 1 | 1 | 1673 | 1 | 0 | 0 | 1 | 129 |
694 | 1 | 3 | 804 | 1 | 0 | 1 | 0 | 0 |
695 | 1 | 1 | 2379 | 0 | 1 | 0 | 1 | 207 |
696 | 1 | 3 | 1248 | 0 | 0 | 1 | 1 | 202 |
697 | 1 | 1 | 3883 | 1 | 0 | 0 | 1 | 97 |
698 | 1 | 3 | 1809 | 1 | 1 | 1 | 1 | 58 |
699 | 1 | 0 | 3852 | 0 | 1 | 0 | 0 | 0 |
700 | 1 | 0 | 136 | 0 | 1 | 0 | 0 | 0 |
701 | 1 | 1 | 994 | 0 | 1 | 0 | 0 | 0 |
702 | 1 | 5 | 549 | 1 | 1 | 1 | 1 | 484 |
703 | 1 | 1 | 2941 | 0 | 0 | 0 | 1 | 285 |
704 | 1 | 1 | 609 | 0 | 1 | 0 | 1 | 162 |
705 | 1 | 3 | 2158 | 1 | 1 | 0 | 1 | 64 |
706 | 0 | 0 | 2768 | 0 | 1 | 0 | 0 | 0 |
707 | 0 | 1 | 1052 | 0 | 1 | 0 | 1 | 170 |
708 | 1 | 2 | 2466 | 1 | 0 | 0 | 1 | 188 |
709 | 1 | 0 | 3558 | 0 | 0 | 0 | 0 | 0 |
710 | 1 | 2 | 1882 | 0 | 0 | 1 | 0 | 0 |
711 | 1 | 1 | 2897 | 1 | 0 | 0 | 0 | 1 |
712 | 1 | 2 | 1563 | 0 | 0 | 0 | 1 | 160 |
713 | 1 | 1 | 3166 | 1 | 0 | 1 | 0 | 0 |
714 | 1 | 0 | 1072 | 0 | 0 | 0 | 0 | 0 |
715 | 1 | 1 | 3650 | 0 | 0 | 0 | 0 | 0 |
716 | 1 | 2 | 465 | 1 | 0 | 1 | 1 | 260 |
717 | 1 | 1 | 3496 | 0 | 1 | 0 | 1 | 40 |
718 | 0 | 1 | 3597 | 0 | 1 | 0 | 0 | 0 |
719 | 1 | 0 | 3507 | 0 | 0 | 0 | 0 | 0 |
720 | 1 | 2 | 841 | 0 | 1 | 1 | 0 | 0 |
721 | 1 | 4 | 1128 | 0 | 1 | 0 | 1 | 620 |
722 | 1 | 2 | 3382 | 1 | 1 | 0 | 1 | 132 |
723 | 1 | 1 | 1058 | 0 | 0 | 1 | 0 | 0 |
724 | 1 | 1 | 582 | 1 | 1 | 0 | 1 | 89 |
725 | 1 | 2 | 1582 | 1 | 0 | 1 | 0 | 0 |
726 | 1 | 1 | 2313 | 0 | 1 | 0 | 1 | 140 |
727 | 0 | 1 | 1437 | 0 | 1 | 0 | 0 | 0 |
728 | 1 | 2 | 1879 | 0 | 1 | 1 | 0 | 0 |
729 | 1 | 1 | 4036 | 0 | 0 | 0 | 1 | 65 |
730 | 0 | 1 | 770 | 0 | 1 | 0 | 1 | 43 |
731 | 1 | 1 | 869 | 1 | 1 | 0 | 0 | 0 |
732 | 0 | 1 | 1521 | 0 | 0 | 0 | 0 | 0 |
733 | 1 | 2 | 1120 | 1 | 0 | 0 | 1 | 193 |
734 | 1 | 2 | 1498 | 1 | 0 | 1 | 1 | 344 |
735 | 0 | 1 | 2634 | 0 | 1 | 0 | 1 | 116 |
736 | 1 | 2 | 1120 | 0 | 1 | 0 | 1 | 124 |
737 | 1 | 1 | 2452 | 1 | 1 | 0 | 1 | 100 |
738 | 1 | 2 | 3769 | 0 | 0 | 0 | 0 | 0 |
739 | 0 | 3 | 1436 | 1 | 0 | 1 | 1 | 192 |
740 | 1 | 2 | 1625 | 0 | 0 | 0 | 1 | 169 |
741 | 1 | 1 | 608 | 0 | 1 | 0 | 0 | 0 |
742 | 1 | 0 | 1112 | 0 | 0 | 0 | 0 | 0 |
743 | 1 | 2 | 3054 | 0 | 1 | 1 | 1 | 99 |
744 | 1 | 3 | 1130 | 0 | 0 | 0 | 1 | 274 |
745 | 1 | 3 | 3583 | 0 | 0 | 0 | 1 | 100 |
746 | 1 | 2 | 3695 | 0 | 1 | 0 | 1 | 129 |
747 | 1 | 1 | 1968 | 0 | 0 | 0 | 0 | 0 |
748 | 1 | 1 | 1233 | 0 | 1 | 0 | 0 | 0 |
749 | 1 | 1 | 2158 | 1 | 0 | 0 | 0 | 0 |
750 | 1 | 4 | 567 | 1 | 0 | 1 | 1 | 410 |
751 | 1 | 0 | 104 | 0 | 0 | 0 | 0 | 0 |
752 | 1 | 2 | 3660 | 0 | 1 | 0 | 0 | 0 |
753 | 1 | 1 | 2947 | 0 | 1 | 0 | 1 | 230 |
754 | 1 | 1 | 4157 | 1 | 0 | 0 | 1 | 33 |
755 | 1 | 2 | 3436 | 0 | 0 | 0 | 1 | 98 |
756 | 0 | 2 | 1801 | 0 | 0 | 1 | 0 | 0 |
757 | 0 | 0 | 3107 | 1 | 1 | 0 | 0 | 0 |
758 | 1 | 0 | 3201 | 0 | 1 | 1 | 0 | 0 |
759 | 1 | 1 | 198 | 1 | 0 | 0 | 0 | 0 |
760 | 1 | 0 | 3746 | 0 | 0 | 0 | 0 | 0 |
761 | 1 | 3 | 3570 | 1 | 0 | 0 | 1 | 98 |
762 | 1 | 2 | 3670 | 1 | 1 | 0 | 1 | 19 |
763 | 1 | 1 | 1240 | 0 | 1 | 0 | 0 | 0 |
764 | 1 | 0 | 3311 | 0 | 1 | 0 | 0 | 0 |
765 | 0 | 3 | 1442 | 0 | 0 | 1 | 1 | 79 |
766 | 1 | 0 | 71 | 0 | 0 | 0 | 0 | 0 |
767 | 0 | 0 | 653 | 0 | 0 | 0 | 0 | 0 |
768 | 1 | 1 | 1605 | 1 | 1 | 0 | 1 | 85 |
769 | 1 | 3 | 1164 | 1 | 1 | 0 | 1 | 349 |
770 | 1 | 1 | 829 | 0 | 1 | 0 | 0 | 0 |
771 | 1 | 1 | 2905 | 0 | 0 | 0 | 0 | 0 |
772 | 1 | 1 | 216 | 0 | 1 | 1 | 0 | 0 |
773 | 1 | 0 | 2773 | 1 | 1 | 0 | 0 | 0 |
774 | 1 | 0 | 2735 | 0 | 1 | 0 | 0 | 0 |
775 | 1 | 1 | 1991 | 0 | 0 | 0 | 1 | 103 |
776 | 1 | 1 | 3264 | 0 | 1 | 0 | 1 | 39 |
777 | 0 | 2 | 822 | 1 | 0 | 0 | 1 | 71 |
778 | 1 | 1 | 1752 | 0 | 1 | 0 | 1 | 54 |
779 | 1 | 2 | 479 | 1 | 1 | 0 | 1 | 204 |
780 | 1 | 3 | 2564 | 0 | 1 | 0 | 1 | 73 |
781 | 1 | 1 | 2058 | 0 | 1 | 1 | 0 | 0 |
782 | 1 | 0 | 1274 | 0 | 1 | 0 | 0 | 0 |
783 | 1 | 2 | 1710 | 0 | 0 | 1 | 1 | 167 |
784 | 0 | 2 | 1463 | 0 | 1 | 1 | 0 | 0 |
785 | 0 | 0 | 3694 | 0 | 0 | 0 | 0 | 0 |
786 | 1 | 1 | 2828 | 0 | 1 | 0 | 1 | 130 |
787 | 1 | 2 | 1043 | 0 | 1 | 0 | 1 | 335 |
788 | 1 | 2 | 2080 | 1 | 1 | 0 | 1 | 130 |
789 | 0 | 0 | 3747 | 1 | 0 | 0 | 0 | 0 |
790 | 1 | 0 | 3746 | 1 | 0 | 0 | 0 | 0 |
791 | 1 | 0 | 744 | 0 | 1 | 0 | 0 | 0 |
792 | 1 | 2 | 2656 | 0 | 1 | 0 | 1 | 85 |
793 | 1 | 1 | 1154 | 1 | 1 | 1 | 1 | 44 |
794 | 1 | 1 | 2815 | 0 | 0 | 0 | 0 | 0 |
795 | 1 | 2 | 1758 | 0 | 0 | 1 | 0 | 0 |
796 | 1 | 0 | 3159 | 0 | 0 | 0 | 0 | 0 |
797 | 1 | 0 | 3423 | 0 | 1 | 0 | 0 | 0 |
798 | 0 | 1 | 1319 | 1 | 1 | 0 | 0 | 0 |
799 | 1 | 3 | 3374 | 0 | 1 | 1 | 1 | 163 |
800 | 1 | 2 | 744 | 0 | 1 | 1 | 0 | 0 |
801 | 1 | 2 | 1710 | 1 | 1 | 1 | 0 | 0 |
802 | 1 | 2 | 539 | 1 | 1 | 0 | 1 | 131 |
803 | 1 | 1 | 2333 | 0 | 1 | 0 | 1 | 161 |
804 | 1 | 1 | 2626 | 1 | 1 | 0 | 1 | 25 |
805 | 1 | 1 | 2375 | 1 | 1 | 0 | 1 | 145 |
806 | 1 | 0 | 2779 | 1 | 1 | 0 | 0 | 0 |
807 | 1 | 2 | 2887 | 0 | 1 | 1 | 1 | 159 |
808 | 1 | 2 | 834 | 1 | 1 | 1 | 0 | 0 |
809 | 1 | 1 | 2796 | 0 | 0 | 0 | 0 | 0 |
810 | 0 | 1 | 1464 | 0 | 1 | 0 | 0 | 0 |
811 | 0 | 0 | 3369 | 1 | 0 | 0 | 0 | 0 |
812 | 0 | 1 | 2590 | 1 | 1 | 0 | 1 | 163 |
813 | 1 | 1 | 905 | 0 | 1 | 0 | 1 | 81 |
814 | 0 | 2 | 1379 | 1 | 0 | 1 | 0 | 0 |
815 | 1 | 0 | 3394 | 0 | 0 | 0 | 0 | 0 |
816 | 0 | 2 | 2571 | 0 | 0 | 0 | 1 | 174 |
817 | 1 | 2 | 841 | 0 | 0 | 1 | 0 | 0 |
818 | 1 | 0 | 3401 | 1 | 0 | 0 | 0 | 0 |
819 | 1 | 1 | 3502 | 0 | 1 | 0 | 0 | 0 |
820 | 1 | 1 | 2417 | 1 | 1 | 0 | 1 | 270 |
821 | 1 | 1 | 3158 | 0 | 1 | 0 | 1 | 34 |
822 | 1 | 1 | 2387 | 0 | 1 | 1 | 0 | 0 |
823 | 0 | 1 | 155 | 0 | 1 | 0 | 1 | 164 |
824 | 1 | 0 | 3507 | 0 | 1 | 0 | 0 | 0 |
825 | 1 | 0 | 3746 | 0 | 1 | 0 | 0 | 0 |
826 | 1 | 2 | 807 | 1 | 0 | 0 | 1 | 114 |
827 | 1 | 0 | 1890 | 0 | 0 | 0 | 0 | 0 |
828 | 1 | 1 | 3251 | 1 | 1 | 0 | 1 | 15 |
829 | 1 | 1 | 3269 | 0 | 1 | 0 | 1 | 31 |
830 | 1 | 4 | 218 | 1 | 0 | 0 | 1 | 581 |
831 | 1 | 1 | 930 | 0 | 1 | 1 | 0 | 0 |
832 | 1 | 1 | 890 | 1 | 1 | 0 | 0 | 0 |
833 | 1 | 2 | 1710 | 1 | 1 | 1 | 0 | 0 |
834 | 1 | 1 | 2786 | 0 | 1 | 0 | 0 | 0 |
835 | 0 | 11 | 918 | 0 | 1 | 0 | 1 | 1441 |
836 | 0 | 1 | 920 | 1 | 1 | 0 | 0 | 0 |
837 | 1 | 4 | 1240 | 0 | 1 | 0 | 1 | 232 |
838 | 1 | 0 | 3694 | 0 | 1 | 0 | 0 | 0 |
839 | 1 | 2 | 4005 | 0 | 1 | 0 | 1 | 141 |
840 | 1 | 2 | 1618 | 1 | 0 | 1 | 1 | 40 |
841 | 1 | 2 | 1882 | 0 | 0 | 1 | 0 | 0 |
842 | 1 | 1 | 1646 | 0 | 1 | 1 | 0 | 0 |
843 | 1 | 3 | 3573 | 0 | 0 | 0 | 1 | 440 |
844 | 1 | 2 | 1962 | 0 | 0 | 1 | 0 | 0 |
845 | 0 | 1 | 1589 | 1 | 0 | 0 | 0 | 0 |
846 | 1 | 1 | 1277 | 1 | 0 | 0 | 1 | 164 |
847 | 1 | 0 | 3852 | 0 | 1 | 0 | 0 | 0 |
848 | 1 | 1 | 3024 | 0 | 0 | 0 | 0 | 0 |
849 | 1 | 0 | 3107 | 0 | 0 | 0 | 0 | 0 |
850 | 0 | 1 | 3944 | 1 | 1 | 0 | 1 | 49 |
851 | 1 | 4 | 801 | 1 | 1 | 0 | 1 | 253 |
852 | 1 | 3 | 1759 | 1 | 1 | 1 | 1 | 130 |
853 | 1 | 1 | 2999 | 1 | 1 | 0 | 1 | 160 |
854 | 0 | 4 | 1794 | 1 | 1 | 0 | 1 | 525 |
855 | 1 | 2 | 2975 | 0 | 0 | 0 | 1 | 160 |
856 | 1 | 4 | 610 | 0 | 0 | 1 | 1 | 317 |
857 | 1 | 1 | 3446 | 0 | 1 | 0 | 0 | 0 |
858 | 1 | 1 | 862 | 0 | 1 | 0 | 0 | 0 |
859 | 1 | 1 | 3397 | 1 | 0 | 0 | 0 | 0 |
860 | 1 | 1 | 869 | 0 | 1 | 0 | 0 | 0 |
861 | 1 | 1 | 1261 | 1 | 0 | 1 | 0 | 0 |
862 | 1 | 1 | 1752 | 0 | 1 | 0 | 1 | 160 |
863 | 1 | 1 | 3822 | 1 | 0 | 0 | 1 | 65 |
864 | 1 | 1 | 3047 | 1 | 1 | 1 | 0 | 0 |
865 | 1 | 2 | 744 | 0 | 1 | 1 | 0 | 0 |
866 | 1 | 1 | 1827 | 1 | 1 | 0 | 0 | 0 |
867 | 1 | 2 | 3536 | 0 | 1 | 0 | 1 | 128 |
868 | 1 | 3 | 1121 | 1 | 1 | 0 | 1 | 247 |
869 | 1 | 1 | 3852 | 1 | 1 | 0 | 1 | 128 |
870 | 1 | 1 | 608 | 0 | 1 | 0 | 0 | 0 |
871 | 1 | 2 | 2018 | 0 | 1 | 0 | 1 | 161 |
872 | 1 | 0 | 2744 | 0 | 0 | 0 | 0 | 0 |
873 | 1 | 2 | 1240 | 1 | 1 | 0 | 1 | 54 |
874 | 1 | 2 | 834 | 0 | 0 | 1 | 0 | 0 |
875 | 1 | 3 | 2438 | 1 | 0 | 1 | 1 | 227 |
876 | 0 | 1 | 2487 | 0 | 0 | 0 | 0 | 0 |
877 | 1 | 1 | 2117 | 0 | 1 | 0 | 1 | 161 |
878 | 1 | 1 | 2138 | 1 | 0 | 1 | 0 | 0 |
879 | 1 | 3 | 3522 | 0 | 0 | 0 | 0 | 0 |
880 | 1 | 2 | 2009 | 0 | 1 | 0 | 1 | 230 |
881 | 1 | 0 | 3694 | 0 | 1 | 0 | 0 | 0 |
882 | 0 | 2 | 2418 | 0 | 0 | 0 | 1 | 263 |
883 | 1 | 1 | 1095 | 1 | 0 | 1 | 0 | 0 |
884 | 1 | 0 | 3747 | 0 | 1 | 0 | 0 | 0 |
885 | 1 | 0 | 3158 | 0 | 0 | 0 | 0 | 0 |
886 | 1 | 0 | 162 | 0 | 0 | 0 | 0 | 0 |
887 | 1 | 2 | 1780 | 1 | 0 | 1 | 1 | 130 |
888 | 1 | 3 | 2893 | 0 | 1 | 0 | 1 | 160 |
889 | 1 | 0 | 3680 | 1 | 0 | 0 | 0 | 0 |
890 | 1 | 2 | 1646 | 0 | 0 | 1 | 0 | 0 |
891 | 1 | 0 | 2901 | 0 | 0 | 0 | 0 | 0 |
892 | 1 | 1 | 2863 | 0 | 1 | 1 | 0 | 0 |
893 | 1 | 4 | 332 | 1 | 1 | 0 | 1 | 410 |
894 | 1 | 2 | 1465 | 0 | 0 | 0 | 1 | 228 |
895 | 1 | 3 | 822 | 0 | 0 | 0 | 1 | 421 |
896 | 1 | 2 | 216 | 1 | 0 | 0 | 1 | 43 |
897 | 1 | 2 | 834 | 1 | 0 | 1 | 0 | 0 |
898 | 1 | 1 | 2544 | 1 | 0 | 0 | 1 | 130 |
899 | 1 | 1 | 2912 | 1 | 1 | 0 | 1 | 96 |
900 | 0 | 1 | 17 | 0 | 1 | 0 | 0 | 0 |
901 | 1 | 2 | 1752 | 1 | 0 | 1 | 0 | 0 |
902 | 1 | 1 | 2508 | 0 | 0 | 0 | 1 | 9 |
903 | 1 | 1 | 868 | 1 | 1 | 0 | 0 | 0 |
904 | 1 | 0 | 2900 | 1 | 0 | 0 | 0 | 0 |
905 | 1 | 8 | 1030 | 1 | 0 | 0 | 1 | 819 |
906 | 0 | 1 | 3578 | 1 | 0 | 1 | 1 | 130 |
907 | 1 | 1 | 891 | 1 | 1 | 0 | 1 | 163 |
908 | 1 | 0 | 2968 | 1 | 1 | 0 | 0 | 0 |
909 | 1 | 1 | 2962 | 0 | 0 | 0 | 1 | 68 |
910 | 1 | 0 | 2728 | 0 | 0 | 0 | 0 | 0 |
911 | 1 | 0 | 1232 | 0 | 1 | 0 | 0 | 0 |
912 | 1 | 1 | 3171 | 0 | 1 | 0 | 1 | 260 |
913 | 0 | 1 | 1107 | 0 | 0 | 0 | 0 | 0 |
914 | 1 | 1 | 3454 | 0 | 0 | 0 | 0 | 0 |
915 | 1 | 2 | 2527 | 0 | 0 | 0 | 1 | 10 |
916 | 1 | 2 | 3201 | 0 | 0 | 0 | 0 | 0 |
917 | 1 | 1 | 3822 | 0 | 1 | 0 | 1 | 128 |
918 | 1 | 3 | 1794 | 1 | 1 | 0 | 1 | 107 |
919 | 1 | 13 | 281 | 1 | 0 | 0 | 1 | 1443 |
920 | 1 | 0 | 3944 | 1 | 1 | 0 | 0 | 0 |
921 | 1 | 0 | 2742 | 0 | 0 | 0 | 0 | 0 |
922 | 1 | 1 | 3641 | 0 | 1 | 0 | 0 | 0 |
923 | 1 | 3 | 2814 | 0 | 0 | 0 | 1 | 162 |
924 | 1 | 1 | 2107 | 1 | 0 | 0 | 1 | 130 |
925 | 0 | 1 | 3425 | 1 | 1 | 0 | 0 | 0 |
926 | 1 | 1 | 1218 | 1 | 1 | 0 | 1 | 44 |
927 | 1 | 3 | 400 | 1 | 1 | 0 | 1 | 522 |
928 | 1 | 0 | 3746 | 0 | 0 | 0 | 0 | 0 |
929 | 1 | 7 | 2842 | 1 | 0 | 0 | 1 | 1393 |
930 | 1 | 3 | 486 | 0 | 1 | 1 | 1 | 266 |
931 | 1 | 2 | 3445 | 0 | 0 | 0 | 0 | 0 |
932 | 1 | 0 | 3680 | 0 | 0 | 0 | 0 | 0 |
933 | 0 | 1 | 1190 | 0 | 1 | 0 | 1 | 205 |
934 | 1 | 5 | 1011 | 0 | 1 | 1 | 1 | 471 |
935 | 1 | 0 | 55 | 0 | 0 | 0 | 0 | 0 |
936 | 1 | 0 | 3747 | 0 | 0 | 0 | 0 | 0 |
937 | 0 | 1 | 2053 | 0 | 0 | 0 | 1 | 345 |
938 | 0 | 0 | 2765 | 0 | 0 | 0 | 0 | 0 |
939 | 1 | 0 | 3354 | 1 | 1 | 0 | 0 | 0 |
940 | 0 | 0 | 4036 | 0 | 0 | 0 | 0 | 0 |
941 | 1 | 1 | 3356 | 0 | 1 | 0 | 0 | 0 |
942 | 1 | 1 | 3249 | 0 | 1 | 0 | 0 | 0 |
943 | 1 | 0 | 3522 | 1 | 1 | 0 | 0 | 0 |
944 | 1 | 0 | 2742 | 0 | 0 | 0 | 0 | 0 |
945 | 1 | 1 | 3678 | 1 | 1 | 0 | 0 | 0 |
946 | 1 | 1 | 2530 | 0 | 0 | 0 | 1 | 136 |
947 | 1 | 0 | 2900 | 0 | 1 | 0 | 0 | 0 |
948 | 1 | 1 | 4096 | 0 | 0 | 1 | 1 | 97 |
949 | 1 | 1 | 1638 | 1 | 1 | 0 | 1 | 30 |
950 | 1 | 3 | 2962 | 1 | 0 | 0 | 1 | 162 |
951 | 1 | 0 | 3339 | 0 | 1 | 0 | 0 | 0 |
952 | 1 | 1 | 4096 | 1 | 0 | 0 | 1 | 128 |
953 | 1 | 0 | 3237 | 0 | 0 | 0 | 0 | 0 |
954 | 1 | 1 | 1288 | 1 | 0 | 1 | 1 | 30 |
955 | 1 | 7 | 1502 | 0 | 0 | 1 | 1 | 347 |
956 | 1 | 0 | 3206 | 0 | 1 | 0 | 0 | 0 |
957 | 0 | 1 | 3548 | 1 | 1 | 0 | 0 | 0 |
958 | 1 | 2 | 2072 | 0 | 1 | 0 | 1 | 105 |
959 | 1 | 3 | 91 | 1 | 0 | 0 | 1 | 1370 |
960 | 1 | 1 | 2533 | 1 | 1 | 0 | 1 | 9 |
961 | 1 | 0 | 3680 | 0 | 1 | 0 | 0 | 0 |
962 | 1 | 3 | 2284 | 1 | 0 | 0 | 1 | 271 |
963 | 1 | 0 | 2735 | 0 | 1 | 0 | 0 | 0 |
964 | 1 | 3 | 2799 | 1 | 0 | 0 | 1 | 104 |
965 | 1 | 3 | 744 | 0 | 1 | 1 | 1 | 128 |
966 | 1 | 1 | 3791 | 0 | 1 | 0 | 0 | 0 |
967 | 0 | 1 | 216 | 1 | 0 | 0 | 1 | 8 |
968 | 0 | 1 | 1556 | 1 | 1 | 0 | 1 | 46 |
969 | 1 | 1 | 2404 | 0 | 0 | 0 | 1 | 114 |
970 | 1 | 2 | 2143 | 0 | 1 | 1 | 0 | 0 |
971 | 1 | 1 | 2100 | 0 | 0 | 0 | 0 | 0 |
972 | 1 | 1 | 2382 | 0 | 1 | 0 | 0 | 0 |
973 | 0 | 1 | 659 | 1 | 1 | 0 | 1 | 58 |
974 | 1 | 1 | 2849 | 1 | 1 | 0 | 0 | 0 |
975 | 1 | 4 | 871 | 0 | 1 | 0 | 1 | 496 |
976 | 1 | 1 | 2677 | 0 | 1 | 0 | 0 | 0 |
977 | 0 | 3 | 1137 | 0 | 1 | 0 | 1 | 292 |
978 | 1 | 2 | 1752 | 0 | 0 | 1 | 0 | 0 |
979 | 0 | 1 | 3222 | 0 | 0 | 0 | 1 | 33 |
980 | 1 | 11 | 220 | 0 | 0 | 0 | 1 | 1227 |
981 | 1 | 4 | 701 | 1 | 1 | 0 | 1 | 290 |
982 | 1 | 1 | 2487 | 1 | 1 | 0 | 0 | 0 |
983 | 1 | 2 | 3791 | 0 | 1 | 1 | 1 | 98 |
984 | 0 | 1 | 427 | 0 | 1 | 0 | 1 | 56 |
985 | 1 | 0 | 3394 | 0 | 1 | 0 | 0 | 0 |
986 | 0 | 1 | 919 | 0 | 1 | 0 | 0 | 0 |
987 | 1 | 3 | 715 | 1 | 1 | 1 | 1 | 129 |
988 | 1 | 0 | 3746 | 0 | 1 | 0 | 0 | 0 |
989 | 1 | 3 | 3521 | 1 | 1 | 0 | 1 | 331 |
990 | 1 | 1 | 3278 | 0 | 0 | 0 | 0 | 0 |
991 | 1 | 0 | 3746 | 1 | 1 | 0 | 0 | 0 |
992 | 1 | 1 | 3229 | 1 | 1 | 0 | 1 | 279 |
993 | 0 | 1 | 2648 | 0 | 0 | 0 | 1 | 46 |
994 | 1 | 1 | 868 | 1 | 0 | 0 | 1 | 43 |
995 | 1 | 1 | 3684 | 0 | 1 | 0 | 0 | 0 |
996 | 1 | 4 | 442 | 1 | 0 | 0 | 1 | 350 |
997 | 1 | 0 | 2768 | 0 | 1 | 0 | 0 | 0 |
998 | 1 | 8 | 1130 | 1 | 0 | 1 | 1 | 559 |
999 | 1 | 1 | 2828 | 1 | 1 | 0 | 1 | 100 |
1000 | 1 | 0 | 3522 | 0 | 0 | 0 | 0 | 0 |
1001 | 1 | 0 | 3403 | 1 | 1 | 0 | 0 | 0 |
1002 | 1 | 1 | 3242 | 0 | 0 | 0 | 0 | 0 |
1003 | 1 | 3 | 2200 | 0 | 1 | 1 | 1 | 161 |
1004 | 1 | 1 | 3024 | 0 | 1 | 0 | 0 | 0 |
1005 | 1 | 1 | 1010 | 1 | 0 | 0 | 0 | 0 |
1006 | 1 | 3 | 1242 | 0 | 0 | 1 | 1 | 165 |
1007 | 1 | 2 | 834 | 0 | 0 | 1 | 0 | 0 |
1008 | 0 | 2 | 469 | 0 | 0 | 0 | 1 | 338 |
1009 | 1 | 1 | 2016 | 0 | 1 | 0 | 1 | 54 |
1010 | 0 | 3 | 1379 | 0 | 1 | 0 | 1 | 143 |
1011 | 1 | 2 | 2361 | 1 | 1 | 1 | 0 | 0 |
1012 | 1 | 1 | 427 | 0 | 1 | 0 | 0 | 0 |
1013 | 1 | 0 | 2773 | 1 | 1 | 0 | 0 | 0 |
1014 | 1 | 1 | 791 | 1 | 1 | 0 | 1 | 68 |
1015 | 1 | 1 | 3433 | 0 | 1 | 0 | 0 | 0 |
1016 | 0 | 1 | 3548 | 0 | 0 | 0 | 0 | 0 |
1017 | 1 | 2 | 1801 | 1 | 1 | 1 | 0 | 0 |
1018 | 1 | 2 | 1710 | 0 | 1 | 1 | 1 | 311 |
1019 | 1 | 1 | 198 | 0 | 0 | 0 | 0 | 0 |
1020 | 1 | 3 | 1879 | 0 | 1 | 1 | 1 | 164 |
1021 | 1 | 1 | 2438 | 1 | 1 | 0 | 1 | 104 |
1022 | 1 | 0 | 2024 | 1 | 1 | 0 | 0 | 0 |
1023 | 0 | 1 | 1095 | 0 | 1 | 1 | 0 | 0 |
1024 | 1 | 1 | 1836 | 1 | 1 | 0 | 1 | 130 |
1025 | 1 | 2 | 549 | 0 | 0 | 0 | 1 | 215 |
1026 | 1 | 4 | 2052 | 1 | 0 | 0 | 1 | 355 |
1027 | 1 | 2 | 3706 | 0 | 1 | 0 | 1 | 228 |
1028 | 1 | 1 | 3360 | 1 | 0 | 0 | 1 | 128 |
1029 | 1 | 4 | 2240 | 0 | 1 | 1 | 1 | 189 |
1030 | 1 | 1 | 2802 | 0 | 0 | 0 | 0 | 0 |
1031 | 1 | 1 | 1577 | 0 | 1 | 0 | 0 | 0 |
1032 | 1 | 1 | 1052 | 1 | 1 | 0 | 1 | 40 |
1033 | 1 | 6 | 1122 | 0 | 0 | 1 | 1 | 425 |
1034 | 1 | 0 | 3680 | 1 | 1 | 0 | 0 | 0 |
1035 | 1 | 5 | 534 | 0 | 0 | 0 | 1 | 361 |
1036 | 1 | 1 | 1 | 1 | 1 | 0 | 1 | 109 |
1037 | 1 | 2 | 492 | 0 | 1 | 0 | 1 | 43 |
1038 | 1 | 1 | 2285 | 1 | 0 | 0 | 0 | 0 |
1039 | 0 | 0 | 2781 | 0 | 1 | 0 | 0 | 0 |
1040 | 1 | 2 | 472 | 1 | 1 | 0 | 1 | 229 |
1041 | 1 | 1 | 2768 | 0 | 1 | 0 | 0 | 0 |
1042 | 1 | 2 | 2464 | 1 | 1 | 0 | 1 | 86 |
1043 | 1 | 0 | 3403 | 0 | 1 | 0 | 0 | 0 |
1044 | 1 | 1 | 2977 | 1 | 1 | 0 | 1 | 160 |
1045 | 0 | 2 | 469 | 1 | 1 | 0 | 1 | 377 |
1046 | 1 | 2 | 3566 | 1 | 1 | 0 | 1 | 229 |
1047 | 1 | 3 | 2389 | 0 | 1 | 0 | 1 | 385 |
1048 | 0 | 1 | 2249 | 1 | 1 | 0 | 1 | 146 |
1049 | 1 | 1 | 4096 | 1 | 1 | 0 | 1 | 229 |
1050 | 1 | 0 | 2977 | 0 | 1 | 0 | 0 | 0 |
1051 | 0 | 1 | 2794 | 1 | 0 | 0 | 0 | 0 |
1052 | 1 | 2 | 1618 | 0 | 0 | 1 | 0 | 0 |
1053 | 1 | 1 | 3067 | 0 | 0 | 0 | 1 | 229 |
1054 | 1 | 1 | 3822 | 1 | 0 | 0 | 0 | 0 |
1055 | 1 | 1 | 513 | 1 | 1 | 0 | 1 | 119 |
1056 | 1 | 2 | 442 | 0 | 0 | 0 | 1 | 138 |
1057 | 1 | 6 | 471 | 0 | 1 | 1 | 1 | 623 |
1058 | 1 | 2 | 1962 | 1 | 0 | 1 | 0 | 0 |
1059 | 0 | 1 | 1800 | 0 | 0 | 1 | 0 | 0 |
1060 | 0 | 5 | 1340 | 1 | 0 | 0 | 1 | 262 |
1061 | 1 | 0 | 1451 | 0 | 0 | 0 | 0 | 0 |
1062 | 0 | 1 | 912 | 0 | 0 | 0 | 1 | 551 |
1063 | 1 | 1 | 2752 | 0 | 1 | 1 | 1 | 35 |
1064 | 1 | 2 | 1379 | 1 | 0 | 1 | 1 | 63 |
1065 | 1 | 2 | 1415 | 0 | 0 | 1 | 0 | 0 |
1066 | 1 | 6 | 380 | 1 | 1 | 0 | 1 | 450 |
1067 | 1 | 2 | 3791 | 0 | 0 | 0 | 0 | 0 |
1068 | 1 | 1 | 1261 | 1 | 0 | 1 | 1 | 44 |
1069 | 1 | 2 | 1379 | 0 | 0 | 1 | 1 | 193 |
1070 | 1 | 1 | 2072 | 0 | 1 | 0 | 0 | 0 |
1071 | 1 | 0 | 3500 | 0 | 1 | 0 | 0 | 0 |
1072 | 1 | 12 | 220 | 0 | 1 | 0 | 1 | 1446 |
1073 | 1 | 1 | 3339 | 1 | 0 | 0 | 1 | 38 |
1074 | 0 | 1 | 604 | 1 | 0 | 0 | 0 | 0 |
1075 | 0 | 2 | 1521 | 1 | 0 | 1 | 0 | 0 |
1076 | 1 | 2 | 3444 | 0 | 1 | 0 | 1 | 71 |
1077 | 0 | 2 | 1582 | 0 | 1 | 0 | 0 | 0 |
1078 | 1 | 0 | 2905 | 0 | 0 | 0 | 0 | 0 |
1079 | 1 | 1 | 967 | 1 | 0 | 0 | 0 | 0 |
1080 | 1 | 2 | 2571 | 1 | 1 | 0 | 1 | 161 |
1081 | 1 | 1 | 2857 | 0 | 0 | 0 | 0 | 0 |
1082 | 1 | 2 | 1882 | 0 | 0 | 1 | 0 | 0 |
1083 | 0 | 0 | 3502 | 0 | 1 | 0 | 0 | 0 |
1084 | 1 | 2 | 2257 | 1 | 0 | 0 | 1 | 264 |
1085 | 0 | 1 | 2961 | 0 | 1 | 1 | 0 | 0 |
1086 | 1 | 2 | 1882 | 1 | 0 | 1 | 1 | 4 |
1087 | 1 | 2 | 3608 | 1 | 0 | 0 | 1 | 22 |
1088 | 0 | 3 | 486 | 0 | 1 | 0 | 1 | 321 |
1089 | 1 | 2 | 490 | 0 | 0 | 0 | 1 | 204 |
1090 | 0 | 1 | 3040 | 1 | 1 | 0 | 0 | 0 |
1091 | 0 | 1 | 1318 | 1 | 1 | 0 | 0 | 0 |
1092 | 0 | 2 | 1920 | 1 | 1 | 0 | 1 | 138 |
1093 | 1 | 2 | 1882 | 0 | 1 | 1 | 0 | 0 |
1094 | 1 | 0 | 3694 | 1 | 1 | 0 | 0 | 0 |
1095 | 0 | 1 | 2978 | 1 | 0 | 0 | 0 | 0 |
1096 | 1 | 2 | 1882 | 0 | 1 | 1 | 0 | 0 |
1097 | 1 | 1 | 1010 | 1 | 0 | 0 | 0 | 0 |
1098 | 0 | 7 | 700 | 1 | 0 | 1 | 1 | 438 |
1099 | 1 | 6 | 239 | 1 | 0 | 0 | 1 | 1161 |
1100 | 1 | 1 | 486 | 1 | 1 | 0 | 1 | 159 |
1101 | 1 | 2 | 1882 | 1 | 1 | 1 | 0 | 0 |
1102 | 1 | 1 | 3009 | 1 | 1 | 0 | 1 | 184 |
1103 | 1 | 1 | 3311 | 1 | 1 | 0 | 0 | 0 |
1104 | 1 | 2 | 2460 | 1 | 1 | 0 | 1 | 162 |
1105 | 1 | 3 | 455 | 0 | 0 | 1 | 0 | 0 |
1106 | 1 | 2 | 1021 | 0 | 1 | 1 | 1 | 285 |
1107 | 1 | 3 | 1109 | 0 | 0 | 1 | 1 | 173 |
1108 | 1 | 1 | 1350 | 1 | 1 | 0 | 0 | 0 |
1109 | 1 | 1 | 2327 | 0 | 0 | 1 | 1 | 158 |
1110 | 1 | 1 | 724 | 1 | 1 | 0 | 1 | 43 |
1111 | 1 | 4 | 1856 | 1 | 0 | 1 | 0 | 0 |
1112 | 1 | 0 | 3694 | 0 | 1 | 0 | 0 | 0 |
1113 | 0 | 1 | 828 | 0 | 0 | 0 | 0 | 0 |
1114 | 1 | 1 | 2506 | 0 | 0 | 0 | 0 | 0 |
1115 | 1 | 2 | 2960 | 0 | 1 | 1 | 1 | 160 |
1116 | 0 | 1 | 372 | 0 | 0 | 0 | 1 | 149 |
1117 | 1 | 2 | 1962 | 0 | 0 | 0 | 0 | 0 |
1118 | 1 | 1 | 556 | 1 | 0 | 0 | 1 | 174 |
1119 | 0 | 0 | 3124 | 0 | 1 | 0 | 0 | 0 |
1120 | 1 | 1 | 2662 | 1 | 1 | 0 | 0 | 0 |
1121 | 0 | 1 | 3548 | 1 | 0 | 0 | 0 | 0 |
1122 | 1 | 1 | 2404 | 0 | 0 | 0 | 0 | 0 |
1123 | 1 | 2 | 1521 | 0 | 1 | 0 | 0 | 0 |
1124 | 1 | 0 | 3747 | 1 | 1 | 0 | 0 | 0 |
1125 | 1 | 1 | 23 | 1 | 1 | 0 | 0 | 0 |
1126 | 1 | 1 | 658 | 0 | 1 | 0 | 1 | 56 |
1127 | 0 | 1 | 1402 | 1 | 0 | 0 | 0 | 0 |
1128 | 1 | 1 | 3400 | 0 | 0 | 0 | 0 | 0 |
1129 | 1 | 1 | 3458 | 0 | 0 | 0 | 0 | 0 |
1130 | 1 | 1 | 1879 | 0 | 0 | 1 | 0 | 0 |
1131 | 1 | 1 | 2856 | 0 | 0 | 0 | 0 | 0 |
1132 | 1 | 1 | 2872 | 0 | 0 | 0 | 0 | 0 |
1133 | 0 | 1 | 829 | 0 | 1 | 0 | 1 | 89 |
1134 | 1 | 1 | 1779 | 0 | 0 | 0 | 0 | 0 |
1135 | 1 | 0 | 1036 | 1 | 1 | 0 | 0 | 0 |
1136 | 1 | 7 | 360 | 1 | 0 | 0 | 1 | 1305 |
1137 | 1 | 1 | 995 | 0 | 1 | 1 | 0 | 0 |
1138 | 1 | 0 | 3694 | 1 | 1 | 0 | 0 | 0 |
1139 | 0 | 3 | 835 | 1 | 0 | 0 | 1 | 241 |
1140 | 1 | 1 | 890 | 1 | 0 | 0 | 0 | 0 |
1141 | 1 | 0 | 3159 | 1 | 0 | 0 | 0 | 0 |
1142 | 1 | 1 | 3292 | 0 | 0 | 0 | 1 | 128 |
1143 | 1 | 1 | 2467 | 1 | 1 | 0 | 1 | 130 |
1144 | 1 | 1 | 1619 | 1 | 0 | 0 | 1 | 20 |
1145 | 0 | 1 | 1079 | 1 | 1 | 0 | 0 | 0 |
1146 | 1 | 1 | 2739 | 0 | 0 | 0 | 1 | 8 |
1147 | 1 | 4 | 1877 | 0 | 0 | 0 | 1 | 255 |
1148 | 1 | 0 | 2877 | 0 | 0 | 0 | 0 | 0 |
1149 | 1 | 1 | 3286 | 1 | 0 | 0 | 1 | 128 |
1150 | 1 | 0 | 2786 | 0 | 1 | 0 | 0 | 0 |
1151 | 1 | 0 | 2900 | 0 | 0 | 0 | 0 | 0 |
1152 | 0 | 0 | 3747 | 1 | 1 | 0 | 0 | 0 |
1153 | 1 | 1 | 3822 | 0 | 1 | 0 | 1 | 97 |
1154 | 1 | 1 | 23 | 1 | 1 | 0 | 0 | 0 |
1155 | 1 | 1 | 27 | 0 | 1 | 0 | 0 | 0 |
1156 | 1 | 1 | 868 | 0 | 1 | 0 | 0 | 0 |
1157 | 1 | 1 | 456 | 1 | 0 | 1 | 1 | 306 |
1158 | 1 | 2 | 3201 | 0 | 1 | 0 | 0 | 0 |
1159 | 1 | 1 | 134 | 0 | 0 | 0 | 1 | 163 |
1160 | 1 | 1 | 4157 | 1 | 1 | 0 | 1 | 254 |
1161 | 1 | 1 | 204 | 0 | 1 | 0 | 0 | 0 |
1162 | 0 | 1 | 1617 | 1 | 1 | 0 | 1 | 170 |
1163 | 1 | 0 | 1583 | 0 | 0 | 0 | 0 | 0 |
1164 | 1 | 1 | 2863 | 0 | 0 | 0 | 0 | 0 |
1165 | 1 | 3 | 499 | 1 | 1 | 0 | 1 | 237 |
1166 | 1 | 1 | 829 | 1 | 1 | 0 | 1 | 202 |
1167 | 1 | 1 | 220 | 0 | 0 | 0 | 0 | 0 |
1168 | 1 | 0 | 3403 | 0 | 1 | 0 | 0 | 0 |
1169 | 1 | 3 | 835 | 0 | 0 | 1 | 1 | 56 |
1170 | 1 | 0 | 3745 | 0 | 0 | 0 | 0 | 0 |
1171 | 0 | 1 | 437 | 0 | 0 | 0 | 1 | 114 |
1172 | 1 | 1 | 2996 | 0 | 1 | 0 | 0 | 0 |
1173 | 1 | 2 | 2809 | 0 | 0 | 0 | 1 | 191 |
1174 | 1 | 0 | 3746 | 0 | 1 | 0 | 0 | 0 |
1175 | 1 | 1 | 4065 | 0 | 1 | 1 | 1 | 99 |
1176 | 1 | 0 | 3619 | 0 | 1 | 0 | 0 | 0 |
1177 | 1 | 2 | 3641 | 1 | 0 | 0 | 0 | 0 |
1178 | 1 | 0 | 2376 | 1 | 0 | 0 | 0 | 0 |
1179 | 0 | 3 | 743 | 1 | 1 | 1 | 1 | 47 |
1180 | 1 | 2 | 804 | 1 | 0 | 0 | 1 | 303 |
1181 | 1 | 0 | 2786 | 0 | 1 | 0 | 0 | 0 |
1182 | 0 | 0 | 2859 | 0 | 1 | 0 | 0 | 0 |
1183 | 1 | 2 | 1710 | 0 | 0 | 1 | 0 | 0 |
1184 | 1 | 2 | 583 | 1 | 1 | 0 | 1 | 149 |
1185 | 0 | 0 | 2767 | 0 | 1 | 0 | 0 | 0 |
1186 | 1 | 0 | 3914 | 0 | 1 | 0 | 0 | 0 |
1187 | 1 | 3 | 471 | 1 | 1 | 0 | 1 | 249 |
1188 | 1 | 5 | 542 | 0 | 0 | 0 | 1 | 578 |
1189 | 1 | 3 | 463 | 0 | 0 | 0 | 1 | 412 |
1190 | 1 | 1 | 925 | 1 | 1 | 0 | 1 | 104 |
1191 | 1 | 1 | 2863 | 1 | 1 | 0 | 0 | 0 |
1192 | 0 | 0 | 3522 | 0 | 1 | 0 | 0 | 0 |
1193 | 1 | 1 | 3587 | 1 | 1 | 0 | 1 | 33 |
1194 | 1 | 1 | 854 | 0 | 1 | 0 | 0 | 0 |
1195 | 1 | 1 | 2963 | 1 | 1 | 0 | 1 | 165 |
1196 | 0 | 0 | 3507 | 1 | 1 | 0 | 0 | 0 |
1197 | 1 | 1 | 4096 | 1 | 0 | 0 | 0 | 0 |
1198 | 0 | 1 | 1010 | 0 | 1 | 0 | 0 | 0 |
1199 | 1 | 0 | 3747 | 1 | 0 | 0 | 0 | 0 |
1200 | 0 | 0 | 2891 | 0 | 1 | 0 | 0 | 0 |
1201 | 1 | 1 | 3125 | 1 | 0 | 0 | 1 | 103 |
1202 | 1 | 2 | 2899 | 1 | 1 | 0 | 1 | 320 |
1203 | 1 | 2 | 744 | 0 | 1 | 1 | 0 | 0 |
1204 | 0 | 1 | 2564 | 0 | 0 | 0 | 0 | 0 |
1205 | 1 | 0 | 3747 | 0 | 1 | 0 | 0 | 0 |
1206 | 0 | 2 | 3474 | 1 | 1 | 0 | 1 | 45 |
1207 | 1 | 1 | 1646 | 0 | 1 | 1 | 0 | 0 |
1208 | 1 | 0 | 3680 | 0 | 0 | 0 | 0 | 0 |
1209 | 1 | 5 | 525 | 0 | 1 | 0 | 1 | 490 |
1210 | 1 | 0 | 3353 | 0 | 0 | 0 | 0 | 0 |
1211 | 0 | 0 | 2765 | 1 | 0 | 0 | 0 | 0 |
1212 | 1 | 0 | 3114 | 0 | 0 | 0 | 0 | 0 |
1213 | 1 | 1 | 2558 | 1 | 1 | 0 | 0 | 0 |
1214 | 1 | 1 | 2634 | 0 | 1 | 0 | 0 | 0 |
1215 | 0 | 4 | 1018 | 1 | 0 | 0 | 1 | 405 |
1216 | 1 | 2 | 869 | 0 | 1 | 0 | 1 | 117 |
1217 | 1 | 1 | 689 | 1 | 0 | 0 | 0 | 0 |
1218 | 1 | 2 | 2856 | 0 | 0 | 0 | 1 | 78 |
1219 | 0 | 0 | 2632 | 0 | 0 | 0 | 0 | 0 |
1220 | 1 | 1 | 2943 | 1 | 1 | 0 | 1 | 183 |
1221 | 1 | 1 | 2851 | 0 | 1 | 0 | 0 | 0 |
1222 | 1 | 1 | 2522 | 0 | 1 | 1 | 0 | 0 |
1223 | 1 | 1 | 3772 | 0 | 1 | 0 | 0 | 0 |
1224 | 1 | 4 | 37 | 0 | 0 | 1 | 1 | 624 |
1225 | 1 | 0 | 3401 | 0 | 0 | 0 | 0 | 0 |
1226 | 1 | 2 | 2318 | 1 | 0 | 0 | 1 | 161 |
1227 | 1 | 2 | 2165 | 1 | 1 | 0 | 1 | 157 |
1228 | 1 | 1 | 2838 | 1 | 0 | 0 | 1 | 35 |
1229 | 1 | 0 | 2730 | 0 | 0 | 0 | 0 | 0 |
1230 | 1 | 0 | 1451 | 1 | 1 | 0 | 0 | 0 |
1231 | 1 | 6 | 408 | 1 | 0 | 0 | 1 | 197 |
1232 | 1 | 1 | 3180 | 0 | 0 | 0 | 0 | 0 |
1233 | 0 | 1 | 532 | 0 | 0 | 0 | 0 | 0 |
1234 | 1 | 2 | 2817 | 1 | 0 | 0 | 1 | 163 |
1235 | 1 | 1 | 3613 | 0 | 1 | 0 | 0 | 0 |
1236 | 1 | 3 | 1877 | 1 | 1 | 0 | 1 | 212 |
1237 | 1 | 1 | 3270 | 0 | 1 | 0 | 1 | 38 |
1238 | 1 | 1 | 3143 | 0 | 1 | 0 | 1 | 229 |
1239 | 0 | 2 | 511 | 0 | 0 | 1 | 1 | 173 |
1240 | 1 | 0 | 2009 | 0 | 1 | 0 | 0 | 0 |
1241 | 1 | 3 | 1017 | 1 | 1 | 0 | 1 | 254 |
1242 | 1 | 1 | 2744 | 1 | 1 | 0 | 1 | 230 |
1243 | 1 | 2 | 1521 | 1 | 0 | 1 | 1 | 40 |
1244 | 1 | 1 | 784 | 1 | 1 | 1 | 0 | 0 |
1245 | 0 | 3 | 1121 | 1 | 1 | 0 | 1 | 288 |
1246 | 1 | 1 | 2787 | 1 | 1 | 0 | 0 | 0 |
1247 | 1 | 1 | 2255 | 0 | 1 | 0 | 1 | 161 |
1248 | 0 | 2 | 1618 | 0 | 1 | 1 | 0 | 0 |
1249 | 1 | 2 | 1436 | 0 | 1 | 1 | 0 | 0 |
1250 | 1 | 2 | 1275 | 1 | 1 | 1 | 1 | 140 |
1251 | 0 | 1 | 3548 | 0 | 0 | 0 | 0 | 0 |
1252 | 0 | 3 | 1241 | 1 | 0 | 1 | 1 | 255 |
1253 | 1 | 1 | 3215 | 0 | 0 | 0 | 1 | 230 |
1254 | 1 | 1 | 1962 | 0 | 0 | 1 | 0 | 0 |
1255 | 1 | 0 | 3403 | 1 | 1 | 0 | 0 | 0 |
1256 | 1 | 2 | 3564 | 1 | 0 | 1 | 1 | 128 |
1257 | 1 | 4 | 463 | 0 | 1 | 0 | 1 | 517 |
1258 | 1 | 0 | 2569 | 1 | 0 | 0 | 0 | 0 |
1259 | 1 | 1 | 1221 | 1 | 1 | 1 | 1 | 164 |
1260 | 1 | 3 | 1430 | 0 | 1 | 1 | 1 | 65 |
1261 | 1 | 1 | 364 | 0 | 1 | 0 | 1 | 149 |
1262 | 1 | 0 | 3694 | 0 | 1 | 0 | 0 | 0 |
1263 | 1 | 4 | 463 | 0 | 0 | 1 | 1 | 406 |
1264 | 1 | 1 | 3304 | 0 | 0 | 0 | 1 | 129 |
1265 | 0 | 0 | 4096 | 0 | 0 | 0 | 0 | 0 |
1266 | 1 | 1 | 2977 | 0 | 0 | 1 | 0 | 0 |
1267 | 1 | 0 | 3852 | 1 | 0 | 1 | 0 | 0 |
1268 | 1 | 0 | 3354 | 0 | 1 | 0 | 0 | 0 |
1269 | 0 | 1 | 2274 | 0 | 0 | 0 | 1 | 46 |
1270 | 1 | 1 | 1221 | 1 | 1 | 1 | 1 | 44 |
1271 | 1 | 1 | 3464 | 1 | 1 | 0 | 0 | 0 |
1272 | 1 | 1 | 3269 | 1 | 0 | 0 | 0 | 0 |
1273 | 1 | 2 | 3426 | 1 | 0 | 0 | 1 | 198 |
1274 | 1 | 2 | 3243 | 1 | 0 | 1 | 1 | 7 |
1275 | 1 | 1 | 3883 | 1 | 0 | 1 | 1 | 128 |
1276 | 1 | 1 | 3353 | 1 | 0 | 0 | 1 | 213 |
1277 | 1 | 1 | 1147 | 1 | 1 | 0 | 1 | 201 |
1278 | 1 | 0 | 3852 | 0 | 0 | 1 | 0 | 0 |
1279 | 1 | 1 | 2527 | 1 | 0 | 0 | 1 | 161 |
1280 | 1 | 2 | 1758 | 0 | 0 | 0 | 1 | 230 |
1281 | 1 | 1 | 216 | 0 | 0 | 1 | 0 | 0 |
1282 | 1 | 1 | 792 | 1 | 1 | 1 | 0 | 0 |
1283 | 1 | 1 | 3717 | 0 | 1 | 0 | 0 | 0 |
1284 | 1 | 1 | 3755 | 0 | 1 | 0 | 0 | 0 |
1285 | 1 | 1 | 4188 | 1 | 0 | 0 | 0 | 0 |
1286 | 1 | 1 | 666 | 0 | 0 | 0 | 1 | 265 |
1287 | 1 | 2 | 3605 | 0 | 0 | 0 | 1 | 246 |
1288 | 1 | 0 | 3536 | 0 | 1 | 0 | 0 | 0 |
1289 | 0 | 2 | 255 | 1 | 1 | 0 | 1 | 318 |
1290 | 0 | 0 | 2915 | 0 | 1 | 1 | 0 | 0 |
1291 | 1 | 0 | 3401 | 0 | 0 | 0 | 0 | 0 |
1292 | 1 | 5 | 1857 | 0 | 1 | 0 | 1 | 240 |
1293 | 1 | 1 | 890 | 1 | 1 | 0 | 0 | 0 |
1294 | 1 | 1 | 2807 | 1 | 0 | 0 | 1 | 54 |
1295 | 1 | 0 | 167 | 0 | 0 | 1 | 0 | 0 |
1296 | 1 | 1 | 1897 | 0 | 0 | 0 | 1 | 165 |
1297 | 1 | 1 | 2618 | 0 | 0 | 0 | 0 | 0 |
1298 | 0 | 3 | 1969 | 1 | 0 | 1 | 1 | 171 |
1299 | 1 | 2 | 3709 | 1 | 1 | 0 | 1 | 98 |
1300 | 1 | 1 | 217 | 1 | 1 | 0 | 1 | 40 |
1301 | 1 | 0 | 2773 | 1 | 0 | 0 | 0 | 0 |
1302 | 1 | 0 | 3430 | 0 | 0 | 0 | 0 | 0 |
1303 | 1 | 5 | 295 | 1 | 1 | 1 | 1 | 531 |
1304 | 1 | 0 | 3944 | 0 | 1 | 0 | 0 | 0 |
1305 | 1 | 1 | 2914 | 1 | 1 | 0 | 1 | 85 |
1306 | 1 | 0 | 3680 | 1 | 0 | 0 | 0 | 0 |
1307 | 0 | 1 | 3328 | 0 | 1 | 0 | 0 | 0 |
1308 | 1 | 2 | 1521 | 1 | 1 | 1 | 0 | 0 |
1309 | 0 | 2 | 1752 | 0 | 0 | 1 | 0 | 0 |
1310 | 1 | 0 | 3746 | 1 | 0 | 0 | 0 | 0 |
1311 | 1 | 4 | 2850 | 1 | 1 | 1 | 1 | 129 |
1312 | 1 | 2 | 1752 | 1 | 1 | 1 | 0 | 0 |
1313 | 1 | 5 | 72 | 1 | 1 | 0 | 1 | 229 |
1314 | 1 | 2 | 744 | 0 | 1 | 1 | 0 | 0 |
1315 | 1 | 1 | 2128 | 0 | 0 | 0 | 0 | 0 |
1316 | 1 | 9 | 1128 | 1 | 1 | 0 | 1 | 1289 |
1317 | 1 | 0 | 3680 | 0 | 1 | 0 | 0 | 0 |
1318 | 1 | 0 | 3104 | 0 | 1 | 1 | 0 | 0 |
1319 | 1 | 1 | 2310 | 0 | 1 | 0 | 1 | 100 |
1320 | 1 | 1 | 897 | 1 | 0 | 0 | 0 | 0 |
1321 | 1 | 3 | 2235 | 1 | 0 | 0 | 1 | 390 |
1322 | 1 | 1 | 1878 | 1 | 1 | 0 | 1 | 35 |
1323 | 1 | 1 | 3020 | 0 | 1 | 0 | 1 | 129 |
1324 | 1 | 2 | 1592 | 1 | 0 | 1 | 1 | 40 |
1325 | 1 | 4 | 14 | 1 | 0 | 1 | 1 | 303 |
1326 | 1 | 0 | 3747 | 0 | 1 | 0 | 0 | 0 |
1327 | 1 | 0 | 462 | 0 | 1 | 0 | 0 | 0 |
1328 | 1 | 1 | 2696 | 0 | 0 | 0 | 1 | 230 |
1329 | 1 | 1 | 3066 | 0 | 1 | 1 | 0 | 0 |
1330 | 0 | 1 | 2101 | 0 | 1 | 0 | 1 | 146 |
1331 | 1 | 6 | 1581 | 0 | 1 | 0 | 1 | 158 |
1332 | 1 | 3 | 1168 | 0 | 1 | 1 | 1 | 40 |
1333 | 1 | 2 | 1882 | 0 | 1 | 1 | 0 | 0 |
1334 | 1 | 1 | 2383 | 0 | 0 | 0 | 1 | 161 |
1335 | 0 | 2 | 2548 | 1 | 1 | 0 | 1 | 236 |
1336 | 1 | 1 | 2912 | 0 | 1 | 0 | 1 | 161 |
1337 | 1 | 3 | 1021 | 1 | 0 | 0 | 1 | 182 |
1338 | 1 | 0 | 4157 | 1 | 0 | 0 | 0 | 0 |
1339 | 0 | 2 | 1701 | 0 | 1 | 0 | 1 | 192 |
1340 | 0 | 0 | 3401 | 1 | 0 | 0 | 0 | 0 |
1341 | 1 | 10 | 289 | 0 | 1 | 0 | 1 | 1214 |
1342 | 0 | 0 | 3401 | 0 | 0 | 0 | 0 | 0 |
1343 | 1 | 2 | 3374 | 1 | 0 | 0 | 1 | 130 |
1344 | 1 | 1 | 1491 | 0 | 1 | 0 | 0 | 0 |
1345 | 1 | 1 | 2719 | 1 | 0 | 0 | 1 | 105 |
1346 | 0 | 1 | 1228 | 0 | 1 | 0 | 0 | 0 |
1347 | 1 | 0 | 3694 | 1 | 1 | 0 | 0 | 0 |
1348 | 1 | 1 | 647 | 1 | 0 | 0 | 0 | 0 |
1349 | 1 | 1 | 2982 | 0 | 1 | 0 | 0 | 0 |
1350 | 1 | 3 | 995 | 1 | 1 | 0 | 1 | 236 |
1351 | 1 | 1 | 178 | 0 | 1 | 0 | 0 | 0 |
1352 | 1 | 2 | 1780 | 0 | 1 | 1 | 0 | 0 |
1353 | 1 | 1 | 653 | 0 | 0 | 0 | 1 | 263 |
1354 | 1 | 3 | 1995 | 0 | 1 | 1 | 1 | 220 |
1355 | 1 | 0 | 3394 | 1 | 0 | 0 | 0 | 0 |
1356 | 1 | 0 | 3680 | 0 | 1 | 0 | 0 | 0 |
1357 | 0 | 1 | 2268 | 1 | 1 | 0 | 0 | 0 |
1358 | 1 | 0 | 2725 | 1 | 1 | 0 | 0 | 0 |
1359 | 1 | 1 | 2400 | 0 | 1 | 0 | 1 | 161 |
1360 | 1 | 1 | 1512 | 1 | 0 | 0 | 0 | 0 |
1361 | 1 | 3 | 3620 | 1 | 0 | 0 | 1 | 72 |
1362 | 1 | 2 | 429 | 0 | 1 | 0 | 1 | 303 |
1363 | 1 | 1 | 2318 | 0 | 0 | 0 | 1 | 85 |
1364 | 0 | 0 | 3489 | 0 | 1 | 0 | 0 | 0 |
1365 | 0 | 1 | 1058 | 1 | 0 | 1 | 0 | 0 |
1366 | 0 | 1 | 28 | 0 | 0 | 0 | 1 | 255 |
1367 | 1 | 0 | 2173 | 0 | 0 | 0 | 0 | 0 |
1368 | 1 | 3 | 1879 | 1 | 1 | 1 | 1 | 258 |
1369 | 0 | 0 | 2982 | 0 | 1 | 0 | 0 | 0 |
1370 | 1 | 2 | 2891 | 1 | 1 | 0 | 1 | 153 |
1371 | 1 | 2 | 1920 | 1 | 1 | 1 | 1 | 130 |
1372 | 1 | 2 | 1646 | 0 | 1 | 1 | 0 | 0 |
1373 | 0 | 3 | 822 | 0 | 0 | 0 | 1 | 429 |
1374 | 0 | 2 | 1962 | 0 | 0 | 1 | 0 | 0 |
1375 | 1 | 1 | 1100 | 1 | 0 | 0 | 1 | 164 |
1376 | 0 | 2 | 2863 | 0 | 1 | 0 | 0 | 0 |
1377 | 0 | 3 | 2333 | 0 | 1 | 0 | 1 | 175 |
1378 | 1 | 1 | 3660 | 1 | 1 | 0 | 0 | 0 |
1379 | 1 | 0 | 3159 | 0 | 0 | 0 | 0 | 0 |
1380 | 1 | 1 | 2962 | 1 | 1 | 0 | 1 | 128 |
1381 | 1 | 0 | 3122 | 0 | 0 | 0 | 0 | 0 |
1382 | 1 | 1 | 608 | 1 | 1 | 0 | 0 | 0 |
1383 | 1 | 0 | 2901 | 0 | 1 | 0 | 0 | 0 |
1384 | 1 | 1 | 3157 | 1 | 1 | 0 | 1 | 220 |
1385 | 1 | 1 | 890 | 0 | 1 | 0 | 0 | 0 |
1386 | 1 | 1 | 2400 | 1 | 1 | 0 | 1 | 13 |
1387 | 1 | 1 | 644 | 0 | 1 | 0 | 0 | 0 |
1388 | 1 | 1 | 2677 | 0 | 0 | 0 | 1 | 161 |
1389 | 1 | 3 | 772 | 0 | 0 | 0 | 1 | 551 |
1390 | 1 | 3 | 2887 | 0 | 1 | 0 | 1 | 162 |
1391 | 1 | 1 | 1261 | 1 | 0 | 1 | 1 | 45 |
1392 | 1 | 2 | 1346 | 0 | 0 | 0 | 0 | 0 |
1393 | 1 | 0 | 2765 | 0 | 1 | 0 | 0 | 0 |
1394 | 1 | 0 | 2900 | 1 | 0 | 0 | 0 | 0 |
1395 | 1 | 0 | 2508 | 0 | 0 | 1 | 0 | 0 |
1396 | 1 | 0 | 2018 | 0 | 0 | 0 | 0 | 0 |
1397 | 1 | 2 | 1801 | 0 | 0 | 1 | 0 | 0 |
1398 | 0 | 1 | 255 | 1 | 0 | 0 | 1 | 244 |
1399 | 1 | 2 | 217 | 1 | 1 | 1 | 1 | 81 |
1400 | 0 | 1 | 658 | 0 | 1 | 1 | 1 | 203 |
1401 | 1 | 1 | 2723 | 0 | 1 | 0 | 0 | 0 |
1402 | 1 | 1 | 2464 | 0 | 0 | 0 | 1 | 35 |
1403 | 1 | 2 | 1842 | 0 | 1 | 1 | 0 | 0 |
1404 | 1 | 1 | 2960 | 0 | 0 | 1 | 1 | 160 |
1405 | 1 | 0 | 3694 | 0 | 0 | 0 | 0 | 0 |
1406 | 1 | 2 | 1498 | 0 | 1 | 1 | 0 | 0 |
1407 | 1 | 0 | 1242 | 0 | 0 | 0 | 0 | 0 |
1408 | 1 | 1 | 3249 | 0 | 0 | 0 | 0 | 0 |
1409 | 0 | 1 | 2880 | 1 | 1 | 0 | 0 | 0 |
1410 | 1 | 0 | 2960 | 0 | 1 | 0 | 0 | 0 |
1411 | 0 | 1 | 2282 | 0 | 0 | 1 | 0 | 0 |
1412 | 1 | 2 | 1346 | 0 | 1 | 1 | 0 | 0 |
1413 | 1 | 1 | 1968 | 1 | 0 | 0 | 0 | 0 |
1414 | 1 | 0 | 3522 | 1 | 0 | 0 | 0 | 0 |
1415 | 1 | 1 | 3125 | 1 | 0 | 0 | 0 | 0 |
1416 | 0 | 1 | 687 | 0 | 1 | 1 | 0 | 0 |
1417 | 0 | 1 | 3458 | 0 | 1 | 0 | 0 | 0 |
1418 | 0 | 0 | 2728 | 0 | 0 | 0 | 0 | 0 |
1419 | 1 | 1 | 889 | 1 | 0 | 0 | 0 | 0 |
1420 | 1 | 3 | 3433 | 1 | 1 | 0 | 1 | 99 |
1421 | 1 | 1 | 192 | 0 | 0 | 0 | 0 | 0 |
1422 | 1 | 0 | 2779 | 1 | 1 | 0 | 0 | 0 |
1423 | 0 | 0 | 3528 | 0 | 0 | 0 | 0 | 0 |
1424 | 1 | 2 | 3390 | 0 | 0 | 1 | 1 | 98 |
1425 | 1 | 0 | 3061 | 0 | 0 | 1 | 0 | 0 |
1426 | 1 | 2 | 2142 | 0 | 1 | 0 | 1 | 68 |
1427 | 1 | 1 | 2873 | 1 | 1 | 1 | 1 | 128 |
1428 | 1 | 0 | 553 | 0 | 0 | 0 | 0 | 0 |
1429 | 1 | 1 | 2848 | 0 | 0 | 0 | 0 | 0 |
1430 | 1 | 2 | 1995 | 1 | 0 | 1 | 1 | 117 |
1431 | 1 | 1 | 3461 | 1 | 1 | 0 | 1 | 198 |
1432 | 1 | 0 | 3536 | 1 | 0 | 0 | 0 | 0 |
1433 | 1 | 5 | 1240 | 0 | 1 | 0 | 1 | 271 |
1434 | 1 | 0 | 3747 | 0 | 1 | 0 | 0 | 0 |
1435 | 1 | 1 | 2324 | 0 | 0 | 0 | 0 | 0 |
1436 | 1 | 0 | 1044 | 0 | 1 | 1 | 0 | 0 |
1437 | 0 | 3 | 1498 | 0 | 1 | 1 | 1 | 162 |
1438 | 1 | 1 | 3465 | 0 | 0 | 0 | 0 | 0 |
1439 | 1 | 0 | 204 | 0 | 1 | 0 | 0 | 0 |
1440 | 1 | 1 | 2955 | 1 | 0 | 1 | 1 | 184 |
1441 | 1 | 0 | 3619 | 0 | 1 | 0 | 0 | 0 |
1442 | 1 | 1 | 3430 | 0 | 1 | 0 | 0 | 0 |
1443 | 0 | 2 | 1865 | 1 | 0 | 1 | 0 | 0 |
1444 | 1 | 1 | 751 | 0 | 1 | 0 | 1 | 43 |
1445 | 1 | 2 | 3391 | 0 | 1 | 0 | 0 | 0 |
1446 | 1 | 1 | 3683 | 0 | 1 | 1 | 0 | 0 |
1447 | 1 | 1 | 2481 | 1 | 1 | 0 | 1 | 130 |
1448 | 1 | 2 | 3791 | 0 | 0 | 0 | 1 | 198 |
1449 | 1 | 1 | 120 | 1 | 1 | 0 | 0 | 0 |
1450 | 0 | 1 | 2599 | 1 | 0 | 0 | 1 | 149 |
1451 | 1 | 2 | 1135 | 1 | 1 | 0 | 1 | 281 |
1452 | 0 | 0 | 3747 | 0 | 0 | 0 | 0 | 0 |
1453 | 0 | 1 | 4036 | 0 | 1 | 1 | 1 | 129 |
1454 | 1 | 0 | 2186 | 0 | 0 | 0 | 0 | 0 |
1455 | 1 | 5 | 520 | 0 | 0 | 0 | 1 | 456 |
1456 | 0 | 2 | 3552 | 1 | 1 | 0 | 1 | 45 |
1457 | 0 | 1 | 1091 | 1 | 0 | 0 | 0 | 0 |
1458 | 1 | 1 | 567 | 1 | 1 | 1 | 1 | 139 |
1459 | 1 | 1 | 167 | 1 | 0 | 0 | 1 | 43 |
1460 | 0 | 0 | 2803 | 0 | 0 | 0 | 0 | 0 |
1461 | 1 | 0 | 3401 | 0 | 1 | 0 | 0 | 0 |
1462 | 1 | 0 | 3745 | 0 | 1 | 0 | 0 | 0 |
1463 | 0 | 1 | 2697 | 1 | 1 | 0 | 0 | 0 |
1464 | 1 | 2 | 619 | 1 | 1 | 1 | 1 | 43 |
1465 | 0 | 1 | 717 | 1 | 1 | 0 | 1 | 40 |
1466 | 1 | 1 | 3311 | 0 | 0 | 0 | 0 | 0 |
1467 | 1 | 1 | 27 | 1 | 0 | 0 | 0 | 0 |
1468 | 1 | 0 | 2837 | 0 | 0 | 0 | 0 | 0 |
1469 | 1 | 2 | 1149 | 1 | 0 | 0 | 1 | 411 |
1470 | 1 | 1 | 2213 | 0 | 0 | 0 | 1 | 65 |
1471 | 0 | 0 | 2879 | 0 | 1 | 0 | 0 | 0 |
1472 | 1 | 2 | 3405 | 1 | 1 | 0 | 1 | 229 |
1473 | 1 | 1 | 2900 | 0 | 1 | 0 | 0 | 0 |
1474 | 1 | 1 | 1647 | 1 | 1 | 0 | 1 | 267 |
1475 | 1 | 2 | 3374 | 1 | 1 | 0 | 1 | 34 |
1476 | 1 | 1 | 2305 | 0 | 0 | 0 | 1 | 161 |
1477 | 1 | 1 | 2856 | 1 | 1 | 0 | 1 | 130 |
1478 | 1 | 1 | 3600 | 0 | 0 | 1 | 1 | 167 |
1479 | 1 | 4 | 519 | 0 | 1 | 0 | 1 | 422 |
1480 | 1 | 2 | 1752 | 0 | 0 | 1 | 0 | 0 |
1481 | 1 | 0 | 3694 | 1 | 1 | 0 | 0 | 0 |
1482 | 0 | 1 | 2961 | 0 | 1 | 0 | 0 | 0 |
1483 | 1 | 1 | 3464 | 0 | 1 | 0 | 0 | 0 |
1484 | 1 | 0 | 616 | 0 | 1 | 0 | 0 | 0 |
1485 | 1 | 1 | 3052 | 1 | 0 | 0 | 1 | 49 |
1486 | 1 | 2 | 2456 | 1 | 0 | 0 | 1 | 266 |
1487 | 1 | 1 | 1968 | 0 | 0 | 0 | 0 | 0 |
1488 | 1 | 2 | 3615 | 0 | 0 | 0 | 1 | 270 |
1489 | 0 | 0 | 3746 | 1 | 1 | 0 | 0 | 0 |
1490 | 1 | 0 | 1224 | 0 | 1 | 0 | 0 | 0 |
1491 | 1 | 1 | 1008 | 1 | 1 | 0 | 0 | 0 |
1492 | 1 | 1 | 3166 | 0 | 1 | 0 | 0 | 0 |
1493 | 0 | 1 | 976 | 1 | 0 | 0 | 1 | 54 |
1494 | 1 | 1 | 469 | 0 | 0 | 1 | 1 | 163 |
1495 | 1 | 0 | 3914 | 0 | 0 | 0 | 0 | 0 |
1496 | 1 | 3 | 3405 | 0 | 0 | 0 | 1 | 131 |
1497 | 1 | 1 | 2710 | 0 | 0 | 1 | 0 | 0 |
1498 | 1 | 0 | 3694 | 0 | 0 | 0 | 0 | 0 |
1499 | 1 | 2 | 757 | 0 | 1 | 0 | 1 | 163 |
1500 | 1 | 6 | 79 | 0 | 0 | 0 | 1 | 415 |
1501 | 1 | 1 | 861 | 1 | 1 | 0 | 1 | 65 |
1502 | 0 | 1 | 3552 | 1 | 1 | 0 | 1 | 100 |
1503 | 1 | 0 | 2542 | 0 | 0 | 0 | 0 | 0 |
1504 | 1 | 1 | 1112 | 1 | 1 | 0 | 0 | 0 |
1505 | 1 | 0 | 2905 | 0 | 0 | 0 | 0 | 0 |
1506 | 1 | 1 | 1836 | 1 | 1 | 0 | 1 | 40 |
1507 | 1 | 1 | 3615 | 1 | 0 | 0 | 1 | 43 |
1508 | 1 | 1 | 2886 | 1 | 0 | 0 | 0 | 0 |
1509 | 0 | 1 | 2872 | 0 | 0 | 0 | 0 | 0 |
1510 | 1 | 2 | 1780 | 0 | 1 | 1 | 0 | 0 |
1511 | 1 | 0 | 3094 | 0 | 0 | 0 | 0 | 0 |
1512 | 1 | 1 | 923 | 1 | 0 | 0 | 0 | 0 |
1513 | 1 | 1 | 1456 | 0 | 1 | 0 | 0 | 0 |
1514 | 1 | 1 | 3306 | 0 | 1 | 0 | 0 | 0 |
1515 | 1 | 1 | 2298 | 1 | 1 | 0 | 0 | 0 |
1516 | 0 | 1 | 3304 | 0 | 0 | 0 | 1 | 140 |
1517 | 1 | 3 | 3387 | 1 | 1 | 0 | 1 | 203 |
1518 | 1 | 0 | 2885 | 0 | 0 | 0 | 0 | 0 |
1519 | 0 | 1 | 2551 | 0 | 1 | 0 | 1 | 282 |
1520 | 1 | 1 | 2681 | 1 | 1 | 0 | 1 | 130 |
1521 | 1 | 1 | 2996 | 1 | 0 | 0 | 1 | 34 |
1522 | 1 | 3 | 1031 | 1 | 0 | 1 | 1 | 214 |
1523 | 1 | 1 | 2530 | 0 | 1 | 0 | 1 | 130 |
1524 | 1 | 1 | 2690 | 0 | 0 | 0 | 0 | 0 |
1525 | 1 | 3 | 2934 | 0 | 0 | 0 | 1 | 160 |
1526 | 1 | 2 | 3768 | 1 | 0 | 0 | 1 | 198 |
1527 | 1 | 1 | 574 | 1 | 1 | 0 | 1 | 495 |
1528 | 0 | 4 | 1521 | 1 | 1 | 1 | 1 | 20 |
1529 | 1 | 5 | 463 | 1 | 1 | 0 | 1 | 402 |
1530 | 1 | 2 | 1882 | 1 | 0 | 1 | 0 | 0 |
1531 | 1 | 3 | 2348 | 1 | 0 | 0 | 1 | 207 |
1532 | 0 | 1 | 115 | 0 | 0 | 0 | 1 | 164 |
1533 | 0 | 2 | 1197 | 1 | 0 | 0 | 1 | 213 |
1534 | 0 | 2 | 987 | 0 | 1 | 1 | 1 | 58 |
1535 | 1 | 1 | 868 | 1 | 1 | 0 | 0 | 0 |
1536 | 1 | 0 | 3694 | 0 | 0 | 0 | 0 | 0 |
1537 | 0 | 1 | 2891 | 1 | 1 | 1 | 1 | 273 |
1538 | 1 | 3 | 3724 | 1 | 0 | 0 | 1 | 38 |
1539 | 1 | 1 | 3024 | 1 | 1 | 0 | 1 | 129 |
1540 | 1 | 1 | 2982 | 0 | 1 | 0 | 0 | 0 |
1541 | 1 | 1 | 2912 | 1 | 1 | 0 | 1 | 161 |
1542 | 0 | 2 | 3642 | 1 | 1 | 0 | 1 | 125 |
1543 | 1 | 2 | 1240 | 1 | 0 | 0 | 1 | 371 |
1544 | 0 | 1 | 973 | 1 | 0 | 1 | 1 | 243 |
1545 | 1 | 3 | 216 | 1 | 1 | 0 | 1 | 360 |
1546 | 1 | 1 | 2583 | 0 | 1 | 0 | 1 | 55 |
1547 | 1 | 0 | 2373 | 0 | 1 | 0 | 0 | 0 |
1548 | 1 | 3 | 463 | 1 | 0 | 1 | 1 | 302 |
1549 | 1 | 3 | 3376 | 0 | 0 | 1 | 1 | 132 |
1550 | 1 | 1 | 2289 | 1 | 0 | 0 | 1 | 230 |
1551 | 1 | 1 | 3464 | 1 | 0 | 0 | 1 | 38 |
1552 | 1 | 2 | 981 | 0 | 1 | 0 | 0 | 0 |
1553 | 1 | 2 | 2831 | 0 | 0 | 0 | 1 | 100 |
1554 | 0 | 1 | 822 | 1 | 1 | 0 | 1 | 56 |
1555 | 0 | 1 | 1232 | 0 | 0 | 0 | 0 | 0 |
1556 | 1 | 1 | 2831 | 1 | 1 | 0 | 1 | 160 |
1557 | 1 | 1 | 701 | 0 | 1 | 0 | 0 | 0 |
1558 | 1 | 1 | 366 | 0 | 0 | 0 | 0 | 0 |
1559 | 1 | 1 | 2578 | 1 | 0 | 0 | 1 | 161 |
1560 | 1 | 3 | 1206 | 0 | 1 | 0 | 1 | 195 |
1561 | 1 | 2 | 835 | 1 | 1 | 0 | 1 | 99 |
1562 | 0 | 2 | 2233 | 0 | 0 | 0 | 1 | 189 |
1563 | 1 | 2 | 3709 | 1 | 1 | 0 | 1 | 198 |
1564 | 1 | 2 | 3704 | 1 | 1 | 0 | 1 | 326 |
1565 | 1 | 1 | 1267 | 1 | 0 | 0 | 0 | 0 |
1566 | 1 | 1 | 3241 | 1 | 0 | 0 | 1 | 48 |
1567 | 1 | 0 | 2728 | 0 | 1 | 0 | 0 | 0 |
1568 | 1 | 1 | 3243 | 0 | 1 | 0 | 1 | 183 |
1569 | 1 | 0 | 2618 | 1 | 0 | 0 | 0 | 0 |
1570 | 1 | 0 | 2900 | 0 | 0 | 0 | 0 | 0 |
1571 | 1 | 1 | 673 | 0 | 1 | 1 | 0 | 0 |
1572 | 1 | 1 | 2278 | 0 | 0 | 0 | 1 | 54 |
1573 | 1 | 0 | 3944 | 0 | 1 | 0 | 0 | 0 |
1574 | 1 | 3 | 1836 | 0 | 0 | 0 | 1 | 179 |
1575 | 1 | 1 | 2331 | 0 | 1 | 0 | 0 | 0 |
1576 | 1 | 1 | 2074 | 0 | 1 | 0 | 1 | 130 |
1577 | 1 | 1 | 3009 | 1 | 0 | 0 | 0 | 0 |
1578 | 0 | 1 | 640 | 1 | 1 | 0 | 1 | 58 |
1579 | 1 | 2 | 1235 | 0 | 0 | 1 | 0 | 0 |
1580 | 0 | 3 | 1646 | 0 | 0 | 1 | 1 | 98 |
1581 | 1 | 0 | 899 | 0 | 1 | 0 | 0 | 0 |
1582 | 0 | 2 | 2803 | 1 | 1 | 0 | 0 | 0 |
1583 | 1 | 2 | 1868 | 1 | 0 | 0 | 1 | 49 |
1584 | 1 | 1 | 2467 | 1 | 0 | 0 | 1 | 136 |
1585 | 0 | 3 | 1722 | 0 | 0 | 1 | 1 | 439 |
1586 | 1 | 0 | 3680 | 0 | 1 | 0 | 0 | 0 |
1587 | 1 | 1 | 3265 | 0 | 1 | 0 | 1 | 98 |
1588 | 1 | 2 | 3684 | 1 | 1 | 0 | 1 | 69 |
1589 | 1 | 1 | 2618 | 0 | 0 | 0 | 1 | 130 |
1590 | 1 | 0 | 4127 | 0 | 1 | 0 | 0 | 0 |
1591 | 1 | 2 | 1226 | 0 | 0 | 1 | 1 | 40 |
1592 | 1 | 2 | 1582 | 0 | 1 | 0 | 0 | 0 |
1593 | 1 | 1 | 1240 | 1 | 1 | 0 | 0 | 0 |
1594 | 1 | 2 | 2375 | 0 | 1 | 1 | 1 | 318 |
1595 | 1 | 5 | 90 | 0 | 0 | 0 | 1 | 399 |
1596 | 1 | 2 | 1436 | 0 | 0 | 1 | 0 | 0 |
1597 | 1 | 1 | 3311 | 0 | 0 | 0 | 1 | 68 |
1598 | 1 | 1 | 2962 | 1 | 1 | 0 | 1 | 181 |
1599 | 1 | 1 | 2310 | 1 | 0 | 0 | 1 | 136 |
1600 | 1 | 4 | 245 | 1 | 0 | 0 | 1 | 655 |
1601 | 0 | 1 | 1322 | 0 | 1 | 0 | 0 | 0 |
1602 | 1 | 4 | 77 | 0 | 0 | 1 | 1 | 407 |
1603 | 1 | 1 | 2192 | 1 | 0 | 0 | 0 | 0 |
1604 | 1 | 1 | 2690 | 1 | 0 | 0 | 1 | 163 |
1605 | 1 | 1 | 2829 | 0 | 0 | 1 | 1 | 185 |
1606 | 1 | 1 | 253 | 0 | 1 | 1 | 0 | 0 |
1607 | 1 | 1 | 3339 | 0 | 0 | 0 | 0 | 0 |
1608 | 1 | 1 | 2499 | 1 | 0 | 0 | 1 | 85 |
1609 | 0 | 0 | 2009 | 1 | 0 | 0 | 0 | 0 |
1610 | 1 | 2 | 1863 | 1 | 0 | 0 | 1 | 136 |
1611 | 1 | 1 | 2005 | 0 | 1 | 0 | 0 | 0 |
1612 | 0 | 1 | 1164 | 0 | 0 | 0 | 0 | 0 |
1613 | 1 | 0 | 3975 | 0 | 1 | 0 | 0 | 0 |
1614 | 1 | 0 | 3185 | 0 | 1 | 0 | 0 | 0 |
1615 | 1 | 3 | 1400 | 1 | 0 | 1 | 1 | 63 |
1616 | 1 | 1 | 3611 | 0 | 0 | 0 | 1 | 173 |
1617 | 1 | 0 | 2901 | 0 | 0 | 0 | 0 | 0 |
1618 | 1 | 1 | 4036 | 1 | 1 | 1 | 1 | 97 |
1619 | 0 | 5 | 1143 | 1 | 0 | 0 | 1 | 1123 |
1620 | 1 | 2 | 1920 | 0 | 1 | 1 | 0 | 0 |
1621 | 1 | 1 | 2807 | 0 | 0 | 1 | 1 | 60 |
1622 | 1 | 0 | 843 | 0 | 1 | 0 | 0 | 0 |
1623 | 1 | 1 | 3125 | 1 | 0 | 0 | 0 | 0 |
1624 | 1 | 1 | 1584 | 0 | 1 | 0 | 1 | 140 |
1625 | 0 | 1 | 1087 | 1 | 1 | 0 | 1 | 263 |
1626 | 1 | 1 | 723 | 0 | 1 | 1 | 0 | 0 |
1627 | 1 | 1 | 197 | 0 | 1 | 0 | 0 | 0 |
1628 | 1 | 4 | 1856 | 0 | 1 | 0 | 1 | 141 |
1629 | 0 | 0 | 3500 | 1 | 0 | 0 | 0 | 0 |
1630 | 0 | 0 | 3401 | 1 | 1 | 0 | 0 | 0 |
1631 | 0 | 1 | 2996 | 0 | 0 | 0 | 1 | 150 |
1632 | 1 | 1 | 4188 | 0 | 0 | 0 | 1 | 127 |
1633 | 1 | 2 | 1582 | 1 | 0 | 1 | 0 | 0 |
1634 | 1 | 2 | 1646 | 0 | 0 | 1 | 1 | 167 |
1635 | 1 | 0 | 2728 | 0 | 0 | 0 | 0 | 0 |
1636 | 1 | 0 | 2009 | 0 | 0 | 0 | 0 | 0 |
1637 | 1 | 2 | 1582 | 0 | 1 | 1 | 0 | 0 |
1638 | 0 | 1 | 3390 | 1 | 0 | 0 | 0 | 0 |
1639 | 0 | 0 | 3528 | 0 | 1 | 0 | 0 | 0 |
1640 | 0 | 3 | 2682 | 0 | 1 | 0 | 1 | 429 |
1641 | 1 | 1 | 1968 | 0 | 0 | 0 | 0 | 0 |
1642 | 1 | 0 | 3852 | 1 | 1 | 0 | 0 | 0 |
1643 | 1 | 1 | 3262 | 0 | 0 | 0 | 0 | 0 |
1644 | 1 | 5 | 2045 | 1 | 1 | 1 | 1 | 187 |
1645 | 1 | 1 | 2150 | 1 | 1 | 1 | 0 | 0 |
1646 | 1 | 0 | 3131 | 0 | 0 | 0 | 0 | 0 |
1647 | 1 | 0 | 2996 | 0 | 1 | 0 | 0 | 0 |
1648 | 0 | 1 | 71 | 1 | 1 | 0 | 1 | 55 |
1649 | 1 | 1 | 164 | 0 | 0 | 0 | 1 | 135 |
1650 | 0 | 1 | 2670 | 0 | 1 | 0 | 0 | 0 |
1651 | 1 | 0 | 2735 | 0 | 0 | 0 | 0 | 0 |
1652 | 0 | 1 | 3325 | 1 | 1 | 0 | 0 | 0 |
1653 | 1 | 0 | 2732 | 0 | 0 | 0 | 0 | 0 |
1654 | 1 | 2 | 1823 | 0 | 1 | 0 | 1 | 75 |
1655 | 1 | 2 | 2080 | 1 | 1 | 1 | 1 | 161 |
1656 | 0 | 1 | 2466 | 0 | 0 | 0 | 1 | 274 |
1657 | 1 | 1 | 160 | 1 | 0 | 0 | 0 | 0 |
1658 | 1 | 0 | 3747 | 0 | 0 | 0 | 0 | 0 |
1659 | 0 | 2 | 1275 | 0 | 0 | 1 | 0 | 0 |
1660 | 0 | 1 | 2878 | 0 | 1 | 0 | 0 | 0 |
1661 | 1 | 3 | 1976 | 1 | 0 | 0 | 1 | 67 |
1662 | 1 | 0 | 3746 | 0 | 0 | 0 | 0 | 0 |
1663 | 1 | 0 | 2900 | 1 | 1 | 0 | 0 | 0 |
1664 | 1 | 2 | 3419 | 1 | 1 | 0 | 0 | 0 |
1665 | 1 | 1 | 2128 | 1 | 0 | 0 | 1 | 80 |
1666 | 1 | 1 | 1128 | 0 | 1 | 0 | 1 | 153 |
1667 | 1 | 0 | 3401 | 0 | 0 | 0 | 0 | 0 |
1668 | 1 | 2 | 1563 | 0 | 0 | 0 | 1 | 463 |
1669 | 1 | 1 | 2415 | 1 | 0 | 0 | 0 | 0 |
1670 | 1 | 0 | 3747 | 0 | 1 | 0 | 0 | 0 |
1671 | 1 | 1 | 2936 | 1 | 0 | 0 | 1 | 66 |
1672 | 1 | 1 | 4005 | 1 | 1 | 0 | 1 | 68 |
1673 | 0 | 1 | 1416 | 0 | 0 | 0 | 0 | 0 |
1674 | 0 | 1 | 1528 | 0 | 1 | 0 | 0 | 0 |
1675 | 1 | 2 | 1382 | 1 | 1 | 0 | 1 | 159 |
1676 | 1 | 2 | 1856 | 0 | 1 | 1 | 0 | 0 |
1677 | 1 | 1 | 260 | 0 | 0 | 0 | 1 | 148 |
1678 | 1 | 1 | 526 | 1 | 0 | 0 | 1 | 280 |
1679 | 1 | 1 | 3360 | 0 | 0 | 1 | 1 | 247 |
1680 | 1 | 0 | 3507 | 1 | 0 | 0 | 0 | 0 |
1681 | 1 | 1 | 2291 | 1 | 0 | 0 | 0 | 0 |
1682 | 1 | 1 | 1240 | 1 | 1 | 0 | 0 | 0 |
1683 | 1 | 0 | 1835 | 0 | 0 | 0 | 0 | 0 |
1684 | 1 | 1 | 2317 | 1 | 1 | 1 | 0 | 0 |
1685 | 1 | 2 | 1879 | 0 | 0 | 1 | 0 | 0 |
1686 | 1 | 1 | 2327 | 1 | 1 | 0 | 1 | 418 |
1687 | 0 | 2 | 1856 | 0 | 0 | 1 | 0 | 0 |
1688 | 1 | 2 | 1115 | 1 | 0 | 0 | 1 | 390 |
1689 | 1 | 1 | 2408 | 0 | 1 | 0 | 1 | 130 |
1690 | 1 | 1 | 1128 | 0 | 0 | 1 | 1 | 44 |
1691 | 1 | 0 | 2766 | 0 | 1 | 0 | 0 | 0 |
1692 | 1 | 2 | 1123 | 1 | 0 | 0 | 1 | 399 |
1693 | 1 | 2 | 1823 | 0 | 0 | 0 | 1 | 230 |
1694 | 1 | 0 | 3353 | 0 | 0 | 0 | 0 | 0 |
1695 | 1 | 2 | 1801 | 0 | 1 | 1 | 0 | 0 |
1696 | 0 | 1 | 617 | 0 | 1 | 0 | 1 | 178 |
1697 | 1 | 1 | 416 | 1 | 1 | 0 | 1 | 40 |
1698 | 1 | 0 | 3403 | 0 | 1 | 0 | 0 | 0 |
1699 | 1 | 0 | 3401 | 0 | 0 | 0 | 0 | 0 |
1700 | 1 | 2 | 434 | 1 | 0 | 0 | 1 | 184 |
1701 | 1 | 1 | 3306 | 0 | 1 | 0 | 1 | 68 |
1702 | 1 | 1 | 3125 | 1 | 1 | 0 | 1 | 134 |
1703 | 1 | 3 | 556 | 0 | 1 | 1 | 0 | 0 |
1704 | 1 | 7 | 1710 | 1 | 0 | 1 | 1 | 213 |
1705 | 0 | 0 | 3852 | 1 | 1 | 0 | 0 | 0 |
1706 | 1 | 1 | 2746 | 1 | 1 | 0 | 1 | 411 |
1707 | 0 | 2 | 3579 | 1 | 1 | 0 | 1 | 107 |
1708 | 1 | 1 | 3397 | 0 | 0 | 0 | 0 | 0 |
1709 | 1 | 2 | 3646 | 0 | 1 | 0 | 1 | 128 |
1710 | 1 | 0 | 3747 | 0 | 1 | 0 | 0 | 0 |
1711 | 0 | 1 | 1850 | 1 | 0 | 0 | 1 | 136 |
1712 | 0 | 1 | 3458 | 1 | 1 | 0 | 0 | 0 |
1713 | 1 | 1 | 3177 | 0 | 1 | 0 | 1 | 10 |
1714 | 1 | 5 | 1577 | 1 | 0 | 1 | 1 | 588 |
1715 | 1 | 0 | 3694 | 1 | 1 | 0 | 0 | 0 |
1716 | 1 | 1 | 2380 | 1 | 0 | 0 | 0 | 0 |
1717 | 1 | 1 | 2787 | 0 | 0 | 0 | 1 | 4 |
1718 | 1 | 0 | 1710 | 0 | 0 | 0 | 0 | 0 |
1719 | 1 | 1 | 709 | 1 | 1 | 1 | 0 | 0 |
1720 | 1 | 1 | 920 | 0 | 1 | 1 | 1 | 141 |
1721 | 1 | 1 | 2417 | 0 | 0 | 0 | 1 | 85 |
1722 | 1 | 2 | 1962 | 0 | 0 | 1 | 0 | 0 |
1723 | 0 | 1 | 1774 | 1 | 0 | 1 | 0 | 0 |
1724 | 1 | 2 | 2284 | 0 | 1 | 1 | 1 | 251 |
1725 | 1 | 1 | 539 | 1 | 0 | 0 | 0 | 0 |
1726 | 1 | 1 | 3583 | 1 | 0 | 0 | 1 | 43 |
1727 | 1 | 9 | 216 | 1 | 0 | 1 | 1 | 378 |
1728 | 1 | 2 | 1856 | 0 | 1 | 1 | 0 | 0 |
1729 | 1 | 0 | 1352 | 0 | 0 | 0 | 0 | 0 |
1730 | 1 | 2 | 962 | 1 | 1 | 0 | 1 | 206 |
1731 | 1 | 0 | 3171 | 0 | 1 | 1 | 0 | 0 |
1732 | 1 | 1 | 198 | 0 | 0 | 0 | 0 | 0 |
1733 | 0 | 0 | 3164 | 0 | 0 | 1 | 0 | 0 |
1734 | 1 | 1 | 2446 | 0 | 0 | 1 | 1 | 100 |
1735 | 1 | 1 | 1877 | 1 | 1 | 0 | 1 | 56 |
1736 | 1 | 0 | 2900 | 0 | 0 | 0 | 0 | 0 |
1737 | 1 | 0 | 2004 | 0 | 0 | 0 | 0 | 0 |
1738 | 1 | 1 | 192 | 1 | 0 | 0 | 0 | 0 |
1739 | 1 | 1 | 532 | 0 | 1 | 1 | 0 | 0 |
1740 | 1 | 1 | 3222 | 1 | 1 | 0 | 1 | 34 |
1741 | 0 | 0 | 2669 | 0 | 1 | 0 | 0 | 0 |
1742 | 1 | 2 | 1618 | 0 | 1 | 1 | 1 | 3 |
1743 | 0 | 0 | 470 | 0 | 1 | 0 | 0 | 0 |
1744 | 1 | 1 | 3243 | 1 | 0 | 0 | 1 | 252 |
1745 | 1 | 0 | 3694 | 0 | 1 | 0 | 0 | 0 |
1746 | 0 | 1 | 3453 | 1 | 0 | 0 | 0 | 0 |
1747 | 0 | 1 | 1036 | 0 | 1 | 0 | 0 | 0 |
1748 | 1 | 15 | 192 | 0 | 0 | 0 | 1 | 1133 |
1749 | 0 | 2 | 1752 | 0 | 0 | 1 | 0 | 0 |
1750 | 1 | 1 | 2311 | 1 | 1 | 0 | 0 | 0 |
1751 | 1 | 1 | 2998 | 0 | 1 | 0 | 1 | 410 |
1752 | 1 | 1 | 1261 | 1 | 1 | 0 | 1 | 44 |
1753 | 1 | 1 | 2634 | 1 | 0 | 0 | 1 | 74 |
1754 | 1 | 1 | 1514 | 0 | 1 | 0 | 0 | 0 |
1755 | 1 | 1 | 3229 | 0 | 0 | 0 | 1 | 136 |
1756 | 1 | 0 | 3746 | 1 | 1 | 0 | 0 | 0 |
1757 | 1 | 1 | 702 | 1 | 1 | 0 | 0 | 0 |
1758 | 1 | 4 | 227 | 1 | 1 | 1 | 1 | 508 |
1759 | 0 | 2 | 3496 | 0 | 0 | 0 | 1 | 3 |
1760 | 0 | 5 | 644 | 1 | 0 | 1 | 1 | 260 |
1761 | 1 | 3 | 1878 | 0 | 1 | 0 | 1 | 182 |
1762 | 1 | 1 | 1968 | 1 | 0 | 0 | 0 | 0 |
1763 | 1 | 7 | 1130 | 1 | 1 | 0 | 1 | 1055 |
1764 | 0 | 1 | 3311 | 0 | 1 | 0 | 0 | 0 |
1765 | 1 | 2 | 360 | 0 | 1 | 0 | 1 | 279 |
1766 | 1 | 8 | 91 | 0 | 0 | 0 | 1 | 1326 |
1767 | 1 | 1 | 2905 | 1 | 1 | 0 | 0 | 0 |
1768 | 1 | 4 | 875 | 0 | 1 | 1 | 1 | 197 |
1769 | 1 | 0 | 896 | 0 | 0 | 0 | 0 | 0 |
1770 | 1 | 1 | 862 | 0 | 0 | 0 | 0 | 0 |
1771 | 1 | 1 | 2243 | 1 | 0 | 0 | 0 | 0 |
1772 | 1 | 0 | 2859 | 0 | 1 | 0 | 0 | 0 |
1773 | 0 | 1 | 2744 | 0 | 1 | 0 | 0 | 0 |
1774 | 1 | 1 | 2787 | 0 | 1 | 0 | 0 | 0 |
1775 | 1 | 1 | 3523 | 1 | 0 | 1 | 1 | 98 |
1776 | 1 | 1 | 3229 | 1 | 1 | 0 | 1 | 68 |
1777 | 1 | 2 | 2971 | 1 | 1 | 0 | 1 | 316 |
1778 | 1 | 0 | 3487 | 0 | 1 | 0 | 0 | 0 |
1779 | 0 | 1 | 2850 | 0 | 1 | 0 | 0 | 0 |
1780 | 0 | 1 | 1018 | 0 | 0 | 0 | 0 | 0 |
1781 | 1 | 1 | 1948 | 1 | 1 | 0 | 1 | 54 |
1782 | 1 | 1 | 3416 | 1 | 1 | 0 | 1 | 10 |
1783 | 0 | 0 | 2844 | 1 | 0 | 0 | 0 | 0 |
1784 | 1 | 1 | 604 | 0 | 1 | 0 | 0 | 0 |
1785 | 0 | 1 | 4127 | 0 | 0 | 0 | 1 | 35 |
1786 | 1 | 2 | 1983 | 1 | 1 | 0 | 1 | 190 |
1787 | 1 | 0 | 3041 | 0 | 1 | 0 | 0 | 0 |
1788 | 1 | 1 | 3249 | 0 | 0 | 0 | 0 | 0 |
1789 | 0 | 1 | 2880 | 0 | 1 | 0 | 0 | 0 |
1790 | 1 | 0 | 3401 | 0 | 1 | 0 | 0 | 0 |
1791 | 1 | 1 | 3177 | 1 | 0 | 1 | 0 | 0 |
1792 | 1 | 2 | 2366 | 1 | 1 | 0 | 1 | 288 |
1793 | 0 | 2 | 982 | 0 | 1 | 1 | 0 | 0 |
1794 | 1 | 2 | 2831 | 0 | 1 | 1 | 1 | 161 |
1795 | 1 | 1 | 192 | 1 | 0 | 0 | 0 | 0 |
1796 | 1 | 2 | 3703 | 0 | 1 | 0 | 0 | 0 |
1797 | 1 | 1 | 723 | 0 | 0 | 1 | 0 | 0 |
1798 | 1 | 0 | 2997 | 1 | 1 | 0 | 0 | 0 |
1799 | 1 | 0 | 2425 | 0 | 0 | 0 | 0 | 0 |
1800 | 1 | 2 | 2326 | 1 | 1 | 0 | 1 | 343 |
1801 | 1 | 1 | 2796 | 1 | 0 | 0 | 0 | 0 |
1802 | 1 | 2 | 470 | 1 | 0 | 1 | 1 | 242 |
1803 | 0 | 1 | 967 | 0 | 0 | 1 | 0 | 0 |
1804 | 0 | 0 | 2950 | 1 | 0 | 0 | 0 | 0 |
1805 | 1 | 3 | 1658 | 1 | 1 | 1 | 1 | 184 |
1806 | 1 | 1 | 2324 | 0 | 0 | 0 | 0 | 0 |
1807 | 1 | 1 | 945 | 0 | 1 | 0 | 0 | 0 |
1808 | 1 | 2 | 721 | 1 | 0 | 0 | 1 | 83 |
1809 | 1 | 2 | 3354 | 1 | 0 | 0 | 1 | 345 |
1810 | 1 | 2 | 2456 | 0 | 1 | 0 | 1 | 220 |
1811 | 1 | 1 | 3069 | 0 | 1 | 1 | 0 | 0 |
1812 | 1 | 1 | 1095 | 1 | 0 | 0 | 0 | 0 |
1813 | 1 | 3 | 2836 | 1 | 1 | 0 | 1 | 506 |
1814 | 0 | 1 | 2955 | 1 | 1 | 1 | 1 | 173 |
1815 | 0 | 1 | 3342 | 0 | 1 | 0 | 1 | 110 |
1816 | 1 | 1 | 974 | 0 | 0 | 0 | 0 | 0 |
1817 | 1 | 0 | 1653 | 0 | 0 | 0 | 0 | 0 |
1818 | 0 | 2 | 3276 | 1 | 0 | 0 | 1 | 140 |
1819 | 0 | 1 | 2975 | 1 | 0 | 0 | 0 | 0 |
1820 | 0 | 0 | 3494 | 0 | 1 | 0 | 0 | 0 |
1821 | 0 | 2 | 1962 | 1 | 0 | 1 | 1 | 138 |
1822 | 1 | 1 | 1346 | 1 | 0 | 1 | 0 | 0 |
1823 | 1 | 1 | 2752 | 0 | 0 | 0 | 0 | 0 |
1824 | 1 | 2 | 2759 | 0 | 1 | 0 | 1 | 136 |
1825 | 1 | 1 | 512 | 0 | 0 | 1 | 0 | 0 |
1826 | 1 | 0 | 3120 | 0 | 0 | 0 | 0 | 0 |
1827 | 1 | 2 | 744 | 0 | 0 | 1 | 1 | 163 |
1828 | 1 | 1 | 1646 | 1 | 0 | 1 | 0 | 0 |
1829 | 1 | 1 | 4005 | 1 | 0 | 0 | 1 | 43 |
1830 | 1 | 1 | 2656 | 1 | 1 | 0 | 1 | 130 |
1831 | 1 | 1 | 2415 | 0 | 0 | 0 | 0 | 0 |
1832 | 1 | 1 | 2158 | 1 | 0 | 0 | 1 | 10 |
1833 | 1 | 1 | 2107 | 1 | 1 | 0 | 1 | 161 |
1834 | 1 | 1 | 304 | 0 | 1 | 0 | 0 | 0 |
1835 | 0 | 1 | 2918 | 0 | 0 | 0 | 0 | 0 |
1836 | 1 | 1 | 960 | 0 | 1 | 0 | 1 | 81 |
1837 | 1 | 2 | 2080 | 0 | 0 | 1 | 0 | 0 |
1838 | 1 | 1 | 3398 | 0 | 1 | 0 | 1 | 10 |
1839 | 1 | 1 | 2257 | 1 | 0 | 0 | 1 | 130 |
1840 | 1 | 1 | 3517 | 1 | 0 | 0 | 0 | 0 |
1841 | 1 | 1 | 2418 | 1 | 1 | 1 | 1 | 161 |
1842 | 1 | 0 | 3522 | 0 | 1 | 0 | 0 | 0 |
1843 | 1 | 1 | 3436 | 1 | 1 | 0 | 0 | 0 |
1844 | 0 | 0 | 2984 | 1 | 0 | 0 | 0 | 0 |
1845 | 1 | 1 | 3590 | 1 | 1 | 0 | 1 | 228 |
1846 | 1 | 1 | 3513 | 1 | 1 | 0 | 0 | 0 |
1847 | 1 | 1 | 608 | 0 | 0 | 0 | 0 | 0 |
1848 | 1 | 2 | 841 | 0 | 1 | 1 | 0 | 0 |
1849 | 1 | 9 | 216 | 0 | 1 | 0 | 1 | 1176 |
1850 | 1 | 0 | 3159 | 0 | 1 | 1 | 0 | 0 |
1851 | 1 | 1 | 868 | 0 | 0 | 0 | 0 | 0 |
1852 | 1 | 1 | 2102 | 0 | 1 | 0 | 1 | 54 |
1853 | 1 | 3 | 3662 | 1 | 0 | 0 | 1 | 128 |
1854 | 1 | 2 | 3662 | 0 | 0 | 0 | 0 | 0 |
1855 | 1 | 2 | 1123 | 0 | 1 | 0 | 1 | 42 |
1856 | 1 | 2 | 3548 | 1 | 1 | 1 | 1 | 131 |
1857 | 0 | 0 | 3694 | 0 | 1 | 0 | 0 | 0 |
1858 | 1 | 2 | 1710 | 0 | 0 | 1 | 0 | 0 |
1859 | 1 | 0 | 2172 | 0 | 1 | 0 | 0 | 0 |
1860 | 1 | 1 | 896 | 0 | 1 | 0 | 0 | 0 |
1861 | 0 | 1 | 1073 | 1 | 0 | 0 | 1 | 105 |
1862 | 1 | 1 | 3587 | 1 | 1 | 0 | 1 | 43 |
1863 | 1 | 0 | 3507 | 1 | 1 | 0 | 0 | 0 |
1864 | 1 | 1 | 2775 | 0 | 1 | 0 | 1 | 33 |
1865 | 1 | 3 | 2143 | 1 | 0 | 1 | 1 | 242 |
1866 | 1 | 0 | 3680 | 0 | 0 | 0 | 0 | 0 |
1867 | 0 | 5 | 1170 | 1 | 0 | 0 | 1 | 598 |
1868 | 1 | 3 | 1842 | 0 | 1 | 1 | 0 | 0 |
1869 | 1 | 2 | 239 | 1 | 1 | 0 | 1 | 1200 |
1870 | 1 | 0 | 2765 | 0 | 0 | 0 | 0 | 0 |
1871 | 1 | 0 | 3747 | 0 | 0 | 0 | 0 | 0 |
1872 | 1 | 2 | 1392 | 1 | 1 | 0 | 1 | 303 |
1873 | 1 | 1 | 532 | 1 | 1 | 1 | 0 | 0 |
1874 | 1 | 0 | 1962 | 1 | 0 | 0 | 0 | 0 |
1875 | 1 | 2 | 3340 | 0 | 0 | 0 | 1 | 199 |
1876 | 1 | 1 | 3019 | 1 | 1 | 0 | 1 | 184 |
1877 | 0 | 1 | 2767 | 1 | 0 | 0 | 0 | 0 |
1878 | 1 | 0 | 3500 | 1 | 1 | 0 | 0 | 0 |
1879 | 0 | 1 | 1052 | 1 | 1 | 1 | 0 | 0 |
1880 | 1 | 0 | 2422 | 1 | 1 | 0 | 0 | 0 |
1881 | 1 | 2 | 1646 | 0 | 0 | 1 | 1 | 130 |
1882 | 1 | 0 | 3522 | 0 | 1 | 0 | 0 | 0 |
1883 | 1 | 3 | 1739 | 0 | 0 | 1 | 1 | 58 |
1884 | 1 | 1 | 15 | 0 | 1 | 0 | 0 | 0 |
1885 | 1 | 2 | 458 | 0 | 0 | 0 | 1 | 142 |
1886 | 1 | 1 | 2128 | 0 | 0 | 0 | 0 | 0 |
1887 | 1 | 1 | 2509 | 0 | 1 | 0 | 0 | 0 |
1888 | 1 | 1 | 3312 | 0 | 0 | 0 | 1 | 132 |
1889 | 0 | 1 | 1052 | 0 | 0 | 0 | 0 | 0 |
1890 | 1 | 0 | 77 | 0 | 0 | 0 | 0 | 0 |
1891 | 0 | 0 | 2492 | 0 | 1 | 1 | 0 | 0 |
1892 | 0 | 1 | 2859 | 0 | 0 | 0 | 0 | 0 |
1893 | 1 | 2 | 1226 | 0 | 0 | 1 | 1 | 104 |
1894 | 1 | 1 | 668 | 1 | 1 | 0 | 1 | 84 |
1895 | 1 | 0 | 1673 | 0 | 1 | 0 | 0 | 0 |
1896 | 1 | 1 | 3047 | 0 | 1 | 1 | 0 | 0 |
1897 | 1 | 1 | 2744 | 0 | 0 | 0 | 0 | 0 |
1898 | 1 | 2 | 3276 | 0 | 0 | 0 | 1 | 128 |
1899 | 0 | 0 | 3507 | 0 | 0 | 0 | 0 | 0 |
1900 | 1 | 3 | 3215 | 0 | 1 | 0 | 1 | 122 |
1901 | 1 | 3 | 834 | 0 | 1 | 1 | 1 | 160 |
1902 | 0 | 4 | 1135 | 0 | 0 | 0 | 1 | 272 |
1903 | 1 | 0 | 2766 | 0 | 0 | 0 | 0 | 0 |
1904 | 1 | 0 | 3747 | 0 | 1 | 0 | 0 | 0 |
1905 | 1 | 0 | 3522 | 0 | 1 | 0 | 0 | 0 |
1906 | 1 | 2 | 1123 | 0 | 0 | 1 | 1 | 217 |
1907 | 1 | 3 | 598 | 0 | 1 | 1 | 1 | 6 |
1908 | 1 | 2 | 2380 | 1 | 1 | 0 | 1 | 88 |
1909 | 1 | 1 | 532 | 0 | 0 | 1 | 0 | 0 |
1910 | 1 | 1 | 4036 | 1 | 0 | 0 | 1 | 30 |
1911 | 1 | 4 | 841 | 0 | 0 | 1 | 1 | 44 |
1912 | 1 | 1 | 1561 | 1 | 1 | 0 | 0 | 0 |
1913 | 0 | 3 | 1261 | 1 | 0 | 0 | 0 | 0 |
1914 | 1 | 1 | 192 | 1 | 1 | 0 | 0 | 0 |
1915 | 1 | 5 | 465 | 1 | 1 | 0 | 1 | 297 |
1916 | 0 | 1 | 1003 | 1 | 1 | 1 | 0 | 0 |
1917 | 0 | 2 | 1752 | 0 | 0 | 1 | 0 | 0 |
1918 | 0 | 0 | 3394 | 0 | 1 | 0 | 0 | 0 |
1919 | 1 | 1 | 2746 | 0 | 1 | 0 | 0 | 0 |
1920 | 0 | 3 | 2296 | 1 | 0 | 0 | 1 | 514 |
1921 | 1 | 1 | 216 | 0 | 1 | 0 | 0 | 0 |
1922 | 1 | 2 | 3660 | 0 | 1 | 0 | 1 | 33 |
1923 | 1 | 2 | 3222 | 1 | 1 | 0 | 1 | 130 |
1924 | 1 | 1 | 2681 | 1 | 1 | 0 | 0 | 0 |
1925 | 1 | 1 | 2404 | 1 | 1 | 0 | 0 | 0 |
1926 | 1 | 1 | 714 | 0 | 1 | 0 | 1 | 163 |
1927 | 0 | 1 | 2583 | 0 | 0 | 0 | 0 | 0 |
1928 | 1 | 2 | 2296 | 0 | 1 | 0 | 1 | 318 |
1929 | 0 | 5 | 1639 | 1 | 0 | 0 | 1 | 563 |
1930 | 1 | 1 | 2960 | 0 | 1 | 1 | 1 | 160 |
1931 | 1 | 0 | 3746 | 0 | 1 | 0 | 0 | 0 |
1932 | 1 | 1 | 359 | 0 | 0 | 0 | 1 | 43 |
1933 | 1 | 0 | 3619 | 1 | 1 | 0 | 0 | 0 |
1934 | 1 | 1 | 2848 | 0 | 1 | 1 | 0 | 0 |
1935 | 0 | 2 | 519 | 0 | 0 | 0 | 1 | 154 |
1936 | 1 | 1 | 2705 | 0 | 1 | 1 | 0 | 0 |
1937 | 1 | 2 | 3716 | 0 | 1 | 0 | 0 | 0 |
1938 | 1 | 1 | 3655 | 1 | 1 | 0 | 0 | 0 |
1939 | 1 | 1 | 216 | 0 | 0 | 1 | 0 | 0 |
1940 | 1 | 1 | 3914 | 1 | 1 | 0 | 1 | 139 |
1941 | 1 | 2 | 1570 | 1 | 1 | 0 | 1 | 203 |
1942 | 1 | 1 | 3384 | 0 | 0 | 0 | 0 | 0 |
1943 | 1 | 0 | 280 | 1 | 1 | 0 | 0 | 0 |
1944 | 1 | 2 | 1710 | 0 | 0 | 1 | 0 | 0 |
1945 | 1 | 0 | 3522 | 1 | 1 | 0 | 0 | 0 |
1946 | 1 | 2 | 1073 | 0 | 1 | 0 | 1 | 57 |
1947 | 1 | 1 | 3032 | 0 | 1 | 0 | 0 | 0 |
1948 | 1 | 0 | 3369 | 0 | 1 | 0 | 0 | 0 |
1949 | 1 | 1 | 1564 | 1 | 1 | 0 | 1 | 91 |
1950 | 1 | 1 | 3243 | 0 | 1 | 0 | 1 | 135 |
1951 | 1 | 2 | 1000 | 0 | 0 | 0 | 1 | 80 |
1952 | 0 | 1 | 2809 | 0 | 0 | 0 | 0 | 0 |
1953 | 1 | 0 | 2186 | 0 | 1 | 0 | 0 | 0 |
1954 | 1 | 2 | 1899 | 1 | 0 | 0 | 1 | 248 |
1955 | 1 | 1 | 1766 | 0 | 1 | 0 | 1 | 142 |
1956 | 1 | 1 | 3604 | 0 | 1 | 0 | 0 | 0 |
1957 | 1 | 1 | 1288 | 0 | 1 | 0 | 1 | 95 |
1958 | 1 | 2 | 491 | 1 | 0 | 1 | 1 | 259 |
1959 | 1 | 2 | 2012 | 0 | 0 | 1 | 1 | 35 |
1960 | 1 | 1 | 2607 | 1 | 1 | 1 | 0 | 0 |
1961 | 1 | 2 | 1288 | 1 | 1 | 0 | 1 | 508 |
1962 | 1 | 1 | 962 | 0 | 1 | 0 | 1 | 263 |
1963 | 1 | 1 | 1464 | 1 | 1 | 0 | 0 | 0 |
1964 | 1 | 1 | 1919 | 1 | 1 | 0 | 1 | 150 |
1965 | 1 | 1 | 3157 | 0 | 0 | 1 | 0 | 0 |
1966 | 0 | 1 | 2165 | 1 | 0 | 0 | 1 | 138 |
1967 | 0 | 1 | 216 | 0 | 1 | 1 | 0 | 0 |
1968 | 1 | 1 | 3453 | 1 | 0 | 0 | 0 | 0 |
1969 | 1 | 3 | 2837 | 0 | 0 | 1 | 1 | 160 |
1970 | 1 | 2 | 1233 | 0 | 1 | 0 | 1 | 81 |
1971 | 1 | 0 | 3159 | 0 | 1 | 0 | 0 | 0 |
1972 | 1 | 2 | 3541 | 1 | 0 | 1 | 1 | 101 |
1973 | 1 | 3 | 939 | 0 | 0 | 0 | 1 | 320 |
1974 | 1 | 2 | 1135 | 1 | 1 | 0 | 1 | 287 |
1975 | 0 | 1 | 1708 | 0 | 0 | 0 | 1 | 98 |
1976 | 1 | 6 | 778 | 0 | 1 | 0 | 1 | 577 |
1977 | 1 | 2 | 3791 | 1 | 0 | 1 | 1 | 98 |
1978 | 1 | 2 | 3678 | 0 | 1 | 0 | 0 | 0 |
1979 | 1 | 1 | 3020 | 1 | 1 | 0 | 0 | 0 |
1980 | 0 | 2 | 1710 | 0 | 0 | 1 | 0 | 0 |
1981 | 1 | 1 | 3340 | 1 | 1 | 0 | 1 | 98 |
1982 | 1 | 2 | 3381 | 1 | 0 | 0 | 0 | 0 |
1983 | 0 | 1 | 2620 | 1 | 0 | 0 | 0 | 0 |
1984 | 1 | 0 | 3772 | 0 | 1 | 0 | 0 | 0 |
1985 | 1 | 2 | 2044 | 0 | 0 | 1 | 0 | 0 |
1986 | 1 | 1 | 659 | 1 | 1 | 0 | 1 | 188 |
1987 | 1 | 4 | 1710 | 0 | 0 | 1 | 1 | 145 |
1988 | 0 | 1 | 695 | 1 | 0 | 0 | 1 | 58 |
1989 | 1 | 1 | 3299 | 1 | 1 | 0 | 1 | 69 |
1990 | 0 | 1 | 3436 | 0 | 1 | 0 | 1 | 1030 |
1991 | 1 | 3 | 1415 | 1 | 1 | 1 | 1 | 130 |
1992 | 1 | 1 | 2807 | 0 | 1 | 0 | 1 | 55 |
1993 | 1 | 2 | 2466 | 0 | 1 | 0 | 1 | 133 |
1994 | 1 | 0 | 3746 | 1 | 0 | 0 | 0 | 0 |
1995 | 1 | 2 | 1136 | 0 | 1 | 0 | 1 | 184 |
1996 | 1 | 1 | 1701 | 1 | 0 | 0 | 1 | 30 |
1997 | 1 | 1 | 2633 | 1 | 1 | 0 | 1 | 10 |
1998 | 1 | 0 | 3394 | 0 | 0 | 0 | 0 | 0 |
1999 | 1 | 1 | 253 | 0 | 1 | 1 | 0 | 0 |
2000 | 1 | 1 | 1261 | 0 | 0 | 0 | 0 | 0 |
NA
Select variables for regression
selected.var <- c("Spending","Address_US","Freq","last_update","Web","Gender",
"Address_RES")
Partition data
set.seed(1) # set seed for reproducing the partition
train.index <- sample(c(1:dim(Tayko.df)[1]), dim(Tayko.df)[1]*0.7)
train.df <- Tayko.df[train.index, selected.var]
valid.df <- Tayko.df[-train.index, selected.var]
Building the model for Spending
Tayko.lm <- lm(Spending ~ ., data = train.df)
options(scipen = 999)
summary(Tayko.lm)
Call:
lm(formula = Spending ~ ., data = train.df)
Residuals:
Min 1Q Median 3Q Max
-419.79 -78.81 -5.26 33.16 1328.95
Coefficients:
Estimate Std. Error t value Pr(>|t|)
(Intercept) 15.282719 13.521836 1.130 0.25858
Address_US -9.557749 9.521419 -1.004 0.31564
Freq 91.015349 2.850089 31.934 < 0.0000000000000002 ***
last_update -0.010032 0.003403 -2.948 0.00325 **
Web 13.261350 7.260800 1.826 0.06800 .
Gender -1.299859 7.153707 -0.182 0.85584
Address_RES -82.835233 8.914574 -9.292 < 0.0000000000000002 ***
---
Signif. codes: 0 ‘***’ 0.001 ‘**’ 0.01 ‘*’ 0.05 ‘.’ 0.1 ‘ ’ 1
Residual standard error: 133.5 on 1393 degrees of freedom
Multiple R-squared: 0.4719, Adjusted R-squared: 0.4697
F-statistic: 207.5 on 6 and 1393 DF, p-value: < 0.00000000000000022
What does it mean?
Call: is the formula of the predictor variable “Spending”
Residuals: are the difference between the actual observed response and the response the values the model predicted, in this case I will plot the residuals to look for symmetry. Another way to think of it is how far the true value is from the predicted value.
plot(Tayko.lm$residuals)
TKLM <- resid(Tayko.lm)
qqnorm(TKLM)
qqline(TKLM)
NA
NA
This plot will suggest that the data is not normally distrubed
Another way to see distrubution is with the Shapiro-Wilk Test
shapiro.test(TKLM)
Shapiro-Wilk normality test
data: TKLM
W = 0.73318, p-value < 0.00000000000000022
In this case it is not normal distrubted as the p-value is not closes to 1.00
Coefficients
the coefficients are two unknown constants that represent the intercept and slope terms in the linear model. In our case how much the consumer is spending. Ultimately, the analyst wants to find an intercept and a slope such that the resulting fitted line is as close as possible ## Coefficient - Estimate The intercept, in our example, is essentially the expected value of “SPending”
Coefficient - Standard Error
The coefficient Standard Error measures the average amount that the coefficient estimates vary from the actual average value of our response variable. We’d ideally want a lower number relative to its coefficients.
Coefficient - t value
The coefficient t-value is a measure of how many standard deviations our coefficient estimate is far away from 0. We want it to be far away from zero as this would indicate we could reject the null hypothesis
Coefficient - Pr(>t)
The Pr(>t) acronym found in the model output relates to the probability of observing any value equal or larger than t. A small p-value indicates that it is unlikely we will observe a relationship between the predictor and response variables due to chance.
Signif. codes:
These help us build a better model. IF we use only the predictors with three stars to build a model we should see a better result
Multiple R-squared, Adjusted R-squared
The R-squared (R2) statistic provides a measure of how well the model is fitting the actual data. It takes the form of a proportion of variance. R2 is a measure of the linear relationship between our predictor variable and our response / target variable. A side note: In multiple regression settings, the R2 will always increase as more variables are included in the model. That’s why the adjusted R2 is the preferred measure as it adjusts for the number of variables considered.
F-Statistic
F-statistic is a good indicator of whether there is a relationship between our predictor and the response variables. The further the F-statistic is from 1 the better it is. However, how much larger the F-statistic needs to be depends on both the number of data points and the number of predictors. Generally, when the number of data points is large, an F-statistic that is only a little bit larger than 1 is already sufficient to reject the null hypothesis (H0 : There is no relationship between the two variables
Note that the model we ran above was just an example to illustrate how a linear model output looks like in R and how we can start to interpret its components. Obviously the model is not optimised. One way we could start to improve is by transforming our response variable
Prediction
library(forecast)
Tayko.lm.pred <- predict(Tayko.lm, valid.df)
accuracy(Tayko.lm.pred, valid.df$Spending)
ME RMSE MAE MPE MAPE
Test set 4.040333 123.228 78.73779 NaN Inf
Varible selection with stepwose regression
null=lm(Spending~1, data=train.df)
forward_model<-step(null, scope=list(lower=null, upper=Tayko.lm), direction="forward")
Start: AIC=14593.15
Spending ~ 1
Df Sum of Sq RSS AIC
+ Freq 1 20491505 26547018 13794
+ last_update 1 3324525 43713998 14492
+ Web 1 553981 46484542 14579
<none> 47038523 14593
+ Gender 1 10046 47028476 14595
+ Address_RES 1 1757 47036765 14595
+ Address_US 1 926 47037597 14595
Step: AIC=13794.28
Spending ~ Freq
Df Sum of Sq RSS AIC
+ Address_RES 1 1471612 25075405 13716
+ Web 1 85416 26461602 13792
+ last_update 1 47115 26499903 13794
<none> 26547018 13794
+ Address_US 1 35793 26511224 13794
+ Gender 1 710 26546307 13796
Step: AIC=13716.44
Spending ~ Freq + Address_RES
Df Sum of Sq RSS AIC
+ last_update 1 157875 24917530 13710
+ Web 1 58515 25016890 13715
<none> 25075405 13716
+ Address_US 1 22117 25053289 13717
+ Gender 1 770 25074636 13718
Step: AIC=13709.6
Spending ~ Freq + Address_RES + last_update
Df Sum of Sq RSS AIC
+ Web 1 59507 24858023 13708
<none> 24917530 13710
+ Address_US 1 18232 24899298 13711
+ Gender 1 728 24916802 13712
Step: AIC=13708.25
Spending ~ Freq + Address_RES + last_update + Web
Df Sum of Sq RSS AIC
<none> 24858023 13708
+ Address_US 1 18160.9 24839862 13709
+ Gender 1 781.8 24857241 13710
stepwise_model<-step(null, scope=list(lower=null, upper=Tayko.lm), direction="both")
Start: AIC=14593.15
Spending ~ 1
Df Sum of Sq RSS AIC
+ Freq 1 20491505 26547018 13794
+ last_update 1 3324525 43713998 14492
+ Web 1 553981 46484542 14579
<none> 47038523 14593
+ Gender 1 10046 47028476 14595
+ Address_RES 1 1757 47036765 14595
+ Address_US 1 926 47037597 14595
Step: AIC=13794.28
Spending ~ Freq
Df Sum of Sq RSS AIC
+ Address_RES 1 1471612 25075405 13716
+ Web 1 85416 26461602 13792
+ last_update 1 47115 26499903 13794
<none> 26547018 13794
+ Address_US 1 35793 26511224 13794
+ Gender 1 710 26546307 13796
- Freq 1 20491505 47038523 14593
Step: AIC=13716.44
Spending ~ Freq + Address_RES
Df Sum of Sq RSS AIC
+ last_update 1 157875 24917530 13710
+ Web 1 58515 25016890 13715
<none> 25075405 13716
+ Address_US 1 22117 25053289 13717
+ Gender 1 770 25074636 13718
- Address_RES 1 1471612 26547018 13794
- Freq 1 21961360 47036765 14595
Step: AIC=13709.6
Spending ~ Freq + Address_RES + last_update
Df Sum of Sq RSS AIC
+ Web 1 59507 24858023 13708
<none> 24917530 13710
+ Address_US 1 18232 24899298 13711
+ Gender 1 728 24916802 13712
- last_update 1 157875 25075405 13716
- Address_RES 1 1582373 26499903 13794
- Freq 1 18588777 43506308 14488
Step: AIC=13708.25
Spending ~ Freq + Address_RES + last_update + Web
Df Sum of Sq RSS AIC
<none> 24858023 13708
+ Address_US 1 18161 24839862 13709
- Web 1 59507 24917530 13710
+ Gender 1 782 24857241 13710
- last_update 1 158867 25016890 13715
- Address_RES 1 1555037 26413060 13791
- Freq 1 18174345 43032368 14474
backward_model<-step(Tayko.lm, direction="backward")
Start: AIC=13711.19
Spending ~ Address_US + Freq + last_update + Web + Gender + Address_RES
Df Sum of Sq RSS AIC
- Gender 1 589 24839862 13709
- Address_US 1 17968 24857241 13710
<none> 24839273 13711
- Web 1 59483 24898757 13712
- last_update 1 154958 24994231 13718
- Address_RES 1 1539631 26378905 13793
- Freq 1 18184451 43023724 14478
Step: AIC=13709.23
Spending ~ Address_US + Freq + last_update + Web + Address_RES
Df Sum of Sq RSS AIC
- Address_US 1 18161 24858023 13708
<none> 24839862 13709
- Web 1 59436 24899298 13711
- last_update 1 154976 24994838 13716
- Address_RES 1 1540033 26379895 13791
- Freq 1 18192258 43032120 14476
Step: AIC=13708.25
Spending ~ Freq + last_update + Web + Address_RES
Df Sum of Sq RSS AIC
<none> 24858023 13708
- Web 1 59507 24917530 13710
- last_update 1 158867 25016890 13715
- Address_RES 1 1555037 26413060 13791
- Freq 1 18174345 43032368 14474
summary(backward_model)
Call:
lm(formula = Spending ~ Freq + last_update + Web + Address_RES,
data = train.df)
Residuals:
Min 1Q Median 3Q Max
-421.55 -77.25 -5.18 31.86 1326.62
Coefficients:
Estimate Std. Error t value Pr(>|t|)
(Intercept) 7.13180 10.70556 0.666 0.50541
Freq 90.92205 2.84699 31.936 < 0.0000000000000002 ***
last_update -0.01015 0.00340 -2.986 0.00288 **
Web 13.26390 7.25827 1.827 0.06785 .
Address_RES -83.10448 8.89611 -9.342 < 0.0000000000000002 ***
---
Signif. codes: 0 ‘***’ 0.001 ‘**’ 0.01 ‘*’ 0.05 ‘.’ 0.1 ‘ ’ 1
Residual standard error: 133.5 on 1395 degrees of freedom
Multiple R-squared: 0.4715, Adjusted R-squared: 0.47
F-statistic: 311.2 on 4 and 1395 DF, p-value: < 0.00000000000000022
summary(forward_model)
Call:
lm(formula = Spending ~ Freq + Address_RES + last_update + Web,
data = train.df)
Residuals:
Min 1Q Median 3Q Max
-421.55 -77.25 -5.18 31.86 1326.62
Coefficients:
Estimate Std. Error t value Pr(>|t|)
(Intercept) 7.13180 10.70556 0.666 0.50541
Freq 90.92205 2.84699 31.936 < 0.0000000000000002 ***
Address_RES -83.10448 8.89611 -9.342 < 0.0000000000000002 ***
last_update -0.01015 0.00340 -2.986 0.00288 **
Web 13.26390 7.25827 1.827 0.06785 .
---
Signif. codes: 0 ‘***’ 0.001 ‘**’ 0.01 ‘*’ 0.05 ‘.’ 0.1 ‘ ’ 1
Residual standard error: 133.5 on 1395 degrees of freedom
Multiple R-squared: 0.4715, Adjusted R-squared: 0.47
F-statistic: 311.2 on 4 and 1395 DF, p-value: < 0.00000000000000022
summary(stepwise_model)
Call:
lm(formula = Spending ~ Freq + Address_RES + last_update + Web,
data = train.df)
Residuals:
Min 1Q Median 3Q Max
-421.55 -77.25 -5.18 31.86 1326.62
Coefficients:
Estimate Std. Error t value Pr(>|t|)
(Intercept) 7.13180 10.70556 0.666 0.50541
Freq 90.92205 2.84699 31.936 < 0.0000000000000002 ***
Address_RES -83.10448 8.89611 -9.342 < 0.0000000000000002 ***
last_update -0.01015 0.00340 -2.986 0.00288 **
Web 13.26390 7.25827 1.827 0.06785 .
---
Signif. codes: 0 ‘***’ 0.001 ‘**’ 0.01 ‘*’ 0.05 ‘.’ 0.1 ‘ ’ 1
Residual standard error: 133.5 on 1395 degrees of freedom
Multiple R-squared: 0.4715, Adjusted R-squared: 0.47
F-statistic: 311.2 on 4 and 1395 DF, p-value: < 0.00000000000000022
Choseing the right variables now
As you can see Freq, last_update, address_RES are the key predictors so lets make a new formula with those.
Better_Model <- lm(formula = Spending ~ Freq + Address_RES + last_update + Web,
data = valid.df)
summary(Better_Model)
Call:
lm(formula = Spending ~ Freq + Address_RES + last_update + Web,
data = valid.df)
Residuals:
Min 1Q Median 3Q Max
-445.94 -74.97 6.52 32.23 999.64
Coefficients:
Estimate Std. Error t value Pr(>|t|)
(Intercept) -21.320770 14.648338 -1.456 0.146
Freq 101.344111 3.624125 27.964 < 0.0000000000000002 ***
Address_RES -85.897719 12.729987 -6.748 0.0000000000357 ***
last_update -0.002733 0.004657 -0.587 0.557
Web 19.650706 10.263894 1.915 0.056 .
---
Signif. codes: 0 ‘***’ 0.001 ‘**’ 0.01 ‘*’ 0.05 ‘.’ 0.1 ‘ ’ 1
Residual standard error: 122.6 on 595 degrees of freedom
Multiple R-squared: 0.6057, Adjusted R-squared: 0.603
F-statistic: 228.5 on 4 and 595 DF, p-value: < 0.00000000000000022
#Conclusion we can now validate that the factor most involved with Spending habits of the customers are Freq and Address. Which is to say that the spending habits of the consumer are american and they have bought before.
Logistic Regression
select variables for logistic regression
Business Problem Will they purchase yes or no?
selected.var <- c("Purchase","Address_US","Freq","last_update","Web","Gender",
"Address_RES")
partition data
set.seed(5) # set seed for reproducing the partition
train.index <- sample(c(1:dim(Tayko.df)[1]), dim(Tayko.df)[1]*0.7)
train.df <- Tayko.df[train.index, selected.var]
valid.df <- Tayko.df[-train.index, selected.var]
logistic regression model using glm()
In statistics, the generalized linear model (GLM) is a flexible generalization of ordinary linear regression that allows for response variables that have error distribution models other than a normal distribution. The glmnet() function has an alpha argument that determines what type of model is fit. If alpha = 0 then a ridge regression model is fit, and if alpha = 1 then a lasso model is fit.
library(caret)
library(glmnet)
logit.reg <- glm(Purchase ~ ., data = train.df, family = "binomial")
options(scipen=999) # remove scientific notation
summary(logit.reg)
Call:
glm(formula = Purchase ~ ., family = "binomial", data = train.df)
Deviance Residuals:
Min 1Q Median 3Q Max
-3.2384 -0.8908 0.0001 0.9792 2.0750
Coefficients:
Estimate Std. Error z value Pr(>|z|)
(Intercept) -2.654978396 0.272584783 -9.740 < 0.0000000000000002 ***
Address_US 0.151198045 0.180471495 0.838 0.402
Freq 2.066303697 0.129641932 15.939 < 0.0000000000000002 ***
last_update -0.000004276 0.000062811 -0.068 0.946
Web 0.923794671 0.137531679 6.717 0.000000000018556 ***
Gender -0.118614602 0.137017893 -0.866 0.387
Address_RES -1.438979263 0.194460099 -7.400 0.000000000000136 ***
---
Signif. codes: 0 ‘***’ 0.001 ‘**’ 0.01 ‘*’ 0.05 ‘.’ 0.1 ‘ ’ 1
(Dispersion parameter for binomial family taken to be 1)
Null deviance: 1940.8 on 1399 degrees of freedom
Residual deviance: 1297.2 on 1393 degrees of freedom
AIC: 1311.2
Number of Fisher Scoring iterations: 6
Prediction
logit.reg.pred <- predict(logit.reg, valid.df, type = "response")
confusionMatrix(as.factor(ifelse(logit.reg.pred > 0.5, "Purchase", "No Purchase")),
as.factor(ifelse(valid.df$Purchase==1,"Purchase","No Purchase")))
Confusion Matrix and Statistics
Reference
Prediction No Purchase Purchase
No Purchase 222 71
Purchase 82 225
Accuracy : 0.745
95% CI : (0.7081, 0.7794)
No Information Rate : 0.5067
P-Value [Acc > NIR] : <0.0000000000000002
Kappa : 0.4902
Mcnemar's Test P-Value : 0.4188
Sensitivity : 0.7303
Specificity : 0.7601
Pos Pred Value : 0.7577
Neg Pred Value : 0.7329
Prevalence : 0.5067
Detection Rate : 0.3700
Detection Prevalence : 0.4883
Balanced Accuracy : 0.7452
'Positive' Class : No Purchase
ctrl <- trainControl(method = "repeatedcv", number = 10, savePredictions = TRUE)
mod_fit <- train(Purchase ~ Address_US+Freq+last_update+Web+Gender+Address_RES,
data=Tayko.df, method="glm", family="binomial",
trControl = ctrl)
You are trying to do regression and your outcome only has two possible values Are you trying to do classification? If so, use a 2 level factor as your outcome column.
summary(mod_fit)
Call:
NULL
Deviance Residuals:
Min 1Q Median 3Q Max
-3.1998 -0.9101 -0.0895 0.8005 2.1205
Coefficients:
Estimate Std. Error z value Pr(>|z|)
(Intercept) -2.69016918 0.23192505 -11.599 <0.0000000000000002 ***
Address_US 0.08887531 0.14967875 0.594 0.553
Freq 2.02725297 0.10620432 19.088 <0.0000000000000002 ***
last_update 0.00002795 0.00005356 0.522 0.602
Web 0.96667886 0.11519835 8.391 <0.0000000000000002 ***
Gender -0.07942773 0.11428352 -0.695 0.487
Address_RES -1.41279307 0.16147795 -8.749 <0.0000000000000002 ***
---
Signif. codes: 0 ‘***’ 0.001 ‘**’ 0.01 ‘*’ 0.05 ‘.’ 0.1 ‘ ’ 1
(Dispersion parameter for binomial family taken to be 1)
Null deviance: 2772.6 on 1999 degrees of freedom
Residual deviance: 1862.1 on 1993 degrees of freedom
AIC: 1876.1
Number of Fisher Scoring iterations: 6
# select variables for regression
selected.var <- c("Spending","Address_US","Freq","last_update","Web","Gender",
"Address_RES")
partition data
set.seed(6) # set seed for reproducing the partition
train.index <- sample(c(1:dim(Tayko.df)[1]), dim(Tayko.df)[1]*0.7)
train.df <- Tayko.df[train.index, selected.var]
valid.df <- Tayko.df[-train.index, selected.var]
#glmnet
Ridge regression with glmnet# The glmnet package provides the functionality for ridge regression via glmnet(). Important things to know:
Rather than accepting a formula and data frame, it requires a vector input and matrix of predictors. You must specify alpha = 0 for ridge regression. Ridge regression involves tuning a hyperparameter, lambda. glmnet() will generate default values for you. Alternatively, it is common practice to define your own with the lambda argument (which we’ll do).
x <- as.matrix(train.df[,c("Address_US","Freq","last_update","Web","Gender",
"Address_RES")])
y <- train.df$Spending
CvRidgeMod <- cv.glmnet(x, y, alpha=0, nlambda=100, lambda.min.ratio=0.0001)
best.lambda <- CvRidgeMod$lambda.min
best.lambda
[1] 13.26638
coef(CvRidgeMod, s = "lambda.min")
7 x 1 sparse Matrix of class "dgCMatrix"
1
(Intercept) 14.43623446
Address_US -2.85562995
Freq 85.24201532
last_update -0.01026718
Web 15.20347802
Gender -2.49455878
Address_RES -68.61384598
x_pred <- as.matrix(valid.df[,c("Address_US","Freq","last_update","Web","Gender",
"Address_RES")])
Ridge.pred <- predict(CvRidgeMod, s = best.lambda, newx = x_pred)
accuracy(as.vector(Ridge.pred), valid.df$Spending)
ME RMSE MAE MPE MAPE
Test set 6.258279 148.1573 85.76817 NaN Inf
plot(CvRidgeMod)
The lowest point in the curve indicates the optimal lambda: the log value of lambda that best minimised the error in cross-validation. We can extract this values as:
opt_lambda <- CvRidgeMod$lambda.min
opt_lambda
[1] 13.26638
Lasso
Least Absolute Shrinkage and Selection Operator
Lasso regression is a parsimonious model that performs L1 regularization. The L1 regularization adds a penalty equivalent to the absolute magnitude of regression coefficients and tries to minimize them. The equation of lasso is similar to ridge regression and looks like as given below. Lasso regression analysis is also used for variable selection as the model imposes coefficients of some variables to shrink towards zero.
The two models, lasso and ridge regression, are almost similar to each other. However, in lasso, the coefficients which are responsible for large variance are converted to zero. On the other hand, coefficients are only shrunk but are never made zero in ridge regression.
# Lasso
CvLassoMod <- cv.glmnet(x, y, alpha=1, nlambda=100, lambda.min.ratio=0.0001)
plot(CvLassoMod)
best.lambda <- CvLassoMod$lambda.min
best.lambda
[1] 0.3778312
coef(CvLassoMod, s = "lambda.min")
7 x 1 sparse Matrix of class "dgCMatrix"
1
(Intercept) 2.017827662
Address_US -2.967548697
Freq 92.273465509
last_update -0.008102858
Web 12.863715457
Gender -1.524717302
Address_RES -76.238518400
Lasso.pred <- predict(CvLassoMod, s = best.lambda, newx = x_pred)
accuracy(as.vector(Lasso.pred), valid.df$Spending)
ME RMSE MAE MPE MAPE
Test set 6.444591 147.2725 86.61665 NaN Inf
# identifying best lamda
best.lambda
[1] 0.3778312
coef(CvLassoMod, s = "lambda.min")
7 x 1 sparse Matrix of class "dgCMatrix"
1
(Intercept) 2.017827662
Address_US -2.967548697
Freq 92.273465509
last_update -0.008102858
Web 12.863715457
Gender -1.524717302
Address_RES -76.238518400